Joint Estimation of SOC and SOH for Lithium-ion Battery Based on Multi-Time Scale
2024 IEEE 3rd International Conference on Electrical Engineering, Big Data and Algorithms (EEBDA)(2024)
摘要
The accurate estimation of the State of Charge (SOC) and State of Health (SOH) of a lithium-ion battery is crucial to ensuring the safe and stable operation of the battery, and is the key technology of the management system of lithium-ion battery. Aiming at the problem that the model parameters and capacity of the battery will change with the increase of the number of cycles, thus affecting the estimation accuracy of SOC. This paper proposes a method of joint estimation of SOC and SOH for lithium-ion batteries based on multi-time scale. At the macro scale, Health Factor (HF) was extracted from the fragment information of the charging curve, and a battery aging model was established by the Support Vector Machine (SVM) to estimate SOH parameters. According to the estimation results of SOH, the model parameters and capacity parameters of the battery used for SOC estimation were updated. At the microscopic scale, the autoregressive exogenous (ARX) model with online parameter identification was used, and the Unscented Kalman Filter (UKF) algorithm was used for real-time estimation of battery SOC, so as to achieve joint state estimation of lithium-ion battery. Experimental results show that the proposed method improves the long-term estimation performance of battery SOC with high accuracy and reliability.
更多查看译文
关键词
Multi-time scale,support vector machine,autoregressive exogenous model,state of charge,state of health
AI 理解论文
溯源树
样例
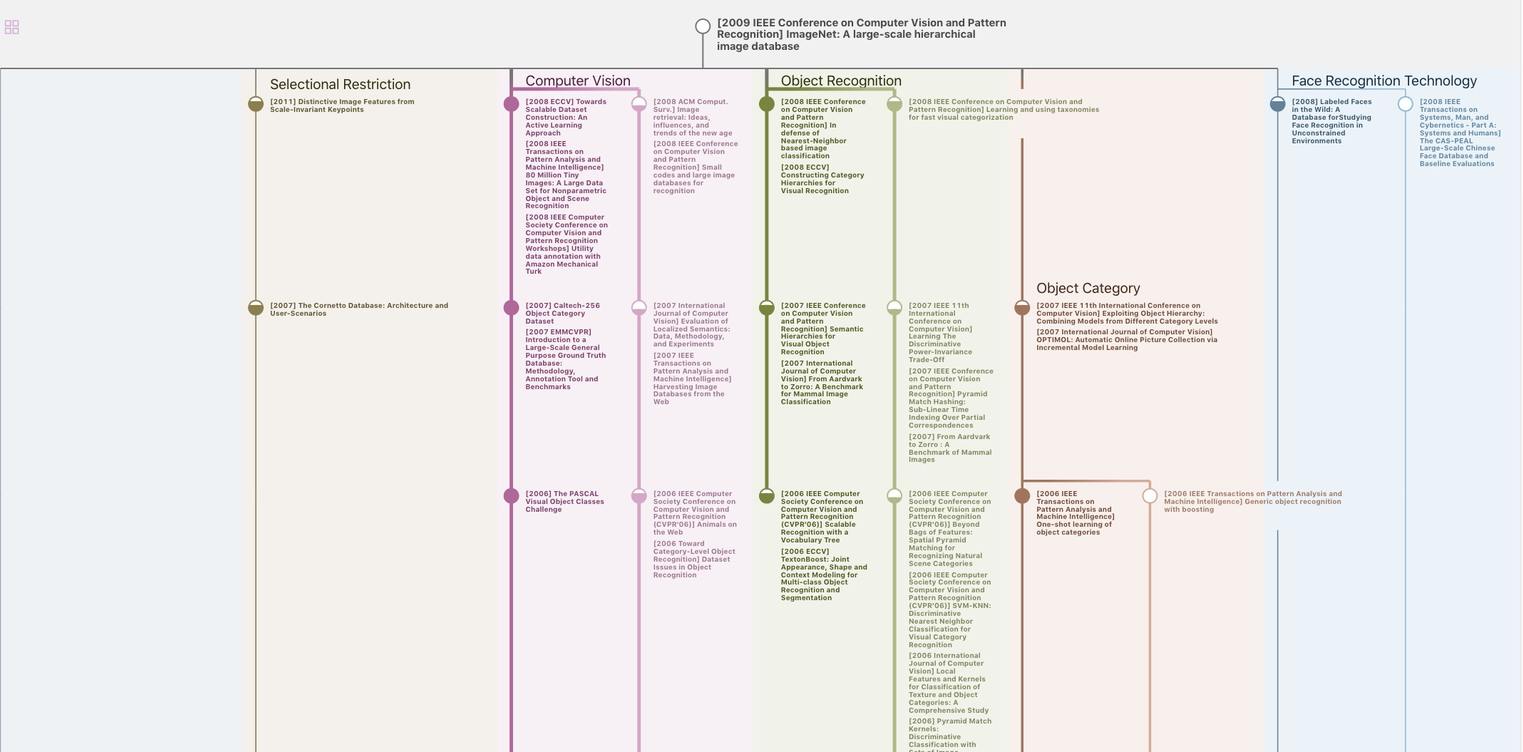
生成溯源树,研究论文发展脉络
Chat Paper
正在生成论文摘要