Prediction of pediatric peanut oral food challenge outcomes using machine learning
Journal of Allergy and Clinical Immunology: Global(2024)
摘要
Background
Clinical testing including food-specific skin and serum immunoglobulin E tests provide limited accuracy to predict food allergy. Confirmatory oral food challenges (OFCs) are often required, but associated risks, cost, and logistical difficulties comprise a barrier to proper diagnosis.
Objective
We sought to utilize advanced machine learning (ML) methodologies to integrate clinical variables associated with peanut allergy to create a predictive model for OFCs to improve predictive performance over purely statistical methods.
Methods
ML was applied to the Learning Early about Peanut Allergy (LEAP) study of 463 peanut OFCs and associated clinical variables. Patient-wise cross-validation was used to create ensemble models that were evaluated on holdout test sets. These models were further evaluated using two additional peanut allergy OFC cohorts: the IMPACT study and a local University of Michigan cohort.
Results
In the LEAP dataset, the ensemble models achieved a maximum mean Area-Under-the-Curve (AUC) of 0.997 with sensitivity and specificity of 0.994 and 1.00 respectively. In the combined validation datasets, the top ensemble model achieved a maximum AUC of 0.871 with sensitivity and specificity of 0.763 and 0.980 respectively.
Conclusions
Machine learning models for predicting peanut OFCs have the potential to accurately predict OFC outcomes, potentially minimizing the need for OFCs while increasing confidence in food allergy diagnoses.
更多查看译文
关键词
Peanut allergy,food allergy,anaphylaxis,oral food challenges,machine learning
AI 理解论文
溯源树
样例
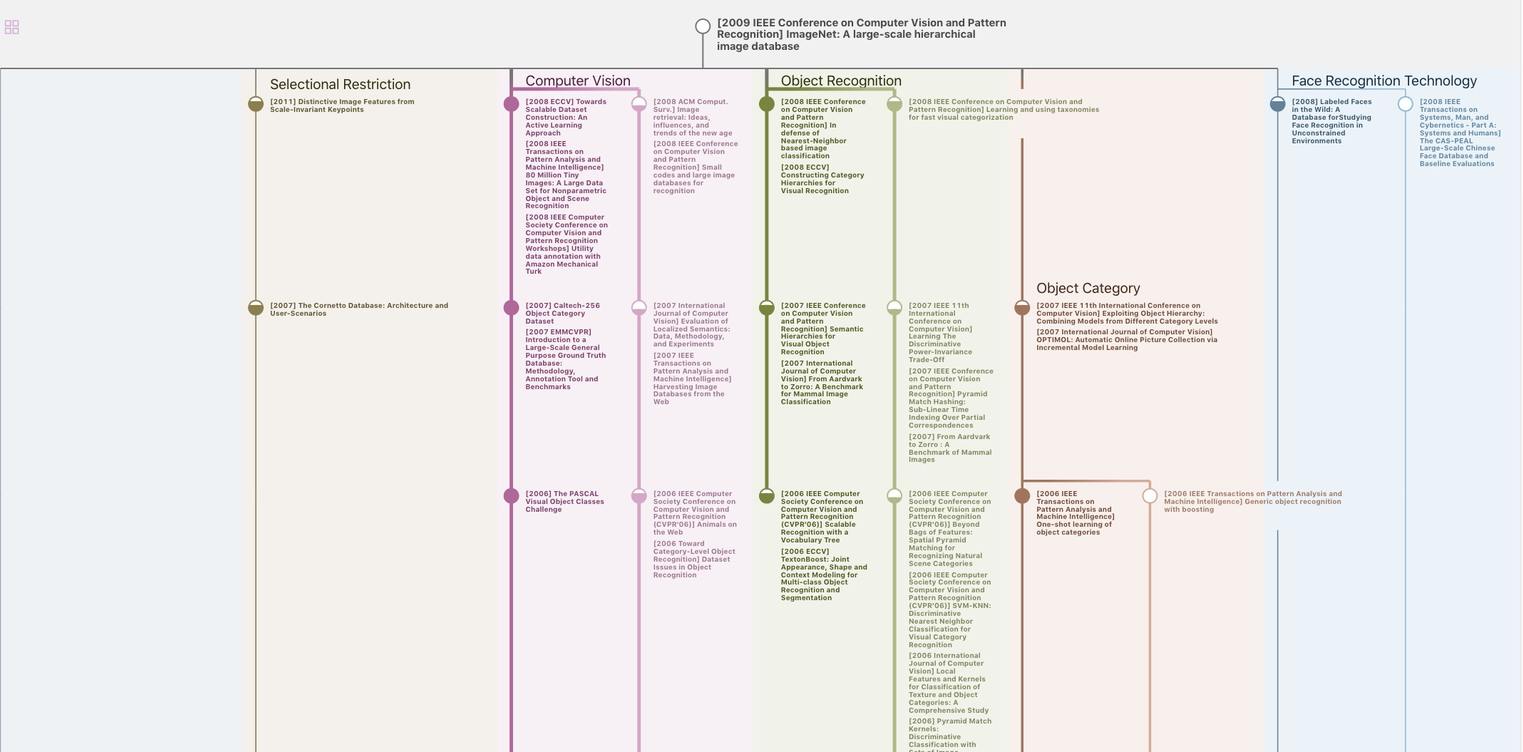
生成溯源树,研究论文发展脉络
Chat Paper
正在生成论文摘要