Performance of Commercial Dermatoscopic Systems That Incorporate Artificial Intelligence for the Identification of Melanoma in General Practice: A Systematic Review
CANCERS(2024)
摘要
Simple Summary Early detection of malignant melanoma (MM) has the potential to significantly reduce morbidity and mortality as the thickness of the lesion is closely associated with prognosis. Artificial intelligence (AI) is a non-invasive technology that has the potential to aid clinicians in the early diagnosis of skin cancers, including melanoma. Performance metrics of machine-based AI have been shown to rival or improve upon clinician diagnosis. However, many studies have limited this scope to pre-build image databases that may not replicate real-world settings experienced in general practice. This systematic review aimed to report the performance of commercial or market-approved dermatoscopic systems with AI in the form of convolutional neural networks (CNNs) when tasked with classifying melanoma. The sensitivity and specificity of CNNs are highly varied and illustrate the necessity of clinician-to-patient interaction in the diagnosis process. Clinicians working in unison with AI show the most promise for better performance when classifying MM.Abstract Background: Cutaneous melanoma remains an increasing global public health burden, particularly in fair-skinned populations. Advancing technologies, particularly artificial intelligence (AI), may provide an additional tool for clinicians to help detect malignancies with a more accurate success rate. This systematic review aimed to report the performance metrics of commercially available convolutional neural networks (CNNs) tasked with detecting MM. Methods: A systematic literature search was performed using CINAHL, Medline, Scopus, ScienceDirect and Web of Science databases. Results: A total of 16 articles reporting MM were included in this review. The combined number of melanomas detected was 1160, and non-melanoma lesions were 33,010. The performance of market-approved technology and clinician performance for classifying melanoma was highly heterogeneous, with sensitivity ranging from 16.4 to 100.0%, specificity between 40.0 and 98.3% and accuracy between 44.0 and 92.0%. Less heterogeneity was observed when clinicians worked in unison with AI, with sensitivity ranging between 83.3 and 100.0%, specificity between 83.7 and 87.3%, and accuracy between 86.4 and 86.9%. Conclusion: Instead of focusing on the performance of AI versus clinicians for classifying melanoma, more consistent performance has been obtained when clinicians' work is supported by AI, facilitating management decisions and improving health outcomes.
更多查看译文
关键词
computer-aided diagnosis,convolutional neural network,deep learning,dermatology,detection,diagnosis,epidemiology,machine learning,skin cancer,total body photography
AI 理解论文
溯源树
样例
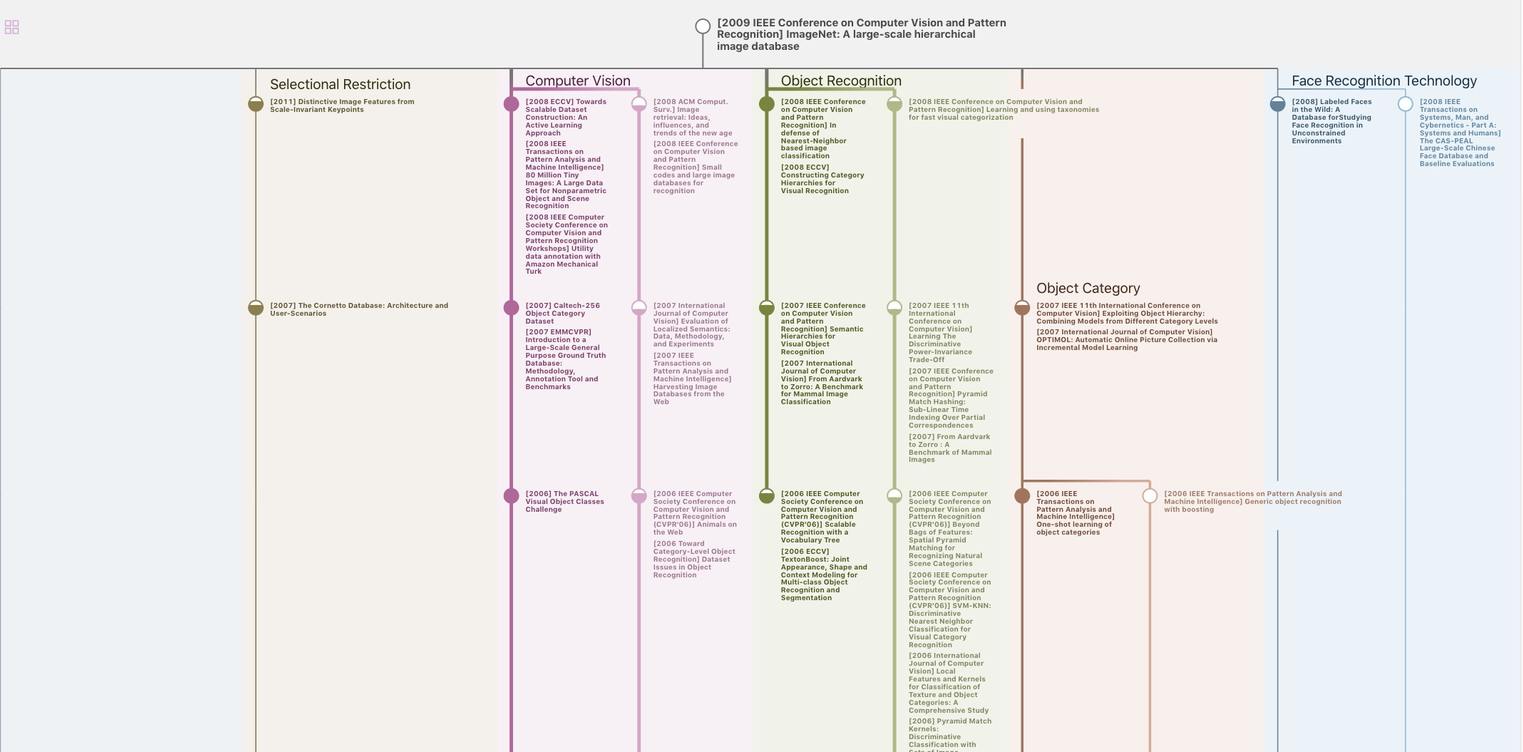
生成溯源树,研究论文发展脉络
Chat Paper
正在生成论文摘要