Hamiltonian Learning using Machine Learning Models Trained with Continuous Measurements
arxiv(2024)
摘要
We build upon recent work on using Machine Learning models to estimate
Hamiltonian parameters using continuous weak measurement of qubits as input. We
consider two settings for the training of our model: (1) supervised learning
where the weak measurement training record can be labeled with known
Hamiltonian parameters, and (2) unsupervised learning where no labels are
available. The first has the advantage of not requiring an explicit
representation of the quantum state, thus potentially scaling very favorably to
larger number of qubits. The second requires the implementation of a physical
model to map the Hamiltonian parameters to a measurement record, which we
implement using an integrator of the physical model with a recurrent neural
network to provide a model-free correction at every time step to account for
small effects not captured by the physical model. We test our construction on a
system of two qubits and demonstrate accurate prediction of multiple physical
parameters in both the supervised and unsupervised context. We demonstrate that
the model benefits from larger training sets establishing that it is in fact
"learning," and we show robustness to errors in the assumed physical model by
achieving accurate parameter estimation in the presence of unanticipated single
particle relaxation.
更多查看译文
AI 理解论文
溯源树
样例
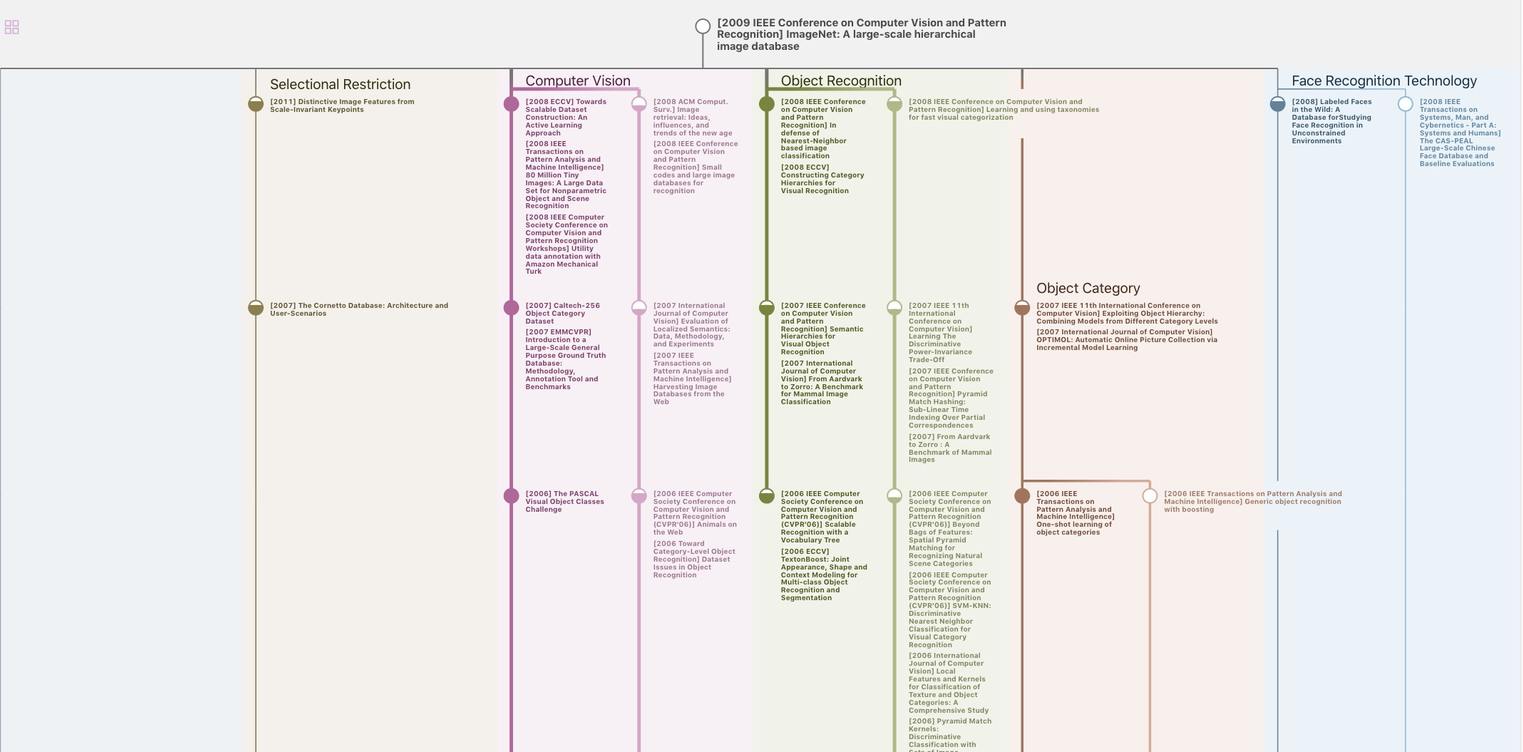
生成溯源树,研究论文发展脉络
Chat Paper
正在生成论文摘要