Reference-free mito-metagenomics using deep-learning method
crossref(2024)
摘要
The mito-metagenomics (MMG) approach inovolves directly sequencing pooled samples, yields numerous mitochondrial reads that can be assembled into full or partial mitogenomes. This method circumvents the challenges associated with PCR-based metabarcoding and hold significant promise in biodiversity and phylogeny study. However, a reference database is typically required to extract mito-reads/contigs and provide taxonomic or phylogenetic context, thereby limiting its applicability. In this study, we introduced a novel reference-free pipeline for MMG assembly. This approach involves assembling raw reads, utilizing a prebuilt deep-learning model to identify and extract mitochondrial contigs, and subsequently predicting and annotating protein coding genes. This pipeline has been integrated into a snakemake workflow, enabling the generation of output that is readily usable for phylogeny reconstruction in a single run. The performance tests have indicated that this new approach surpasses reference-based methods in soil nematode community profiling. The taxa that remain unrecovered can be attributed to various factors such as low DNA quantity and unsuccessful DNA extraction. We have demonstrated that assembly quality improves with increasing sequencing depth, recommending an average of 1–2 Gb per species to achieve acceptable MMG assembly. Our pipeline presents an opportunity to create high-resolution phylogenies and assess diversity for poorly understood taxa, including neglected microscopic eukaryotes. This advancement opens up avenues for enhanced understanding and exploration of these lesser-known organisms.
更多查看译文
AI 理解论文
溯源树
样例
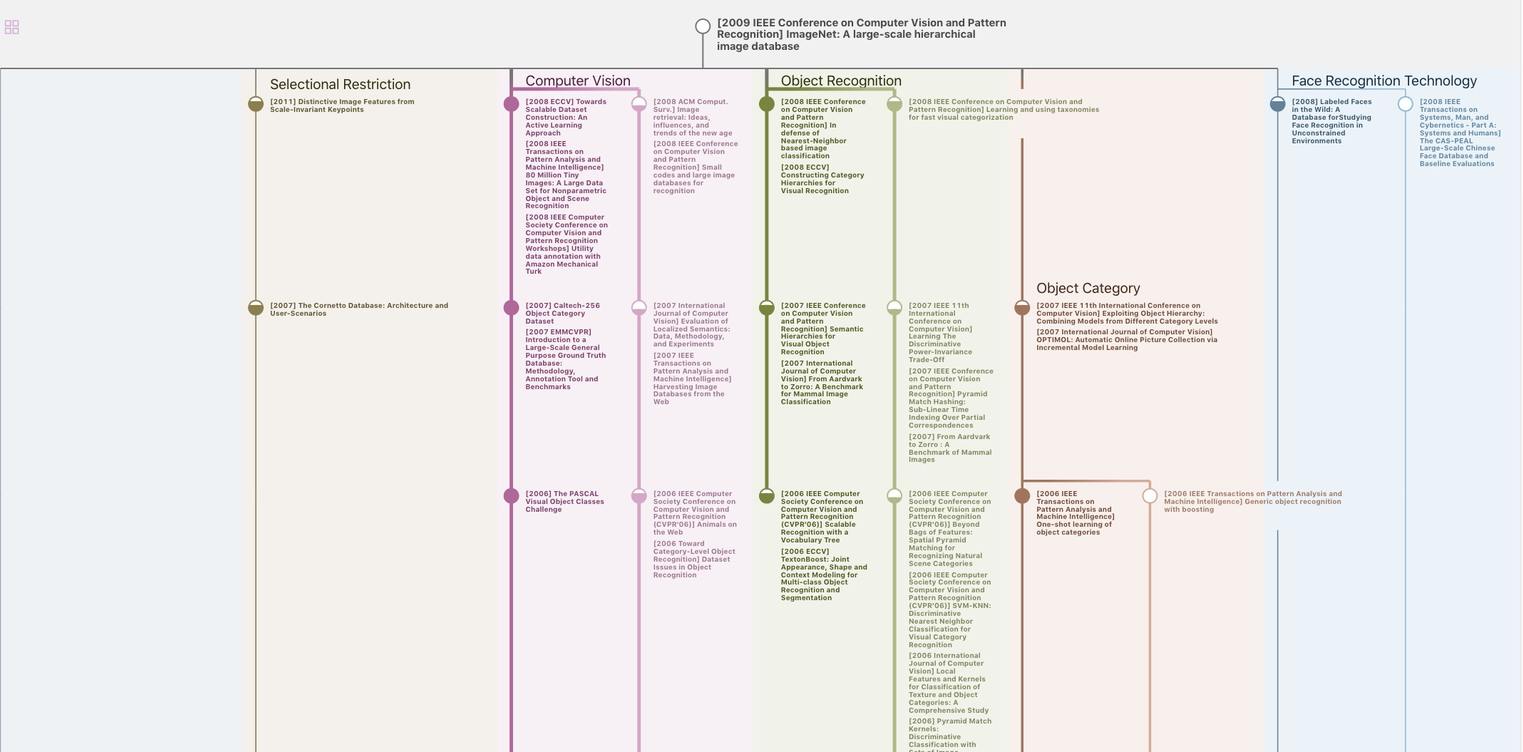
生成溯源树,研究论文发展脉络
Chat Paper
正在生成论文摘要