Exploring Machine Learning for Untargeted Metabolomics Using Molecular Fingerprints
Computer Methods and Programs in Biomedicine(2024)
摘要
Background
Metabolomics, the study of substrates and products of cellular metabolism, offers valuable insights into an organism's state under specific conditions and has the potential to revolutionise preventive healthcare and pharmaceutical research. However, analysing large metabolomics datasets remains challenging, with available methods relying on limited and incompletely annotated metabolic pathways.
Methods
This study, inspired by well-established methods in drug discovery, employs machine learning on metabolite fingerprints to explore the relationship of their structure with responses in experimental conditions beyond known pathways, shedding light on metabolic processes. It evaluates fingerprinting effectiveness in representing metabolites, addressing challenges like class imbalance, data sparsity, high dimensionality, duplicate structural encoding, and interpretable features. Feature importance analysis is then applied to reveal key chemical configurations affecting classification, identifying related metabolite groups.
Results
The approach is tested on two datasets: one on Ataxia Telangiectasia and another on endothelial cells under low oxygen. Machine learning on molecular fingerprints predicts metabolite responses effectively, and feature importance analysis aligns with known metabolic pathways, unveiling new affected metabolite groups for further study.
Conclusion
In conclusion, the presented approach leverages the strengths of drug discovery to address critical issues in metabolomics research and aims to bridge the gap between these two disciplines. This work lays the foundation for future research in this direction, possibly exploring alternative structural encodings and machine learning models.
更多查看译文
关键词
Ataxia telangiectasia,mass spectrometry,molecular fingerprinting,untargeted metabolomics,machine learning
AI 理解论文
溯源树
样例
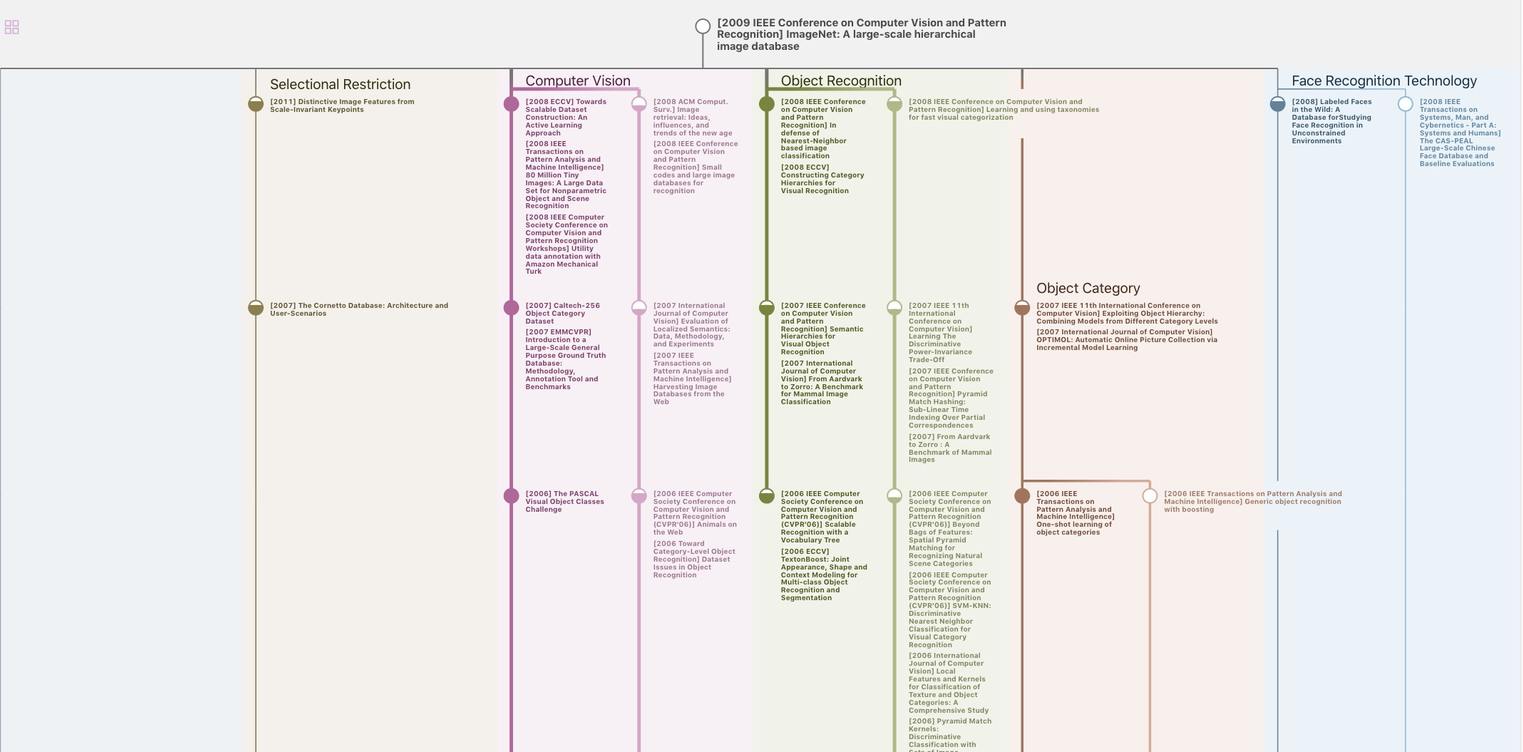
生成溯源树,研究论文发展脉络
Chat Paper
正在生成论文摘要