Mixture of Low-rank Experts for Transferable AI-Generated Image Detection
CoRR(2024)
摘要
Generative models have shown a giant leap in synthesizing photo-realistic
images with minimal expertise, sparking concerns about the authenticity of
online information. This study aims to develop a universal AI-generated image
detector capable of identifying images from diverse sources. Existing methods
struggle to generalize across unseen generative models when provided with
limited sample sources. Inspired by the zero-shot transferability of
pre-trained vision-language models, we seek to harness the nontrivial
visual-world knowledge and descriptive proficiency of CLIP-ViT to generalize
over unknown domains. This paper presents a novel parameter-efficient
fine-tuning approach, mixture of low-rank experts, to fully exploit CLIP-ViT's
potential while preserving knowledge and expanding capacity for transferable
detection. We adapt only the MLP layers of deeper ViT blocks via an integration
of shared and separate LoRAs within an MoE-based structure. Extensive
experiments on public benchmarks show that our method achieves superiority over
state-of-the-art approaches in cross-generator generalization and robustness to
perturbations. Remarkably, our best-performing ViT-L/14 variant requires
training only 0.08
mAP and +12.72
even outperforms the baseline with just 0.28
and pre-trained models will be available at
https://github.com/zhliuworks/CLIPMoLE.
更多查看译文
AI 理解论文
溯源树
样例
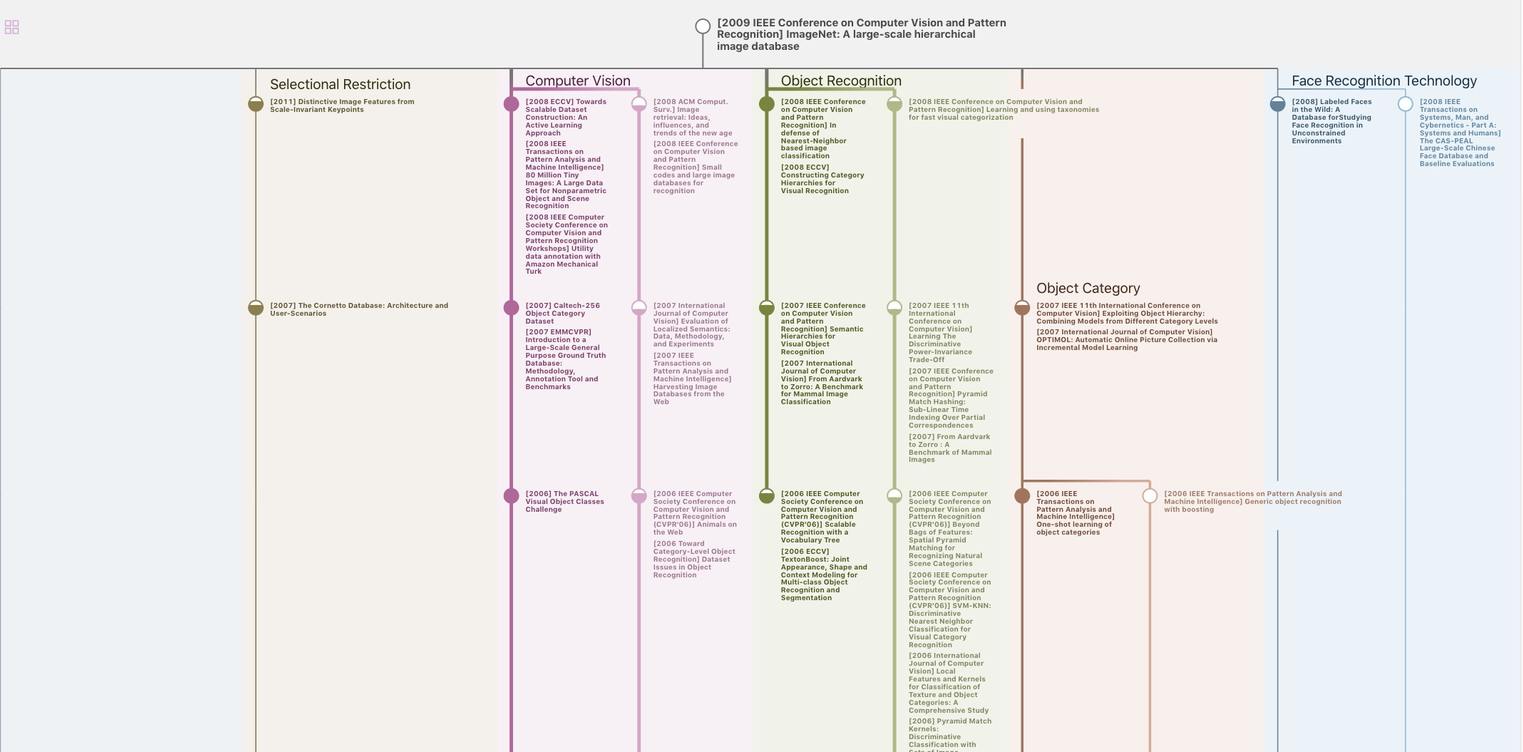
生成溯源树,研究论文发展脉络
Chat Paper
正在生成论文摘要