CantTalkAboutThis: Aligning Language Models to Stay on Topic in Dialogues
arXiv (Cornell University)(2024)
摘要
Recent advancements in instruction-tuning datasets have predominantly focusedon specific tasks like mathematical or logical reasoning. There has been anotable gap in data designed for aligning language models to maintain topicrelevance in conversations - a critical aspect for deploying chatbots toproduction. We introduce the CantTalkAboutThis dataset to help language modelsremain focused on the subject at hand during task-oriented interactions. Itconsists of synthetic dialogues on a wide range of conversation topics fromdifferent domains. These dialogues are interspersed with distractor turns thatintentionally divert the chatbot from the predefined topic. Fine-tuninglanguage models on this dataset helps make them resilient to deviating from therole assigned and improves their ability to maintain topical coherence comparedto general-purpose instruction-tuned LLMs like GPT-4-turbo andMixtral-Instruct. Additionally, preliminary observations suggest that trainingmodels on this dataset also enhance their performance on fine-grainedinstruction following tasks, including safety alignment.
更多查看译文
AI 理解论文
溯源树
样例
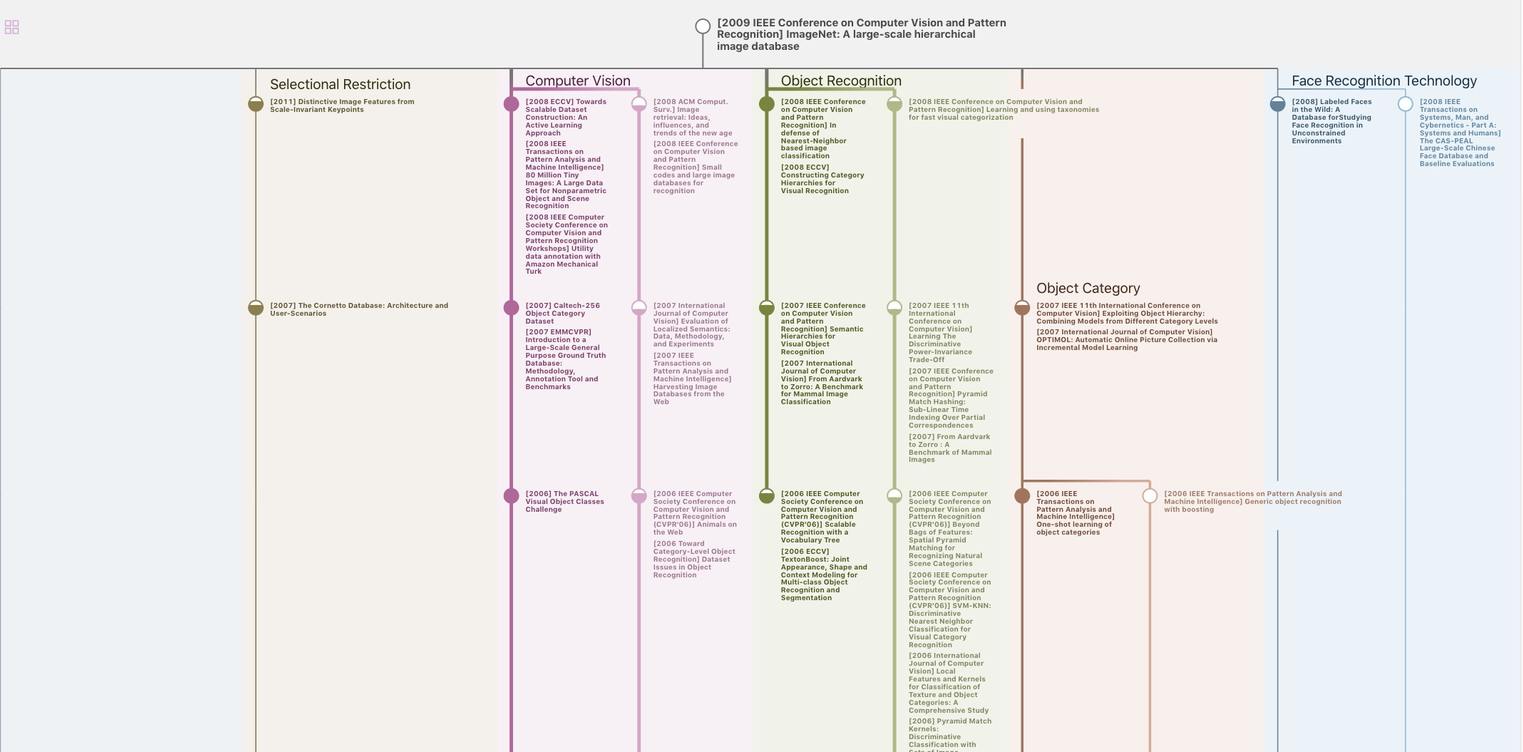
生成溯源树,研究论文发展脉络
Chat Paper
正在生成论文摘要