A Multi-View Graph Contrastive Learning Framework for Defending Against Adversarial Attacks
IEEE TRANSACTIONS ON EMERGING TOPICS IN COMPUTATIONAL INTELLIGENCE(2024)
摘要
Graph neural networks are easily deceived by adversarial attacks that intentionally modify the graph structure. Particularly, homophilous edges connecting similar nodes can be maliciously deleted when adversarial edges are inserted into the graph. Graph structure learning (GSL) reconstructs an optimal graph structure and corresponding representation and has recently received considerable attention in adversarial attacks. However, constrained by a single topology view of the poisoned graph and few labels, most GSL techniques are difficult to effectively learn robust representations that sufficiently carry precise structure information and similar node information. Therefore, this paper develops a robust multi-view graph contrastive learning (RM-GCL) framework to defend against adversarial attacks. It exploits additional structural information and contrastive supervision signals from the data to guide graph structure optimization. In particular, an adaptive graph-augmented contrastive learning (AGCL) module is devised to obtain reliable representations. Besides, a node-level attention mechanism is incorporated to fuse these representations adaptively acquired from AGCL and then complete node classification tasks. Experiments on multiple datasets manifest that RM-GCL exceeds the state-of-the-art approaches and successfully defends against various attacks.
更多查看译文
关键词
Adversarial defence,graph neural network,graph structure learning,robustness
AI 理解论文
溯源树
样例
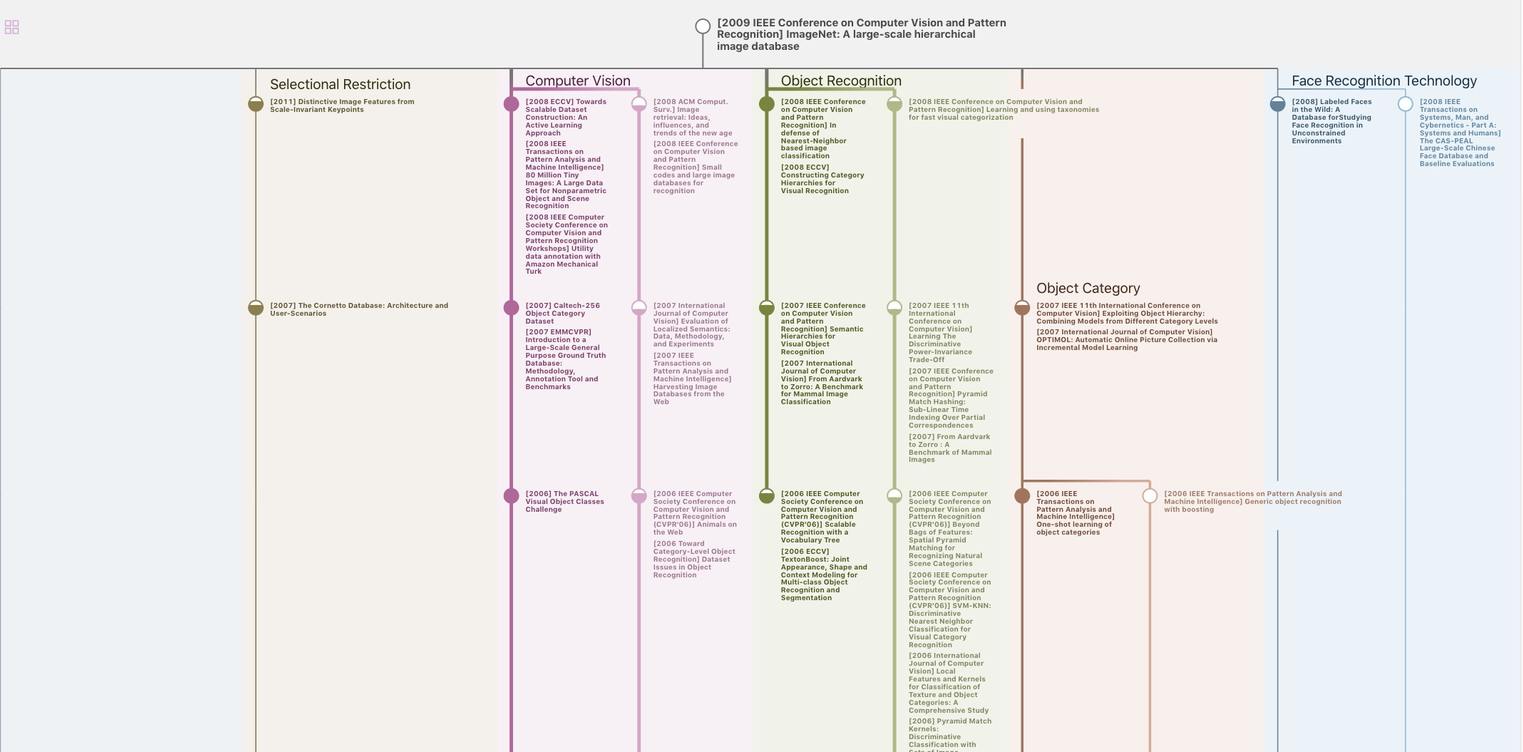
生成溯源树,研究论文发展脉络
Chat Paper
正在生成论文摘要