Local Extremum Constrained Total Variation Model for Natural and Hyperspectral Image Non-Blind Deblurring
IEEE Transactions on Circuits and Systems for Video Technology(2024)
摘要
Blurring and noise degrade the performance of image processing. To mitigate this effect, various regularization-based deblurring methods have been proposed. Total variation regularization is widely used owing to its excellent ability in preserving the salient edges, but it also tends to smooth the image details. In this paper, we propose a local extremum-constrained total variation (LECTV) framework for image deblurring. In the developed deblurring framework, we integrate prior knowledge of the dark channel with the structural features of the image into a single regularization term. Furthermore, unlike most existing methods that focus on the overall sparsity of the dark channel, the defined regularization term allows for a pixel-wise adaptive description of the image to restore its inherent spatial texture structure. Finally, a majorization-minimization-based method is designed to solve the developed LECTV framework. Experimental results on natural and hyperspectral images show that the designed framework exhibits excellent performance in removing multiple types and degrees of blurring. Extensive evaluations also further show its superiority compared to other advanced methods.
更多查看译文
关键词
Non-blind image deblurring,hyperspectral image (HSI),local extremum constraints,total variation (TV)
AI 理解论文
溯源树
样例
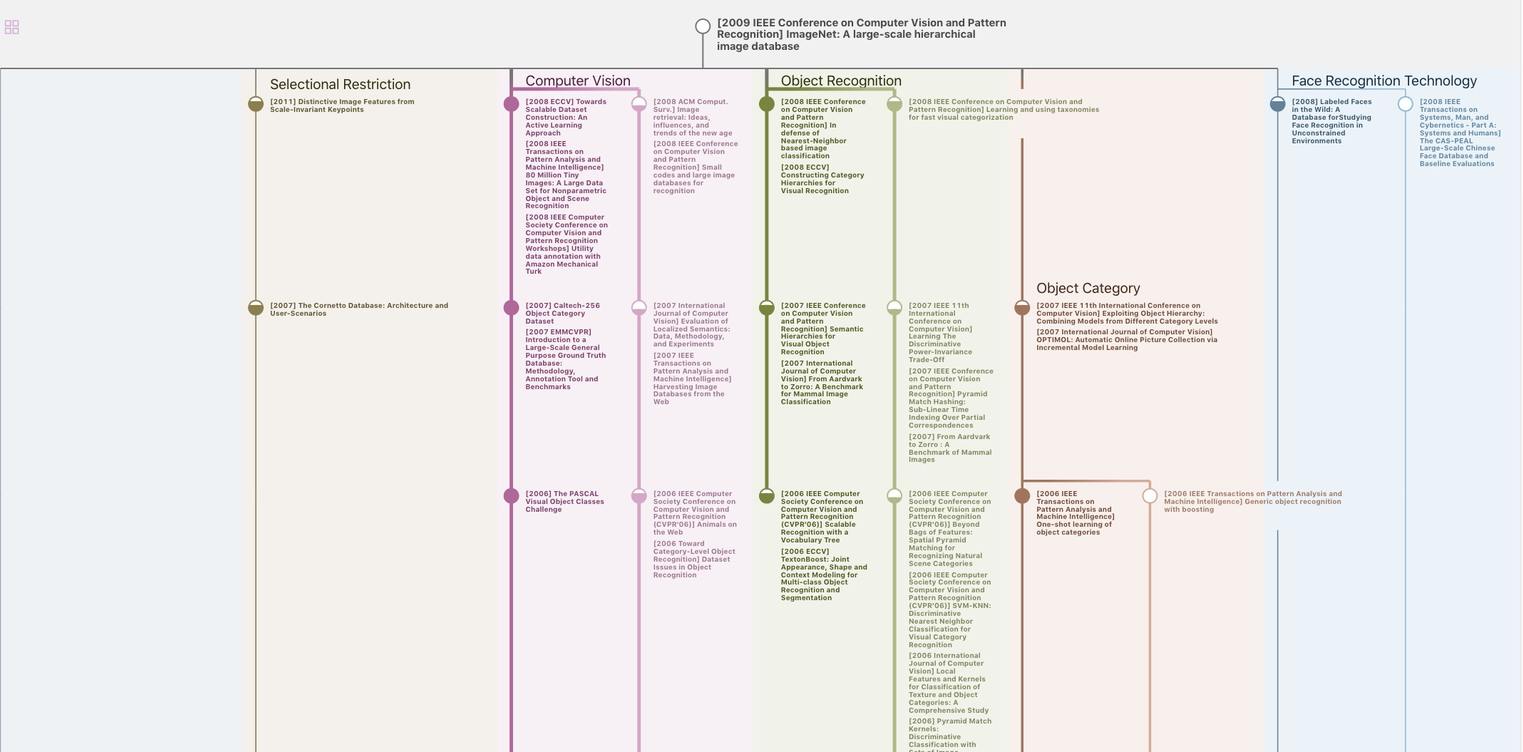
生成溯源树,研究论文发展脉络
Chat Paper
正在生成论文摘要