The ESPRIT algorithm under high noise: Optimal error scaling and noisy super-resolution
arxiv(2024)
摘要
Subspace-based signal processing techniques, such as the Estimation of Signal
Parameters via Rotational Invariant Techniques (ESPRIT) algorithm, are popular
methods for spectral estimation. These algorithms can achieve the so-called
super-resolution scaling under low noise conditions, surpassing the well-known
Nyquist limit. However, the performance of these algorithms under high-noise
conditions is not as well understood. Existing state-of-the-art analysis
indicates that ESPRIT and related algorithms can be resilient even for signals
where each observation is corrupted by statistically independent, mean-zero
noise of size 𝒪(1), but these analyses only show that the error
ϵ decays at a slow rate ϵ=Õ(n^-1/2) with
respect to the cutoff frequency n. In this work, we prove that under certain
assumptions of bias and high noise, the ESPRIT algorithm can attain a
significantly improved error scaling ϵ =
Õ(n^-3/2), exhibiting noisy super-resolution scaling
beyond the Nyquist limit. We further establish a theoretical lower bound and
show that this scaling is optimal. Our analysis introduces novel matrix
perturbation results, which could be of independent interest.
更多查看译文
AI 理解论文
溯源树
样例
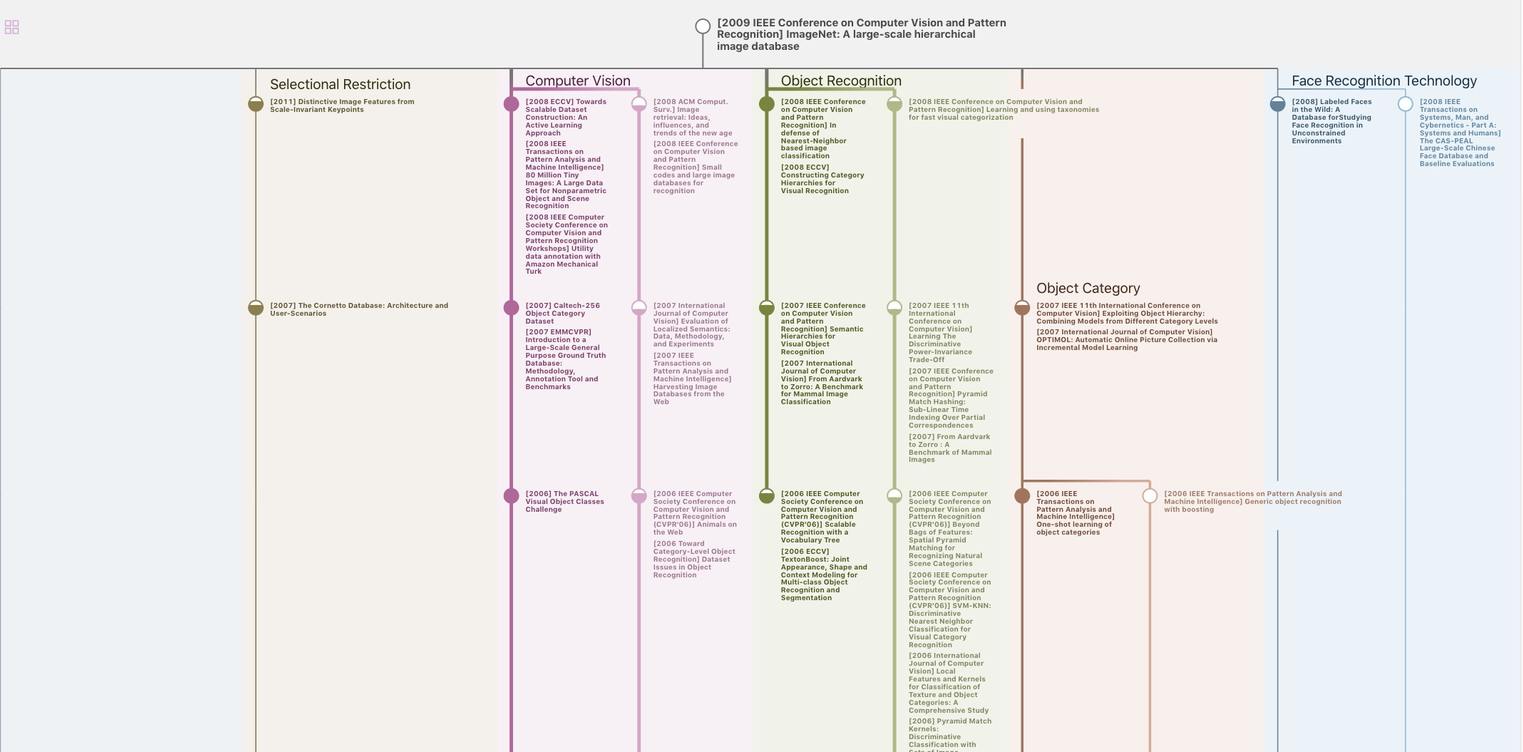
生成溯源树,研究论文发展脉络
Chat Paper
正在生成论文摘要