A Modular Framework for FAIR Shallow Landslide Susceptibility Mapping Based on Machine Learning
NATURAL HAZARDS(2024)
摘要
Machine learning has grown in popularity in the past few years for susceptibility and hazard mapping tasks. Necessary steps for the generation of a susceptibility or hazard map are repeatedly implemented in new studies. We present a Random Forest classifier-based landslide susceptibility and hazard mapping framework to facilitate future mapping studies using machine learning. The framework, as a piece of software, follows the FAIR paradigm, and hence is set up as a transparent, reproducible and modularly extensible workflow. It contains pre-implemented steps from conceptualisation to map generation, such as the generation of input datasets. The framework can be applied to different areas of interest using different environmental features and is also flexible in terms of the desired scale and resolution of the final map. To demonstrate the functionality and validity of the framework, and to explore the challenges and limitations of Random Forest-based susceptibility and hazard mapping, we apply the framework to a test case. This test case conveys the influence of the training dataset on the generated susceptibility maps in terms of feature combination, influence of non-landslide instances and representativeness of the training data with respect to the area of interest. A comparison of the test case results with the literature shows that the framework works reliably. Furthermore, the results obtained in this study complement the findings of previous studies that demonstrate the sensitivity of the training process to the training data, particularly in terms of its representativeness.
更多查看译文
关键词
Shallow landslides,Hazard mapping,Machine learning,Random Forest,FAIR data,Shallow landslide susceptibility
AI 理解论文
溯源树
样例
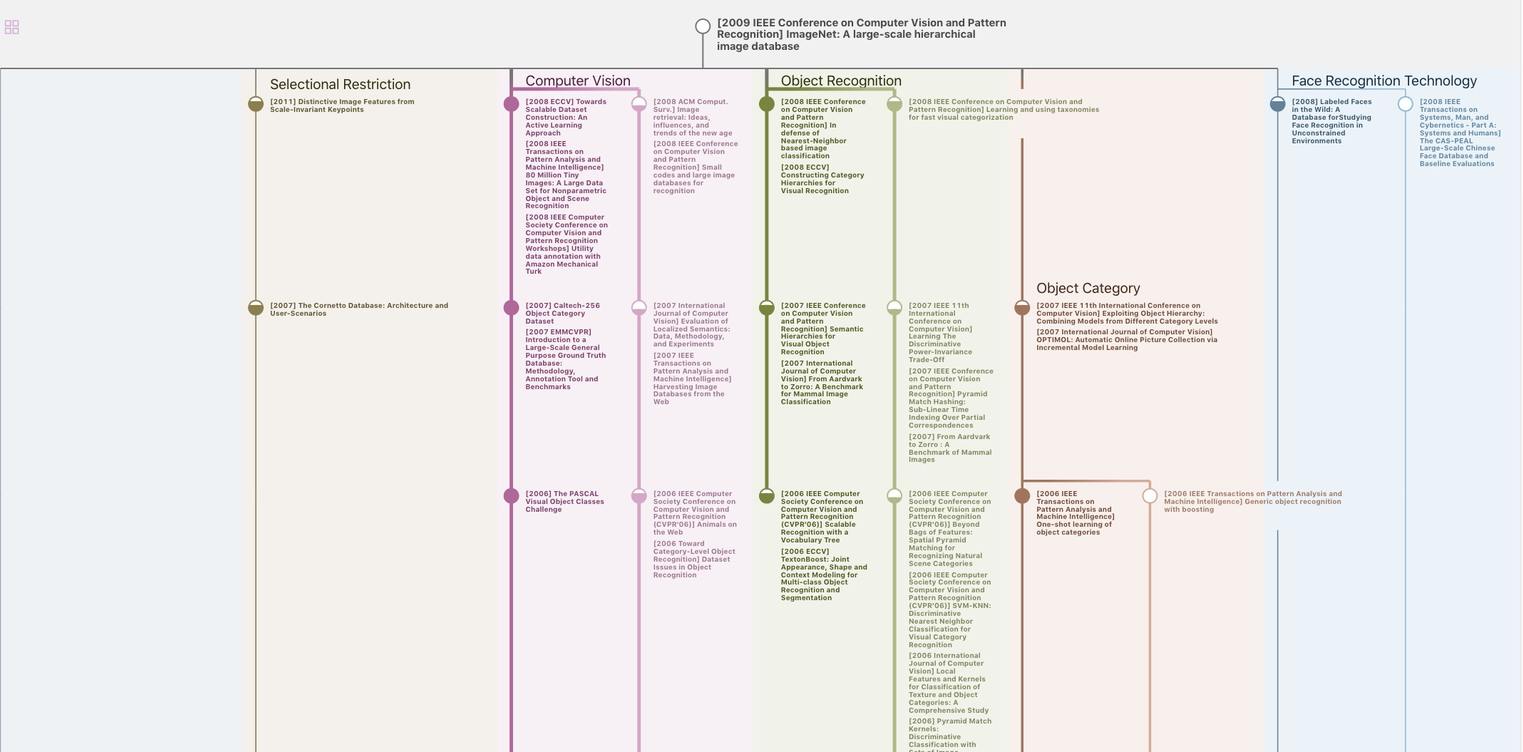
生成溯源树,研究论文发展脉络
Chat Paper
正在生成论文摘要