Deep learning-based phenotype classification of three ark shells: Anadara kagoshimensis, Tegillarca granosa, and Anadara broughtonii
FRONTIERS IN MARINE SCIENCE(2024)
摘要
The rapid and accurate classification of aquatic products is crucial for ensuring food safety, production efficiency, and economic benefits. However, traditional manual methods for classifying ark shell species based on phenotype are time-consuming and inefficient, especially during peak seasons when the demand is high and labor is scarce. This study aimed to develop a deep learning model for the automated identification and classification of commercially important three ark shells (Tegillarca granosa, Anadara broughtonii, and Anadara kagoshimensis) from images. The ark shells were collected and identified using a polymerase chain reaction method developed in a previous study, and a total of 1,400 images were categorized into three species. Three convolutional neural network (CNN) models, Visual Geometry Group Network (VGGnet), Inception-Residual Network (ResNet), and SqueezeNet, were then applied to two different classification sets, Set-1 (four bivalve species) and Set-2 (three ark shell species). Our results showed that SqueezeNet demonstrated the highest accuracy during the training phase for both classification sets, whereas Inception-ResNet exhibited superior accuracy during the validation phase. Similar results were obtained after developing a third classification set (Set-3) to classify six categories by combining Set-1 and Set-2. Overall, the developed CNN-based classification model exhibited a performance comparable or superior to that presented in previous studies and can provide a theoretical basis for bivalve classification, thereby contributing to improved food safety, production efficiency, and economic benefits in the aquatic products industry.
更多查看译文
关键词
Anadara kagoshimensis,Tegillarca granosa,Anadara broughtonii,convolutional neural networks,food fraud,image classification
AI 理解论文
溯源树
样例
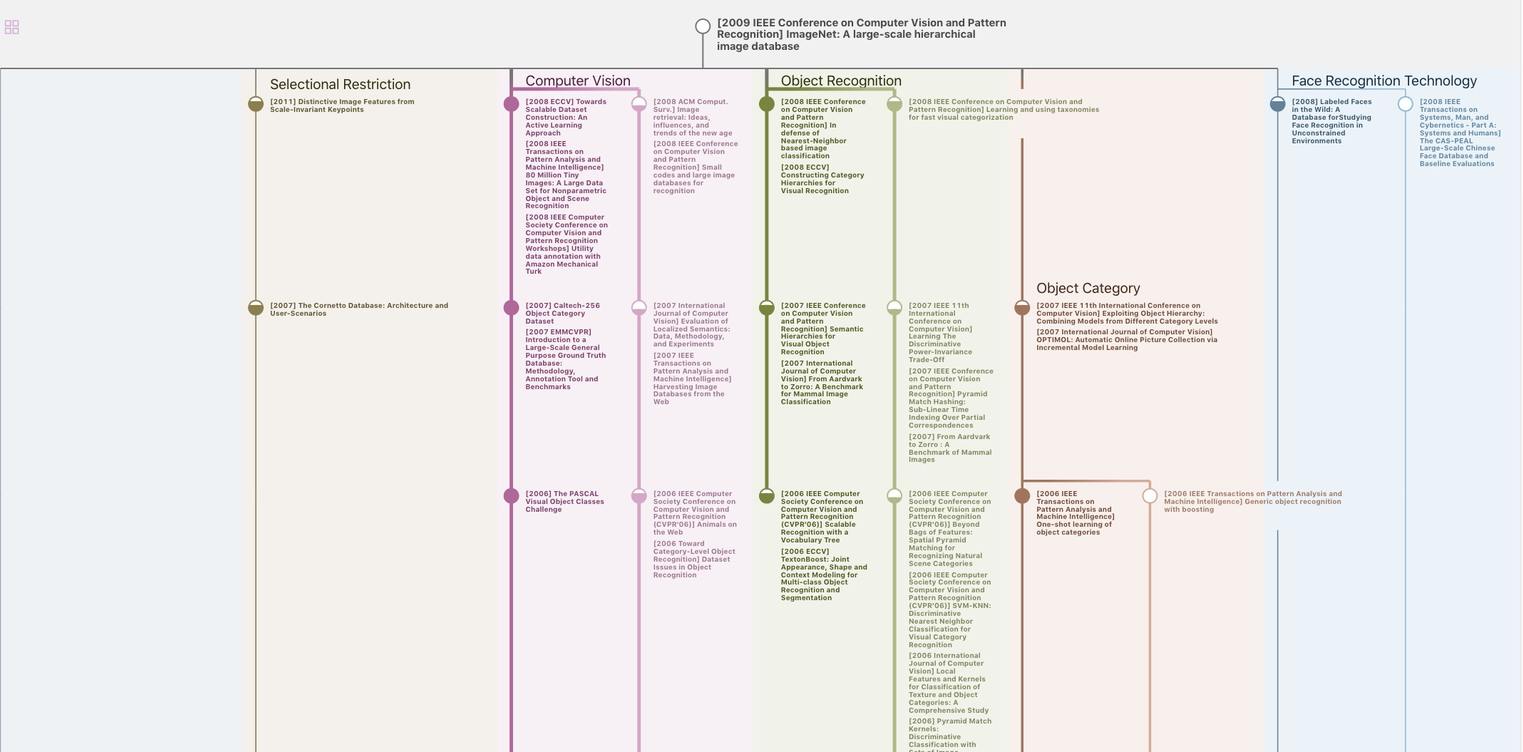
生成溯源树,研究论文发展脉络
Chat Paper
正在生成论文摘要