The Enhancement of Unsupervised Cross-Modality Domain Adaptive Cardiac Segmentation: Self-Training++ and FC-CRF Optimization
crossref(2024)
摘要
Abstract The training of deep convolutional neural networks requires a substantial amount of labeled data. Nevertheless, the available kinds of labels that are implemented currently to segment medical images cause high-quality image annotations that bring costly and time-consuming procedures. Moreover, distinct modalities such as CT and MRI-based cardiac images make it difficult to train a model. So, one set of image data in one modality cannot be segmented into image data in another modality efficiently. Thus, an unsupervised domain-adaptive cardiac segmentation algorithm implementing a Self-training + + algorithm is suggested to conduct a process called the cross-modality medical-image segmentation known as modality data. The algorithm produces pseudo-labels for images utilized in the target domain and introduces rare class sampling (RCS) by implementing the Self-training + + approach. Additionally, the network called the Mix Transformer semantic segmentation is enhanced by incorporating the module called the atrous spatial pyramid pooling to acquire multiscale semantic information as if it were a teacher and student network to advance the robustness of the segmentation network. Then, fully connected conditional random fields (FC-CRF) are implemented to raise the confidence level of pseudo-labeling. The proposed algorithm substantially decreases the domain bias impact on the outcomes of cardiac image segmentation when distinct modalities exist and alleviates the dependence on expert-labeled data. Then, domain-adaptive training for full cardiac medical-image segmentation is performed in two directions, namely, CT to MRI and MRI to CT cardiac images, and promising experimental outcomes are achieved even though a limited number of data is employed.
更多查看译文
AI 理解论文
溯源树
样例
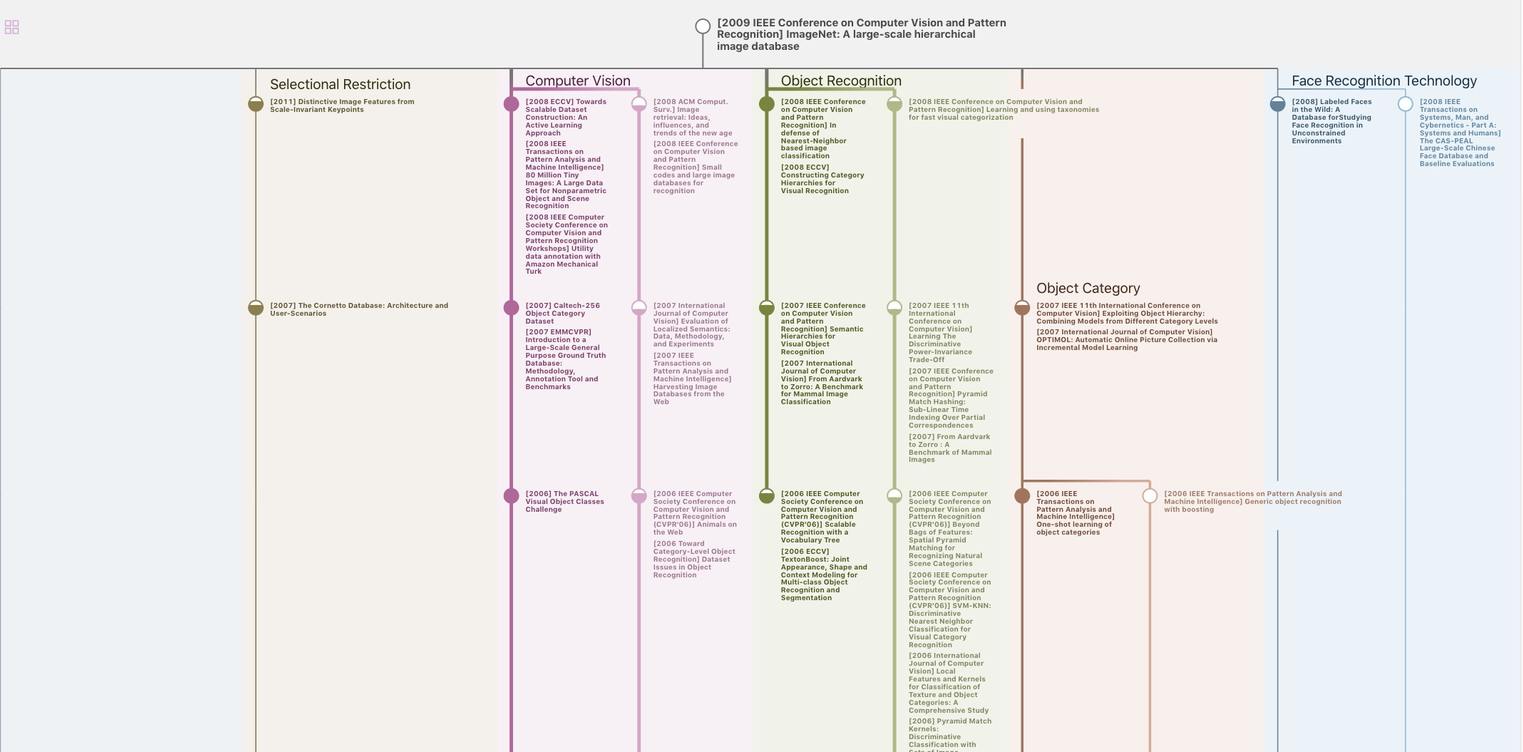
生成溯源树,研究论文发展脉络
Chat Paper
正在生成论文摘要