Improving the estimation accuracy of confined vapor-liquid equilibria by fine-tuning the pure component parameter in the PC-SAFT equation of state
PHYSICAL CHEMISTRY CHEMICAL PHYSICS(2024)
摘要
We propose a thermodynamic model that combines the Young-Laplace equation and perturbed chain-statistical associating fluid theory (PC-SAFT) equation of state to estimate capillary condensation pressure in microporous and mesoporous sorbents. We adjust the PC-SAFT dispersion-energy parameter when the pore size becomes comparable to the molecular dimension. This modelling framework is applied to diverse systems containing associating and non-associating gases, various sorbents, and a wide range of temperatures. Our simulation results show that under extreme confinement, a higher value of the dispersion-energy parameter (epsilon) is required. Furthermore, using the experimental saturation pressure data for 18 different associating and non-associating confined fluids, we find that the shift in the PC-SAFT dispersion energy correlates with the ratio of the sorbent mean pore size to the PC-SAFT segment size (r(p)/sigma). By fitting to the capillary condensation data, the relative deviation between the confined and bulk PC-SAFT dispersion energy parameter is only 0.1% at r(p)/sigma = 15; however, this deviation starts to increase exponentially as r(p)/sigma decreases. For a sorbent with large pores, when r(p)/sigma > 15, the capillary condensation pressure results from our model are similar to the predictions from the Kelvin equation. Using a dataset containing 235 saturation pressure data points composed of 18 pure gases and 4 binary mixtures, the overall AARD% from our model is 12.26%, which verifies the good accuracy of our model. Because the mean sorbent pore radius (r(p)), the PC-SAFT energy parameter (epsilon), and segment size (sigma) are known a priori, our model estimates the corrected energy parameter for small pores and, thus, extends its applicability.
更多查看译文
AI 理解论文
溯源树
样例
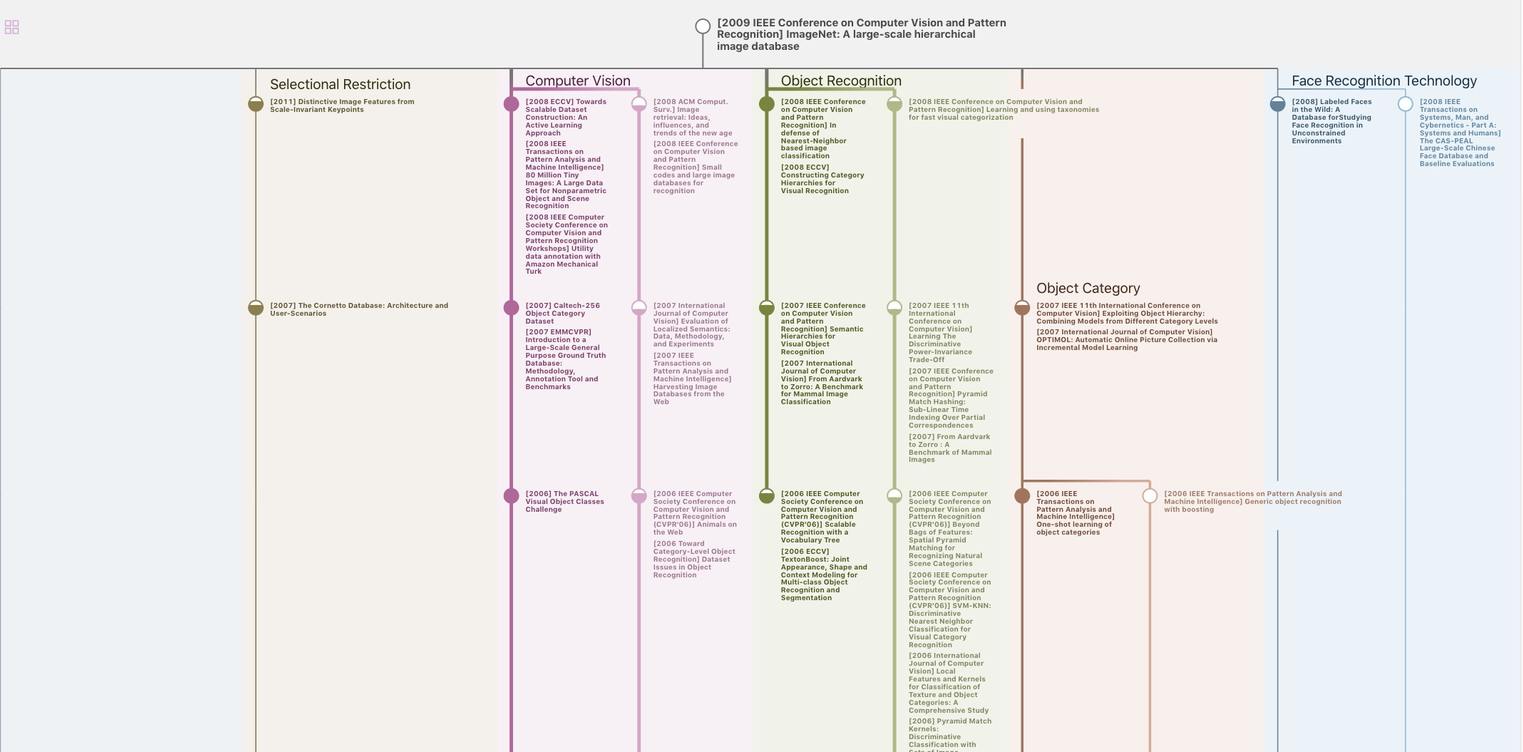
生成溯源树,研究论文发展脉络
Chat Paper
正在生成论文摘要