Parameter Efficient Quasi-Orthogonal Fine-Tuning via Givens Rotation
arxiv(2024)
摘要
With the increasingly powerful performances and enormous scales of Pretrained
Language Models (PLMs), promoting parameter efficiency in fine-tuning has
become a crucial need for effective and efficient adaptation to various
downstream tasks. One representative line of fine-tuning methods is Orthogonal
Fine-tuning (OFT), which rigorously preserves the angular distances within the
parameter space to preserve the pretrained knowledge. Despite the empirical
effectiveness, OFT still suffers low parameter efficiency at 𝒪(d^2)
and limited capability of downstream adaptation. Inspired by Givens rotation,
in this paper, we proposed quasi-Givens Orthogonal Fine-Tuning (qGOFT) to
address the problems. We first use 𝒪(d) Givens rotations to
accomplish arbitrary orthogonal transformation in SO(d) with provable
equivalence, reducing parameter complexity from 𝒪(d^2) to
𝒪(d). Then we introduce flexible norm and relative angular
adjustments under soft orthogonality regularization to enhance the adaptation
capability of downstream semantic deviations. Extensive experiments on various
tasks and PLMs validate the effectiveness of our methods.
更多查看译文
AI 理解论文
溯源树
样例
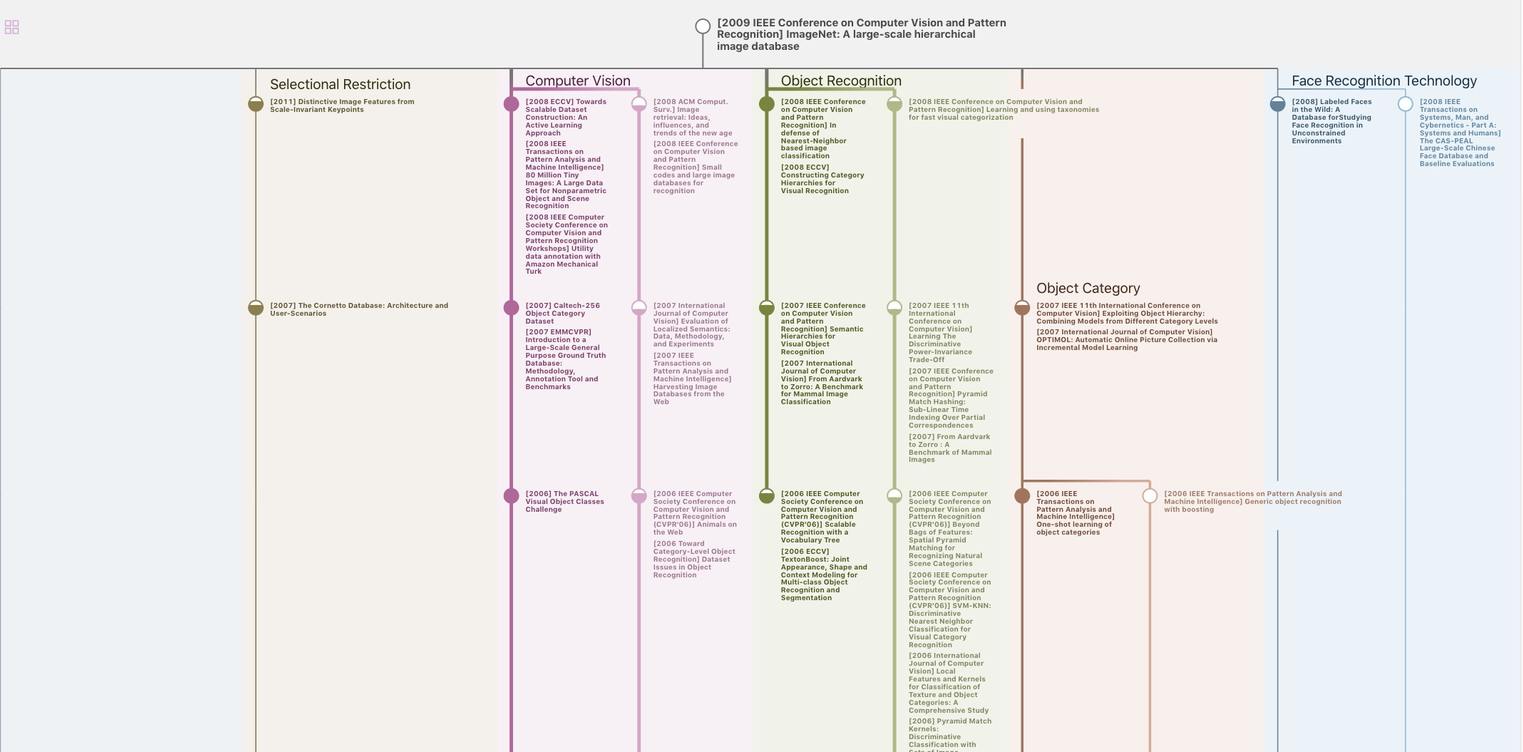
生成溯源树,研究论文发展脉络
Chat Paper
正在生成论文摘要