Prompt-based and Fine-tuned GPT Models for Context-Dependent and -Independent Deductive Coding in Social Annotation
FOURTEENTH INTERNATIONAL CONFERENCE ON LEARNING ANALYTICS & KNOWLEDGE, LAK 2024(2024)
摘要
GPT has demonstrated impressive capabilities in executing various natural language processing (NLP) and reasoning tasks, showcasing its potential for deductive coding in social annotations. This research explored the effectiveness of prompt engineering and fine-tuning approaches of GPT for deductive coding of contextdependent and context-independent dimensions. Coding contextdependent dimensions (i.e., Theorizing, Integration, Reflection) requires a contextualized understanding that connects the target comment with reading materials and previous comments, whereas coding context-independent dimensions (i.e., Appraisal, Questioning, Social, Curiosity, Surprise) relies more on the comment itself. Utilizing strategies such as prompt decomposition, multi-prompt learning, and a codebook-centered approach, we found that prompt engineering can achieve fair to substantial agreement with expertlabeled data across various coding dimensions. These results affirm GPT ' s potential for effective application in real-world coding tasks. Compared to context-independent coding, context-dependent dimensions had lower agreement with expert-labeled data. To enhance accuracy, GPT models were fine-tuned using 102 pieces of expert-labeled data, with an additional 102 cases used for validation. The fine-tuned models demonstrated substantial agreement with ground truth in context-independent dimensions and elevated the inter-rater reliability of context-dependent categories to moderate levels. This approach represents a promising path for significantly reducing human labor and time, especially with large unstructured datasets, without sacrificing the accuracy and reliability of deductive coding tasks in social annotation. The study marks a step toward optimizing and streamlining coding processes in social annotation. Our findings suggest the promise of using GPT to analyze qualitative data and provide detailed, immediate feedback for students to elicit deepening inquiries.
更多查看译文
关键词
GPT,Prompt Engineering,Fine-tuning,Social Annotation,ContextDependent,deductive coding
AI 理解论文
溯源树
样例
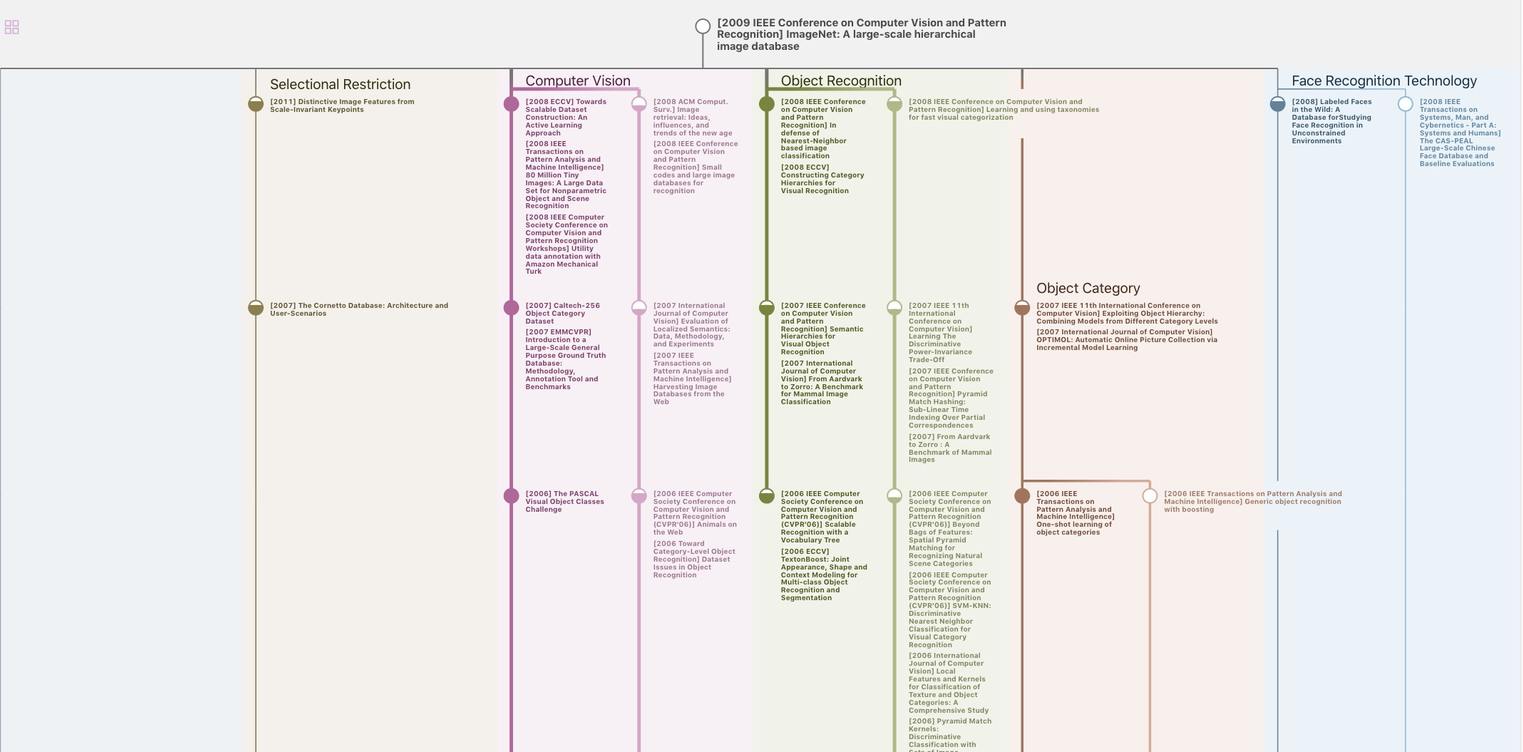
生成溯源树,研究论文发展脉络
Chat Paper
正在生成论文摘要