Multitask Augmented Random Search in deep reinforcement learning
Applied Soft Computing(2024)
摘要
Reinforcement Learning (RL) has gained significant popularity in recent years for its ability to solve complex control problems. However, most existing RL algorithms are designed to train policies for each environment in isolation, limiting their applicability to real-world scenarios with many related environments. Recently, many multitask optimization algorithms have been proposed and successfully applied to a wide range of optimization problems. However, existing studies mainly focus on solving multiple continuous function benchmarks and overlook the potential of tailoring towards RL. In this paper, we propose a simple multitask optimization algorithm called Multitask Augmented Random Search (MARS) that trains multiple RL agents together and exploits the performance surplus from highly correlated tasks. MARS is a modification of the simple random search Augmented Random Search (ARS) algorithm, which has been shown to outperform complicated methods in solving continuous control MuJoCo environments such as Soft Actor-Critic (SAC), Proximal Policy Optimization (PPO), and Trusted Region Policy Optimization (TRPO). The experimental results also demonstrate that our proposed algorithm is more consistent in solving different instances of MuJoCo benchmark than ARS, Multifactorial Evolution Algorithm (MFEA), and Adaptive MFEA RL (AMFEARL) within the same number of training episodes.
更多查看译文
关键词
Multifactorial evolutionary algorithm,Deep reinforcement learning,Augmented random search
AI 理解论文
溯源树
样例
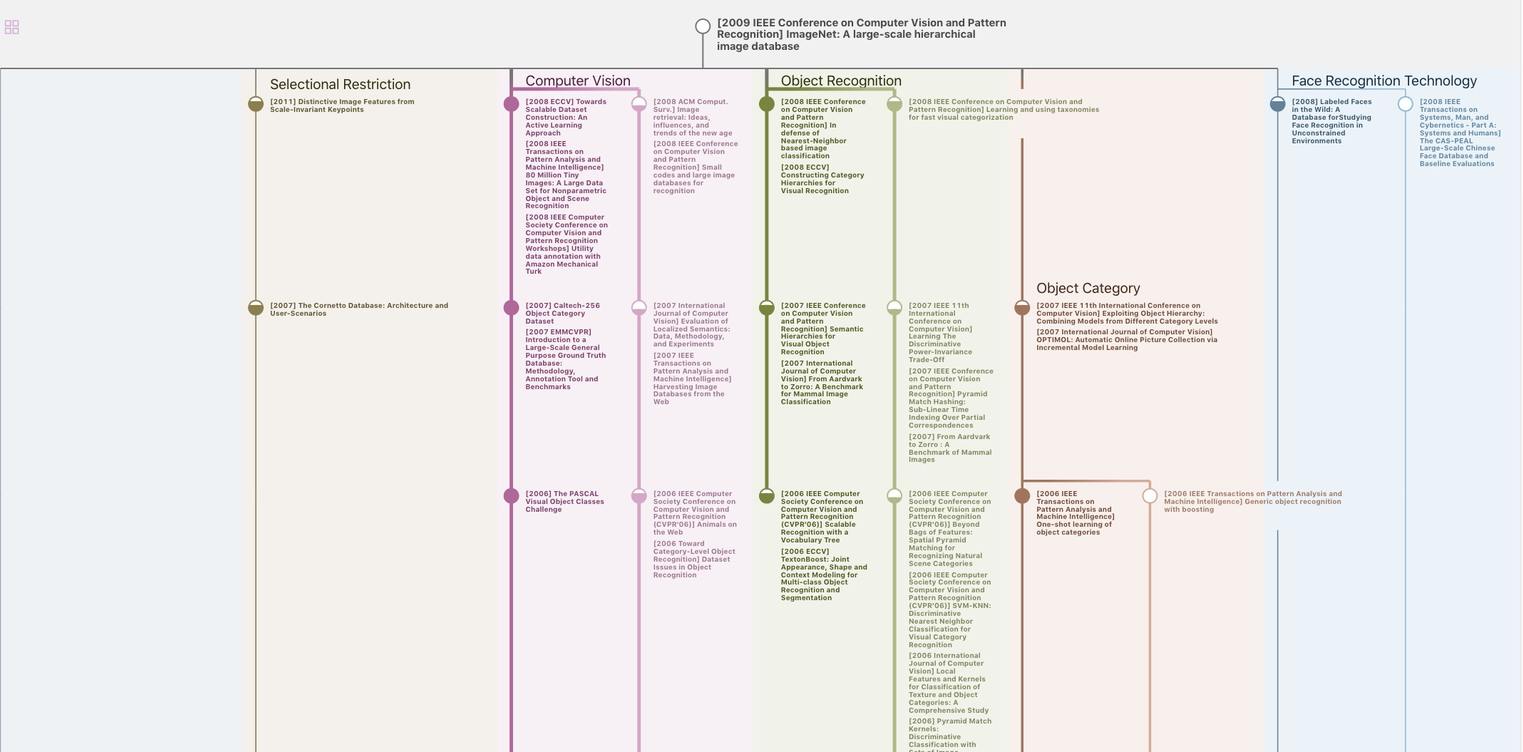
生成溯源树,研究论文发展脉络
Chat Paper
正在生成论文摘要