Identification of Distinct Immune Signatures in Inclusion Body Myositis by Peripheral Blood Immunophenotyping Using Machine Learning Models.
Clinical & translational immunology(2024)
摘要
ObjectiveInclusion body myositis (IBM) is a progressive late-onset muscle disease characterised by preferential weakness of quadriceps femoris and finger flexors, with elusive causes involving immune, degenerative, genetic and age-related factors. Overlapping with normal muscle ageing makes diagnosis and prognosis problematic.MethodsWe characterised peripheral blood leucocytes in 81 IBM patients and 45 healthy controls using flow cytometry. Using a random forest classifier, we identified immune changes in IBM compared to HC. K-means clustering and the random forest one-versus-rest model classified patients into three immunophenotypic clusters. Functional outcome measures including mTUG, 2MWT, IBM-FRS, EAT-10, knee extension and grip strength were assessed across clusters.ResultsThe random forest model achieved a 94% AUC ROC with 82.76% specificity and 100% sensitivity. Significant differences were found in IBM patients, including increased CD8+ T-bet+ cells, CD4+ T cells skewed towards a Th1 phenotype and altered gamma delta T cell repertoire with a reduced proportion of V gamma 9+V delta 2+ cells. IBM patients formed three clusters: (i) activated and inflammatory CD8+ and CD4+ T-cell profile and the highest proportion of anti-cN1A-positive patients in cluster 1; (ii) limited inflammation in cluster 2; (iii) highly differentiated, pro-inflammatory T-cell profile in cluster 3. Additionally, no significant differences in patients' age and gender were detected between immunophenotype clusters; however, worsening trends were detected with several functional outcomes.ConclusionThese findings unveil distinct immune profiles in IBM, shedding light on underlying pathological mechanisms for potential immunoregulatory therapeutic development. Peripheral blood leucocytes from 81 inclusion body myositis (IBM) patients and 45 healthy controls were analysed using flow cytometry. A supervised computational approach using the random forest classifier identified immune signatures in IBM, while unsupervised modelling stratified patients into distinct immunophenotypic clusters. These findings shed light on the underlying pathological mechanisms and hold potential for future clinical applications, spanning IBM diagnosis, prognosis and management.a image
更多查看译文
关键词
AI,IBM,inflammatory myopathies,machine learning,random forest
AI 理解论文
溯源树
样例
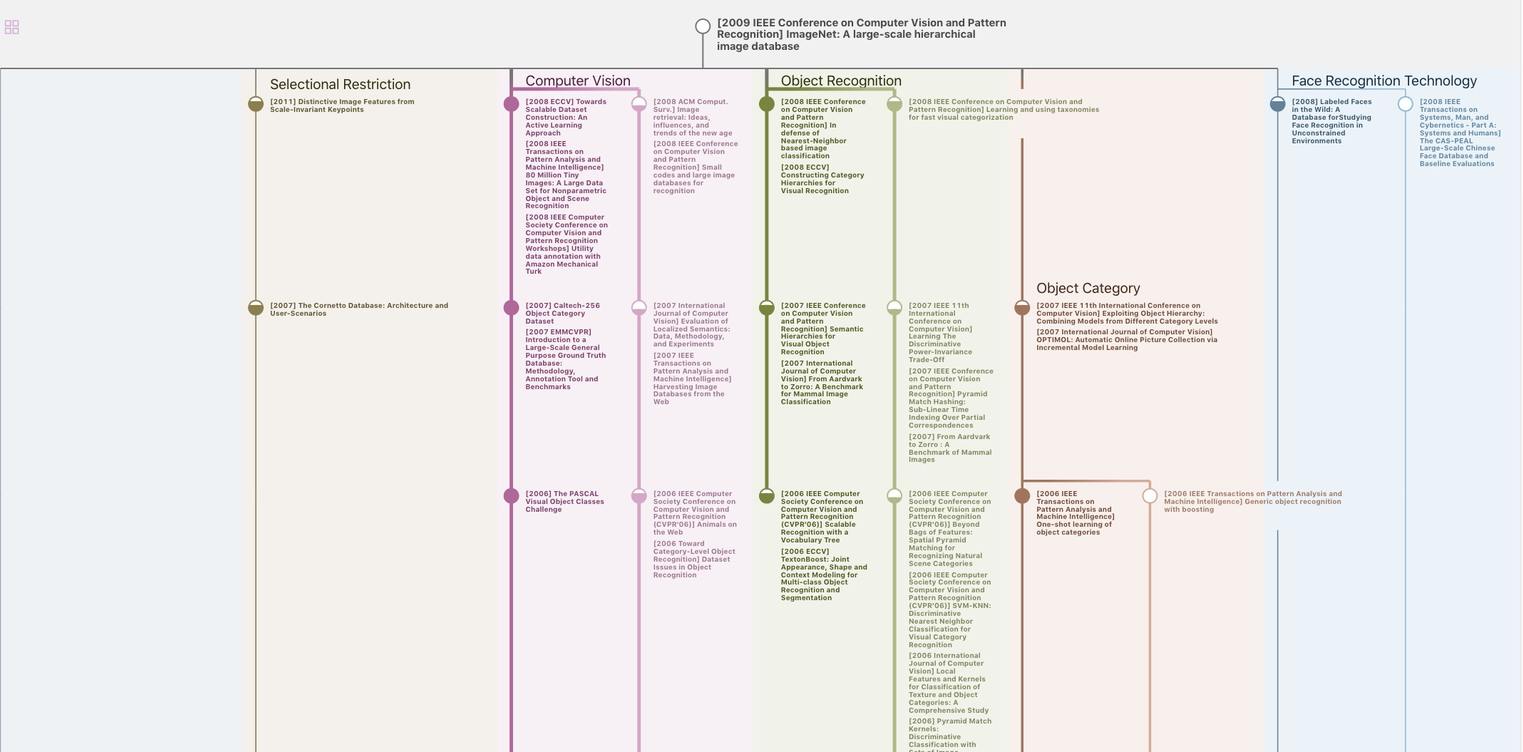
生成溯源树,研究论文发展脉络
Chat Paper
正在生成论文摘要