Collaborative knowledge amalgamation: Preserving discriminability and transferability in unsupervised learning
Information Sciences(2024)
摘要
Unsupervised Knowledge Amalgamation (UKA) trains a versatile student model with an unlabeled dataset to handle joint objectives of multiple off-the-shelf teacher models, each specialized for distinct tasks. Existing research primarily focuses on facilitating the learning of discriminative knowledge by student models from their teacher models within a latent feature space, but often overlooks the transferability of such knowledge to downstream tasks. In this paper, we propose a novel Collaborative Knowledge Amalgamation (Co-KA) framework, which aims to preserve both the discriminability and transferability of knowledge in the UKA task. Specifically, within the latent feature space, we introduce the concept of “inherent semantic relationships” between mini-batch samples to maintain knowledge transferability through a distance-based contrastive objective. Additionally, we employ alignment loss to ensure knowledge discrimination between the target student and teachers. In the logit space, we design a decoupled soft-target distillation to enable the student model to acquire both discriminative and transferable knowledge from the aggregate teachers. Extensive experiments on various benchmarks and challenging cross-dataset tasks empirically demonstrate the efficacy and adaptability of our approach for unsupervised knowledge amalgamation.
更多查看译文
关键词
Knowledge Amalgamation,Transferability,Discriminability,Unsupervised Learning,Contrastive Learning
AI 理解论文
溯源树
样例
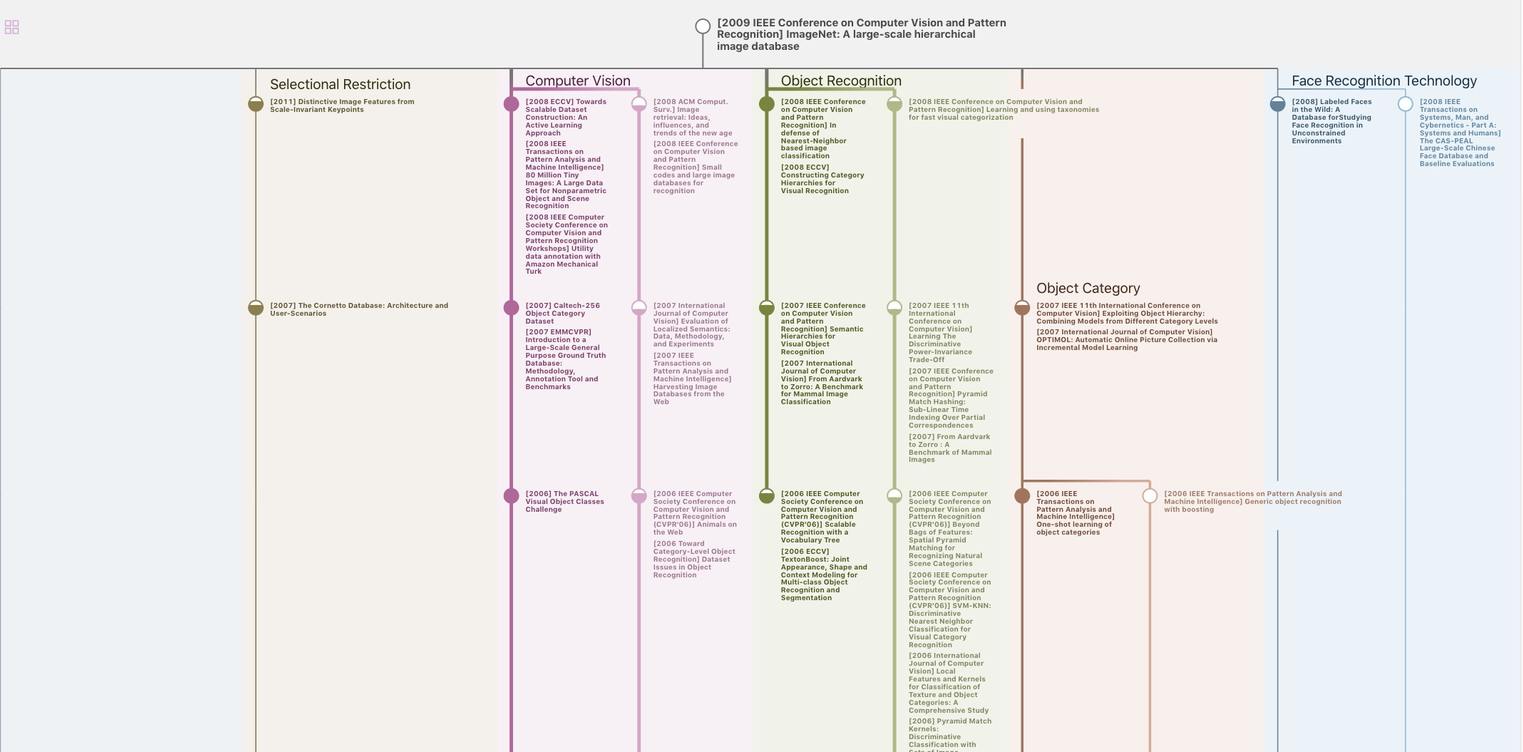
生成溯源树,研究论文发展脉络
Chat Paper
正在生成论文摘要