The Guiding Function of Different SAR Image Quality Indicators on Object Detection Tasks
2024 IEEE 3rd International Conference on Electrical Engineering, Big Data and Algorithms (EEBDA)(2024)
摘要
In the big data era, Synthetic Aperture Radar (SAR) imagery has become increasingly critical, particularly for data-driven object detection tasks. Various downstream tasks are extremely sensitive to SAR image quality because the SAR image is extremely influenced by diverse factors such as speckle noise. Therefore, the quality assessment of SAR images is crucial, especially in the context of no-reference image quality metrics. However, there is no universal standard to quantitatively assess the SAR image quality, and how these metrics influence the SAR detection tasks is ambiguous. This paper emphasizes the guiding function of different SAR image quality assessment metrics on SAR target detection tasks. A comprehensive comparison of several widely used SAR image quality metrics is undertaken, including entropy, RMS contrast, equivalent number of looks (ENL), Laplacian and Tenengrad sharpness, and frequency domain sharpness. First, utilizing the MSAR large-scale dataset, we analyze these metrics to identify the most suitable ones for object detection tasks. Further, we demonstrate the practical application of these metrics by integrating them into the YOLOv5 object detection framework. This research not only offers a new perspective on SAR image quality assessment but also provides practical guidance for future applications, aligning with the broader need for efficient and accurate no-reference quality assessment methods in areas like remote sensing, where image distortions and the unavailability of reference images are common challenges.
更多查看译文
关键词
Synthetic Aperture Radar (SAR),YOLOv5,SAR image quality metrics,object detection
AI 理解论文
溯源树
样例
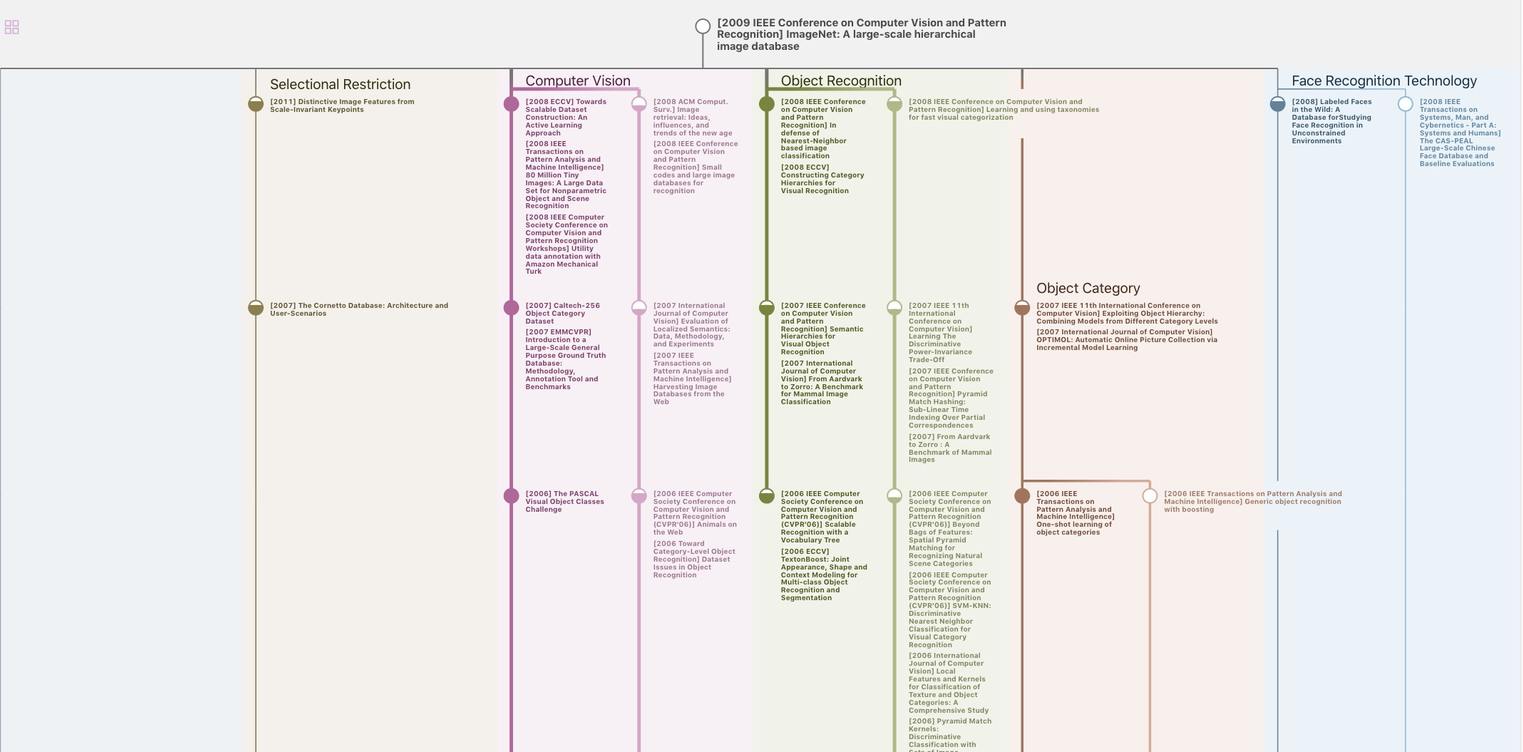
生成溯源树,研究论文发展脉络
Chat Paper
正在生成论文摘要