Unbinding tensor product representations for image captioning with semantic alignment and complementation
Multimedia Systems(2024)
摘要
Image captioning, which describes an image with natural language, is an important but challenging multi-modal task. Many state-of-the-art methods generally adopt the encoder–decoder framework to implement information conversion from image modality to text modality. However, most methods are limited by the local view during encoding and lack consideration of word organization logic during decoding, prone to generating captions that are patchworks of the salient visual content and relying on high-frequency expression templates subject to the dataset bias. To alleviate the phenomenon, we propose a novel encoding–decoding-based image captioning method, unbinding tensor product representations for image captioning with semantic alignment and complementation (uTPR-SAC). uTPR-SAC acquires the semantic content reflecting the global cognition of the images through semantic alignment based on the common subspace projection. The structural information of visual features are complemented by guidance of semantic content, which helps to generate the intermediate representations with the deep semantic understanding. To avoid the dependence on high-frequency templates, the unbinding operation of TPR optimizes the word prediction by reasoning word structures with both an orthogonal structure matrix and visual structure information of the intermediate representations. Comparison with other state-of-the-art methods at MSCOCO validates the competitiveness and effectiveness of uTPR-SAC, where it, respectively, reaches 81.0, 65.9, 51.7, 39.8 and 59.4 on BLEU-1, 2, 3, 4, and ROUGE-L. Extensive visualization experiments not only show the sensitivity of semantic content to important visual content, but also demonstrate the validity of the word structures obtained by unbinding, both of which contribute to the semantic accuracy of the generated captions.
更多查看译文
关键词
Image captioning,Tensor product representations,Semantic content,Intermediate representations
AI 理解论文
溯源树
样例
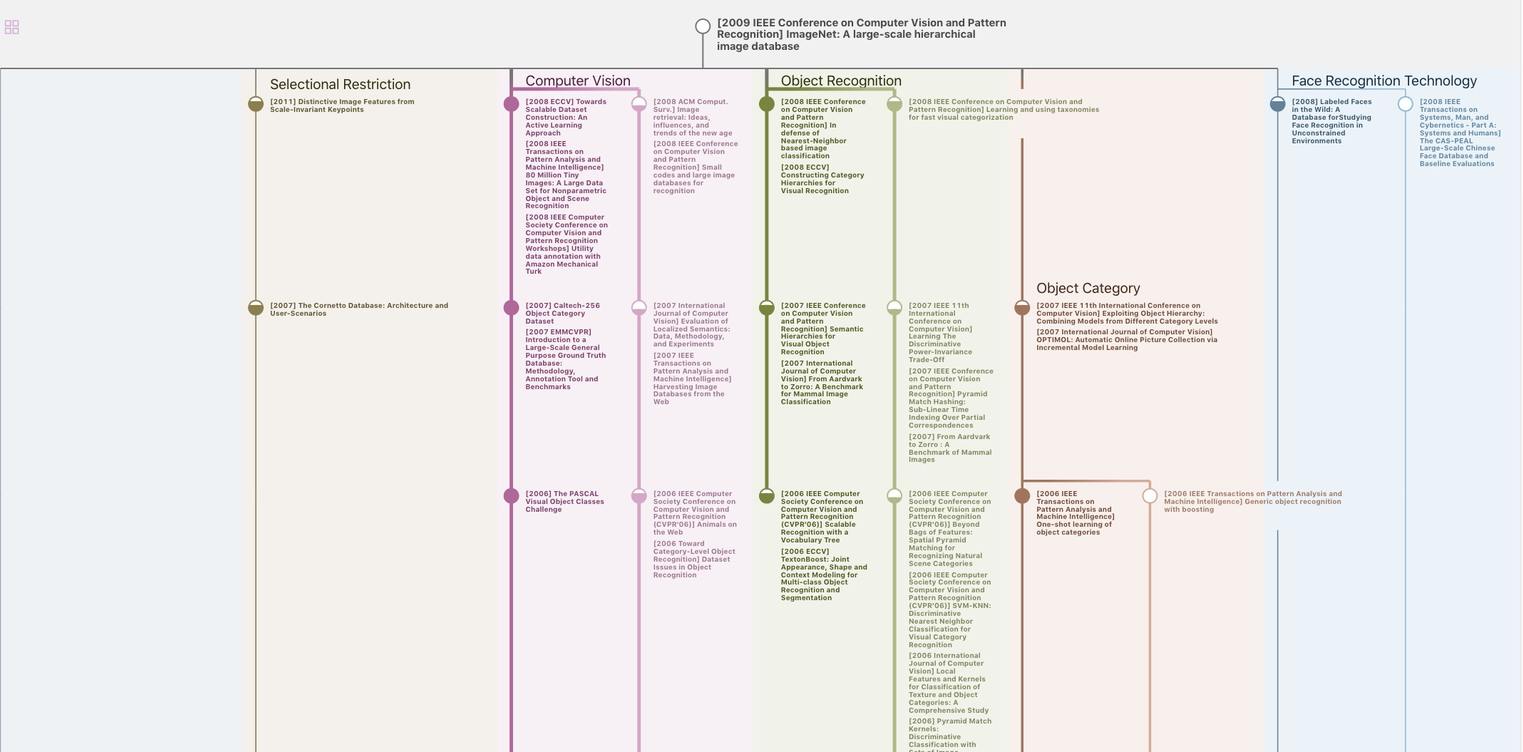
生成溯源树,研究论文发展脉络
Chat Paper
正在生成论文摘要