Effecti-Net: A Multimodal Framework and Database for Educational Content Effectiveness Analysis
FOURTEENTH INTERNATIONAL CONFERENCE ON LEARNING ANALYTICS & KNOWLEDGE, LAK 2024(2024)
摘要
Amid the evolving landscape of education, evaluating the impact of educational video content on students remains a challenge. Existing methods for assessment often rely on heuristics and self-reporting, leaving room for subjectivity and limited insight. This study addresses this issue by leveraging physiological sensor data to predict student-perceived content effectiveness. Within the realm of educational content evaluation, prior studies focused on conventional approaches, leaving a gap in understanding the nuanced responses of students to educational materials. To bridge this gap, our research introduces a novel perspective, building upon previous work in multimodal physiological data analysis. Our primary contributions encompass two key elements. First, we present the 'Effecti-Net' architecture, a sophisticated deep learning model that integrates data from multiple sensor modalities, including Electroencephalogram (EEG), Eye Tracker, Galvanic Skin Response (GSR), and Photoplethysmography (PPG). Second, we introduce the 'DECEP' dataset, a repository comprising 597 minutes of multimodal sensor data. To assess the effectiveness of our approach, we benchmark it against conventional methods. Remarkably, our model achieves a lowest MSE of 0.1651 and MAE of 0.3544 on the DECEP dataset. It offers educators and content creators a comprehensive framework that promotes the development of more engaging educational content.
更多查看译文
关键词
Multi-Modal frameworks,Deep Learning,Machine Learning,Affective,Datasets,User Studies,Physiological Data Analysis
AI 理解论文
溯源树
样例
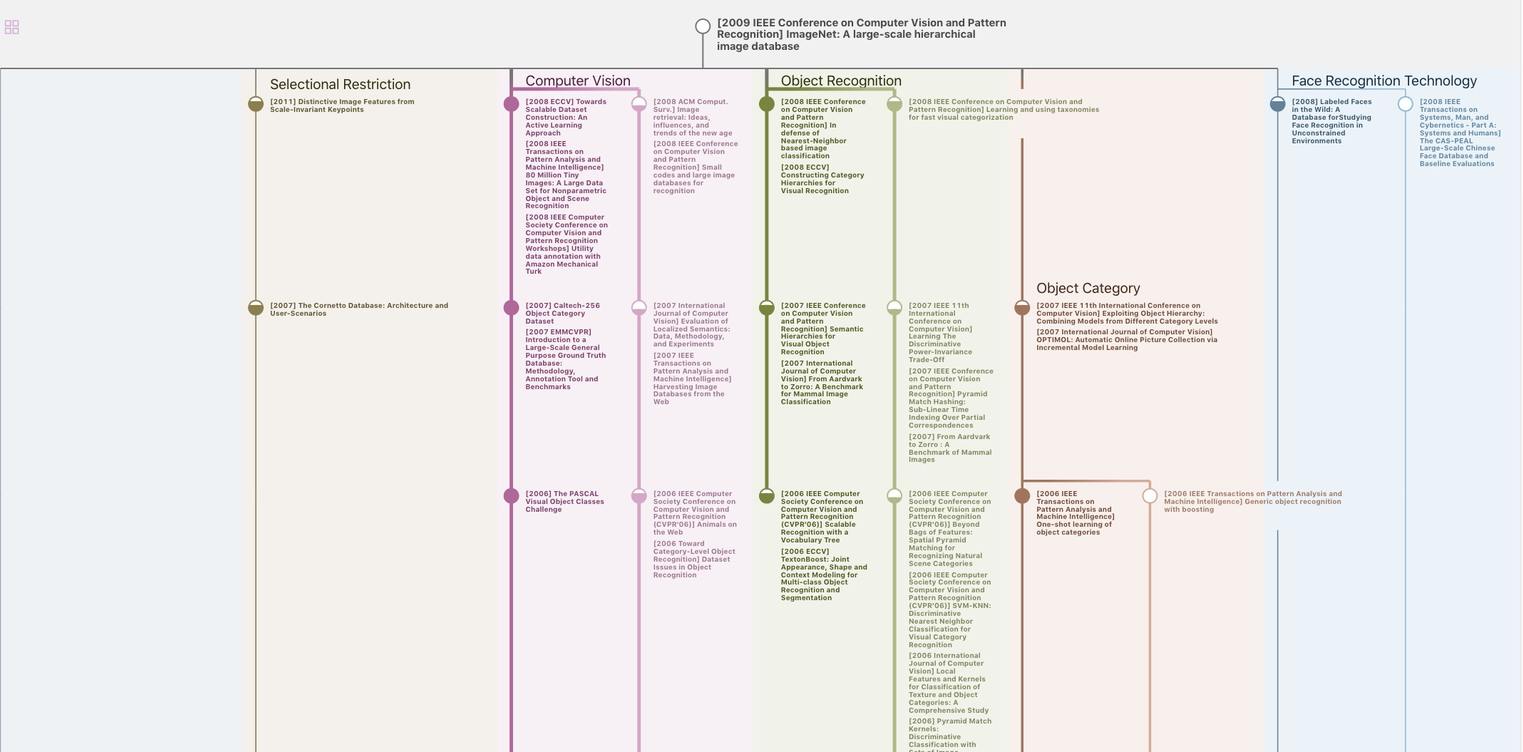
生成溯源树,研究论文发展脉络
Chat Paper
正在生成论文摘要