Uniform Memory Retrieval with Larger Capacity for Modern Hopfield Models
arxiv(2024)
摘要
We propose a two-stage memory retrieval dynamics for modern Hopfield models,
termed 𝚄-𝙷𝚘𝚙, with enhanced memory capacity. Our key
contribution is a learnable feature map Φ which transforms the Hopfield
energy function into a kernel space. This transformation ensures convergence
between the local minima of energy and the fixed points of retrieval dynamics
within the kernel space. Consequently, the kernel norm induced by Φ serves
as a novel similarity measure. It utilizes the stored memory patterns as
learning data to enhance memory capacity across all modern Hopfield models.
Specifically, we accomplish this by constructing a separation loss
ℒ_Φ that separates the local minima of kernelized energy by
separating stored memory patterns in kernel space. Methodologically,
𝚄-𝙷𝚘𝚙 memory retrieval process consists of:
(Stage I.) minimizing separation loss for a more uniformed memory
(local minimum) distribution, followed by (Stage II.) standard
Hopfield energy minimization for memory retrieval. This results in a
significant reduction of possible meta-stable states in the Hopfield energy
function, thus enhancing memory capacity by preventing memory confusion.
Empirically, with real-world datasets, we demonstrate that
𝚄-𝙷𝚘𝚙 outperforms all existing modern Hopfield models and
SOTA similarity measures, achieving substantial improvements in both
associative memory retrieval and deep learning tasks.
更多查看译文
AI 理解论文
溯源树
样例
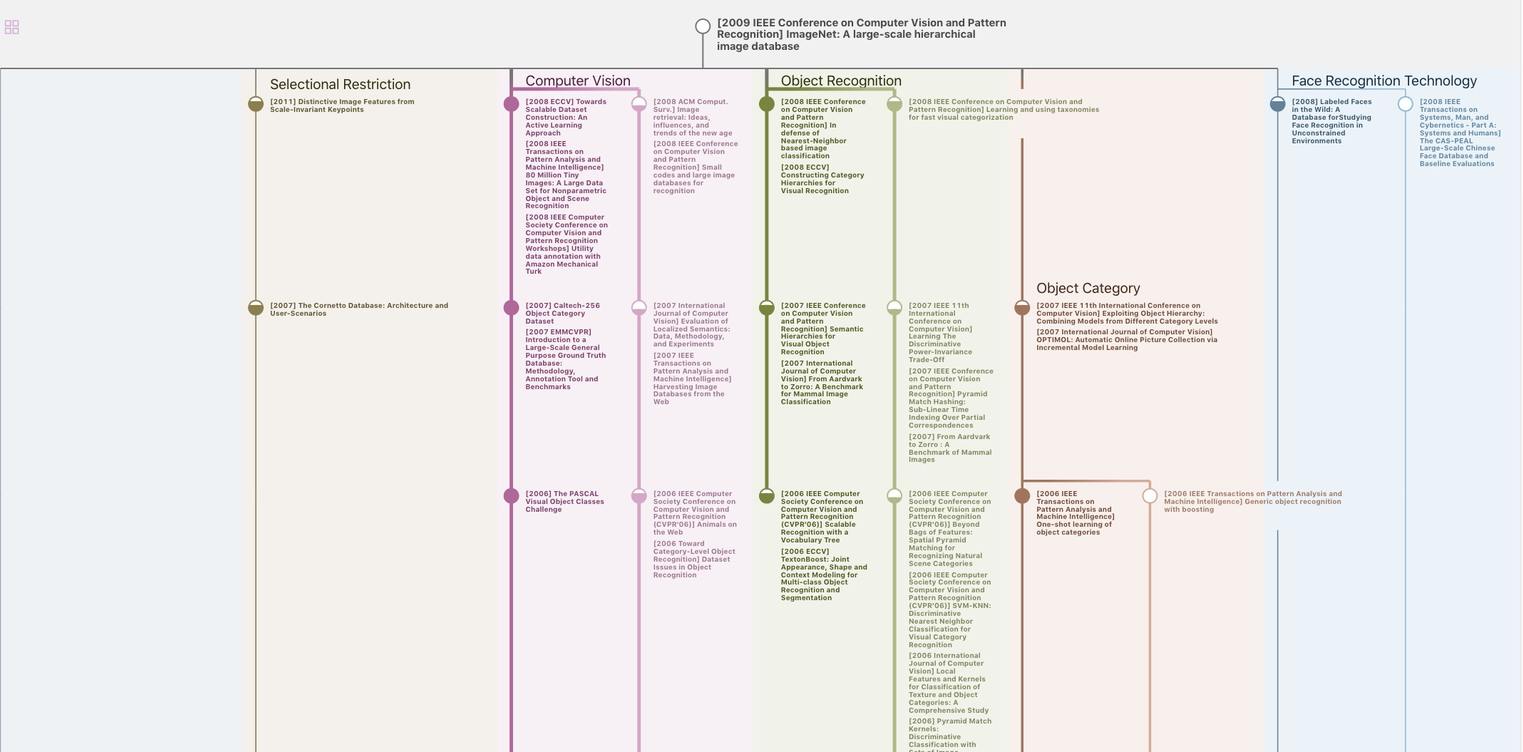
生成溯源树,研究论文发展脉络
Chat Paper
正在生成论文摘要