DTNet: A Specialized Dual-Tuning Network for Infrared Vehicle Detection in Aerial Images
IEEE Transactions on Geoscience and Remote Sensing(2024)
摘要
Vehicle detection in infrared aerial images is vital for both military and civilian applications, as infrared imaging remains effective under low-light conditions and various adverse weather scenarios. However, the longer wavelengths of long-wave infrared, compared to visible light, make diffraction more noticeable, leading to low-frequency degradation of vehicle information. Thermal radiation from the environment and optical system leads to higher noise in infrared images. Additionally, atmospheric transport models for various weather conditions can degrade infrared images to different extents. These factors lead to a reduced signal-to-noise ratio, which complicates the extraction of clear features. To overcome these challenges, we propose the Dual-Tuning Network (DTNet), an advanced framework for vehicle detection in infrared aerial images, developed based on the mechanisms of infrared imaging. Specifically, the core component of DTNet is the Dual-Tuning Block (DTBlock), which works alongside the Dynamic Guided Filtering Module (DGFM) and the Point Spread Recovery Module (PSRM) for feature extraction. DTBlock decomposes feature maps into low- and high-frequency components with learnable low-pass filters. DGFM eliminates disturbance from the optical system and background thermal radiation in the high-frequency component of feature maps, while preserving the details and texture of vehicles. PSRM aggregates vehicle features in the low-frequency component of feature maps, which is proposed with reference to diffraction and atmospheric models. The concept of Dual-Tuning refers to enhancing the signal and suppressing interference in the low and high frequency parts, respectively. Experimental results on the DroneVehicle public dataset for infrared vehicle detection indicate that our proposed approach achieves state-of-the-art (SOTA) performance. Moreover, extensive ablation studies confirm the superior capability of our DTNet in robust feature extraction from infrared images.
更多查看译文
关键词
Infrared aerial image,vehicle detection,image degradation,feature extraction,frequency decomposition
AI 理解论文
溯源树
样例
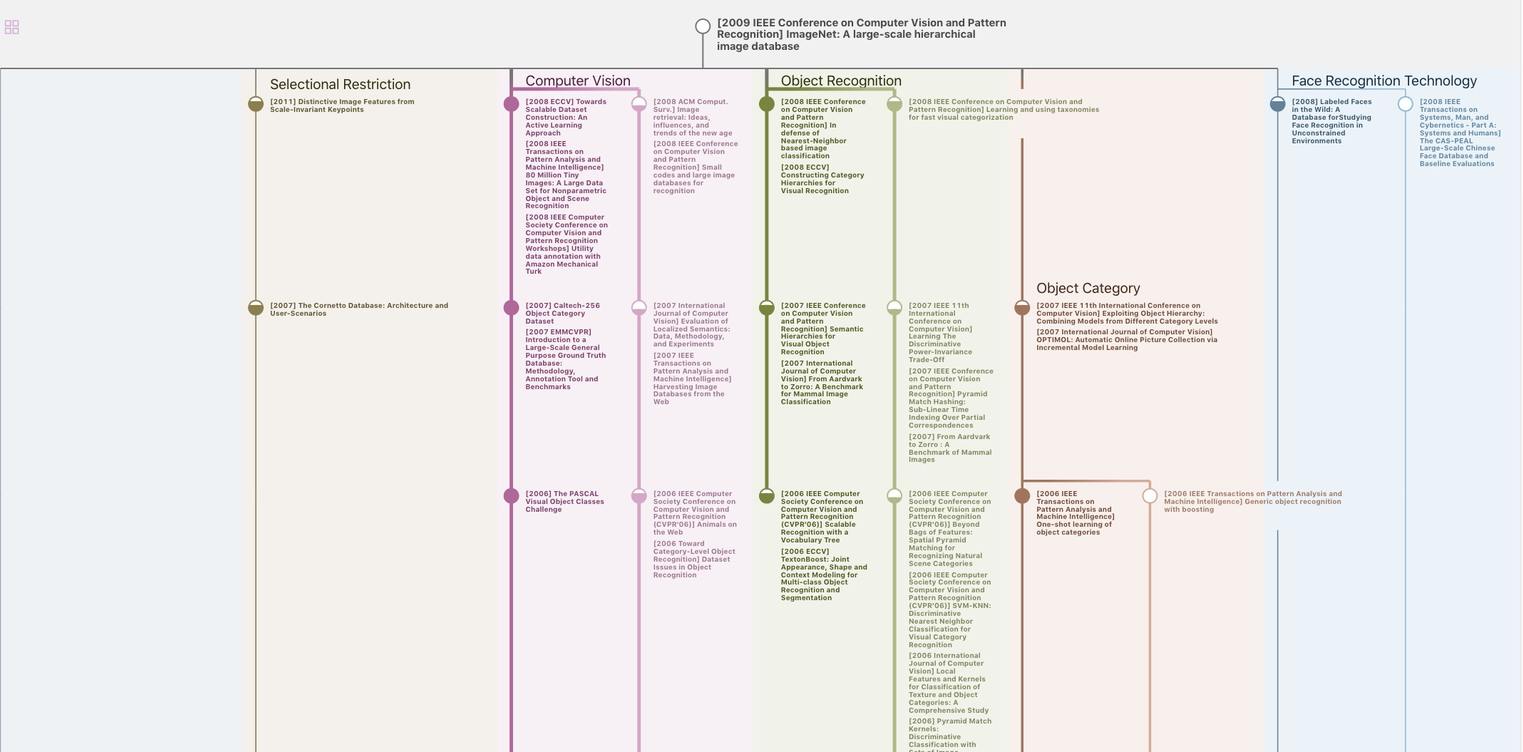
生成溯源树,研究论文发展脉络
Chat Paper
正在生成论文摘要