Positional Feature Generator-Based Transformer for Image Captioning
2023 18th International Conference on Intelligent Systems and Knowledge Engineering (ISKE)(2023)
摘要
The Transformer-based architecture achieves state-of-the-art results in image captioning. Due to its non-recurrent nature, additional positional information needs to be provided. However, existing advanced methods attach positional information to the model by additional encoding or embedding, which is independently decoupled from the original input features. In addition, no matter absolute or relative methods, the encodings are fused with input features by add operation, which leads to information interference between the two types of features and affects the performance of the model. In this paper, we propose a novel architecture to remedy the above limitations, called positional feature generator (PFG). This module is effective in modeling image spatial positional frame by graph structure, which can learn absolute position explicitly and relative position implicitly. Meanwhile, we concatenate the captured positional features with the original features, making the positional information as a separate additional feature to avoid feature interference. Extensive experiments on MS COCO validate the effectiveness of PFG. Moreover, PFG outperforms some state-of-the-art positional representation methods, and positional feature generator-based Transformer (PFGT) is competitive with some state-of-the-art image captioning algorithms.
更多查看译文
关键词
Image Captioning,Transformer,Positional Encoding
AI 理解论文
溯源树
样例
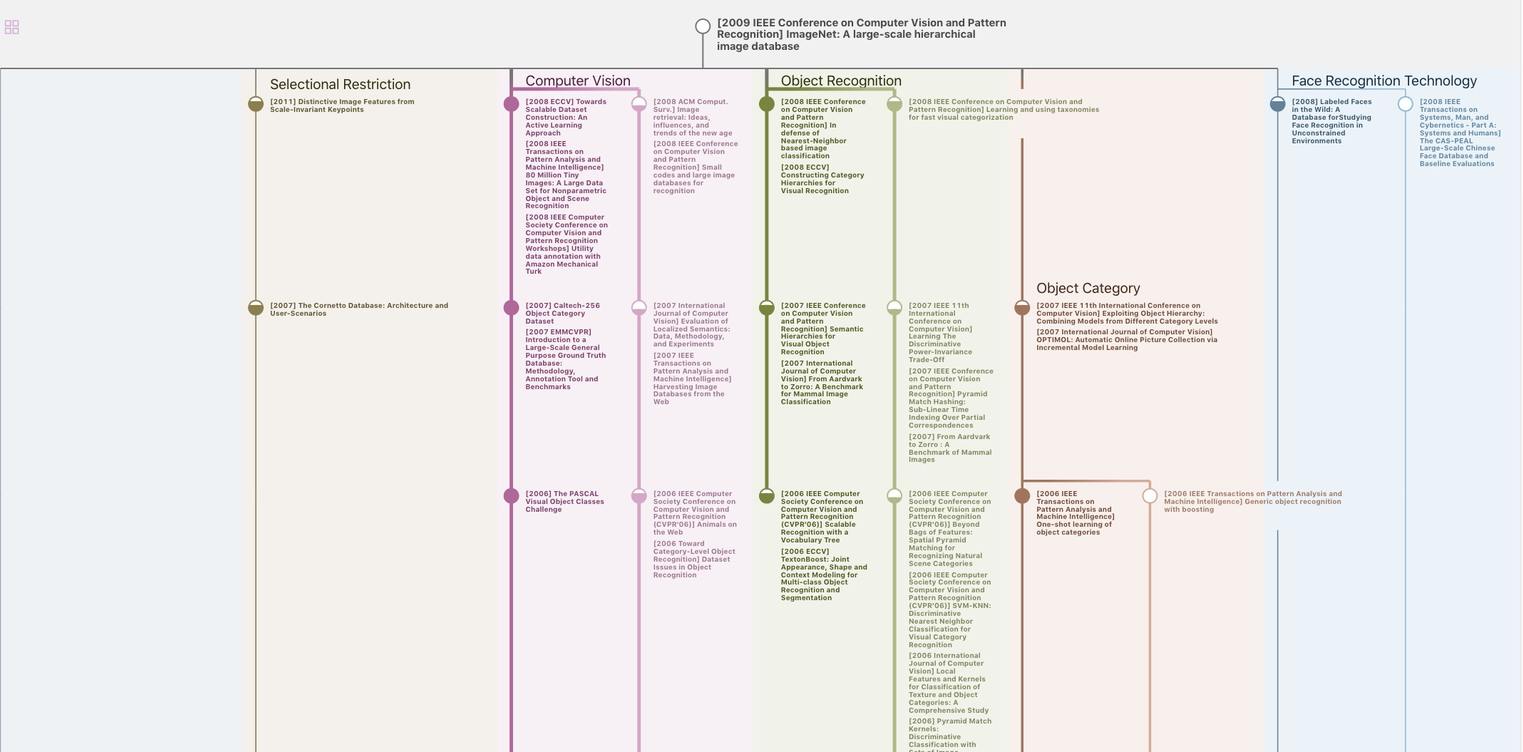
生成溯源树,研究论文发展脉络
Chat Paper
正在生成论文摘要