Mineral Prospectivity Mapping Using Knowledge Embedding and Explainable Ensemble Learning: A Case Study of the Keeryin Ore Concentration in Sichuan, China
Ore geology reviews(2024)
Abstract
GIS-based Mineral Prospectivity Mapping (MPM) has been widely employed, however, the absence of correlative interpretation between the final prospectivity maps and metallogeneny has resulted in low credibility in the prediction results. Therefore, in this study, machine learning technology combined with knowledge embedding and explainable ensemble learning is utilized to improve interpretability of the result. The Best-worst method (BWM) was employed for the embedding of prior knowledge. Stacking ensemble learning integrated these weights into the predictive model and generated targets. Permutation Importance (PI), Partial Dependence Plots (PDP), and Local Interpretable Model-agnostic Explanations (LIME), were applied to calculate the global and local output weights of features, thereby enhancing the interpretability of the targets. An experiment was carried out at the Keeryin ore concentration in Sichuan, China. The experiment demonstrates effectiveness of this method, wherein 84 % of samples falling within high and extremely high mineralization probability zones, covering 6.58 % of the total area. Na-feldspar spectrum, Na2O + K2O, ring structures, Li/La, and two-mica granite have emerged as key predictive features in sequence, which displays orderliness and verifies their tight correlations with the metallogenic environment and prospecting indicators of pegmatite-type lithium deposit in Keeryin.
MoreTranslated text
Key words
Knowledge Embedding,Stacking Ensemble Learning,Explainability,Keeryin Ore Concentration
AI Read Science
Must-Reading Tree
Example
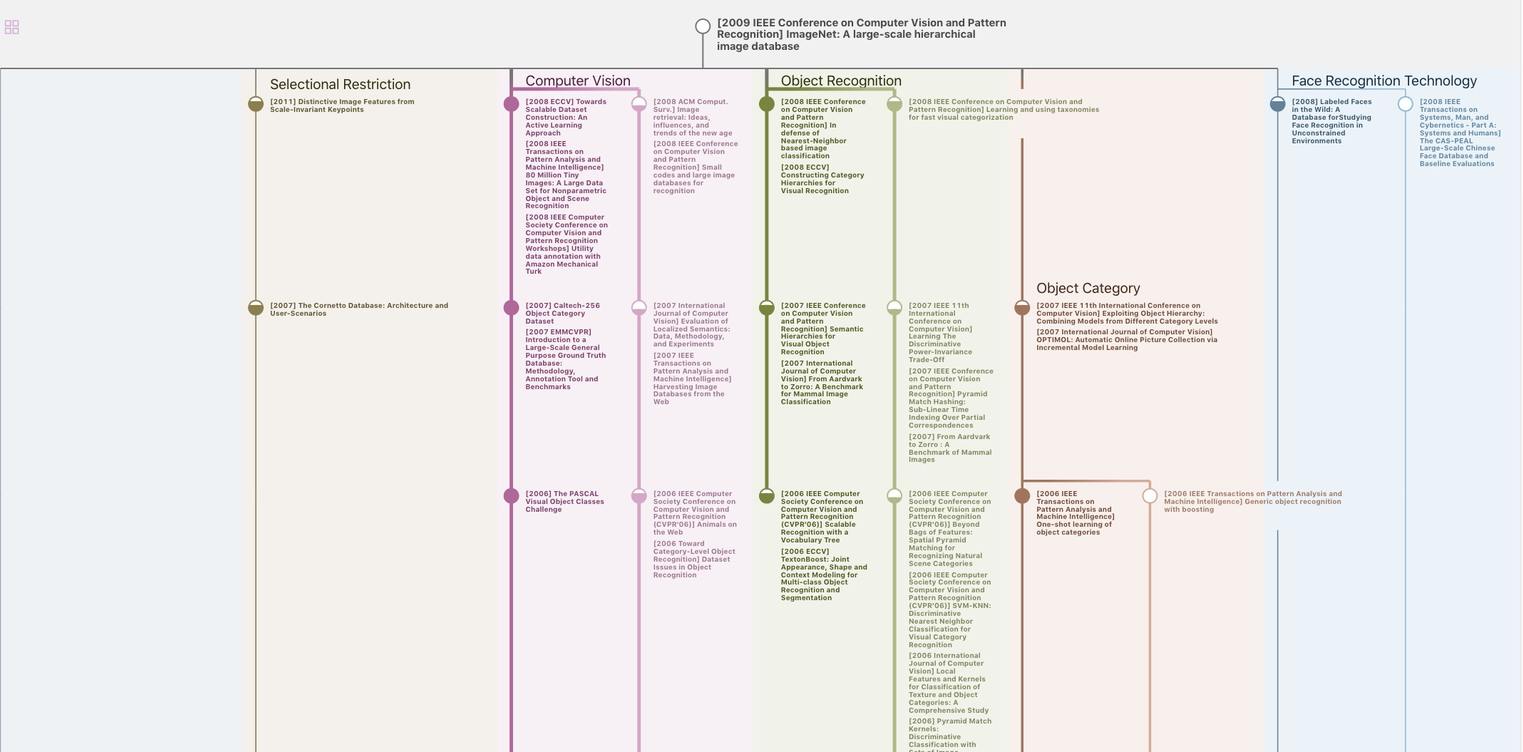
Generate MRT to find the research sequence of this paper
Chat Paper
Summary is being generated by the instructions you defined