Indoor localization and trajectory correction with point cloud-derived backbone map
International Journal of Applied Earth Observation and Geoinformation(2024)
摘要
As a commonly used indoor internet-of-things (IoT) positioning method, fingerprinting is frequently carried out through the fusion of inertial and WiFi or magnetic data. Nevertheless, signals like magnetic intensity and WiFi received signal strength tend to fluctuate and cannot always provide high accuracy. Thus, other available data can be introduced to maintain positioning continuity and reliability. Among available data sources, 3D point clouds can be used to provide high-accuracy geospatial information. However, applying it directly in positioning leads to considerable memory, computational consumption, and restriction in use patterns. To address this challenge, this paper proposed a lightweight localization method that uses 3D point clouds to reliably correct the dispersed user trajectory to assist indoor IoT localization. Within this approach, the point cloud is transformed into a screened backbone map, diminishing data volume and computational demands. Subsequently, considering the fact that mass-market users do not always follow the route-screened backbone map, a screened backbone map-based user trajectory correction algorithm is presented to enhance localization accuracy and reliability. Within a 100 m * 60 m indoor parking lot, the proposed approaches reduced the memory from over 1 GB in point clouds to 500 kB in screened backbone map, provided 100 % correct matching between user trajectory and generated screened backbone map, and improved magnetic positioning accuracy by over 80 % when aiding with the generated route-screened backbone map.
更多查看译文
关键词
Positioning,Trajectory correction,Backbone map,Trajectory similarity judgment
AI 理解论文
溯源树
样例
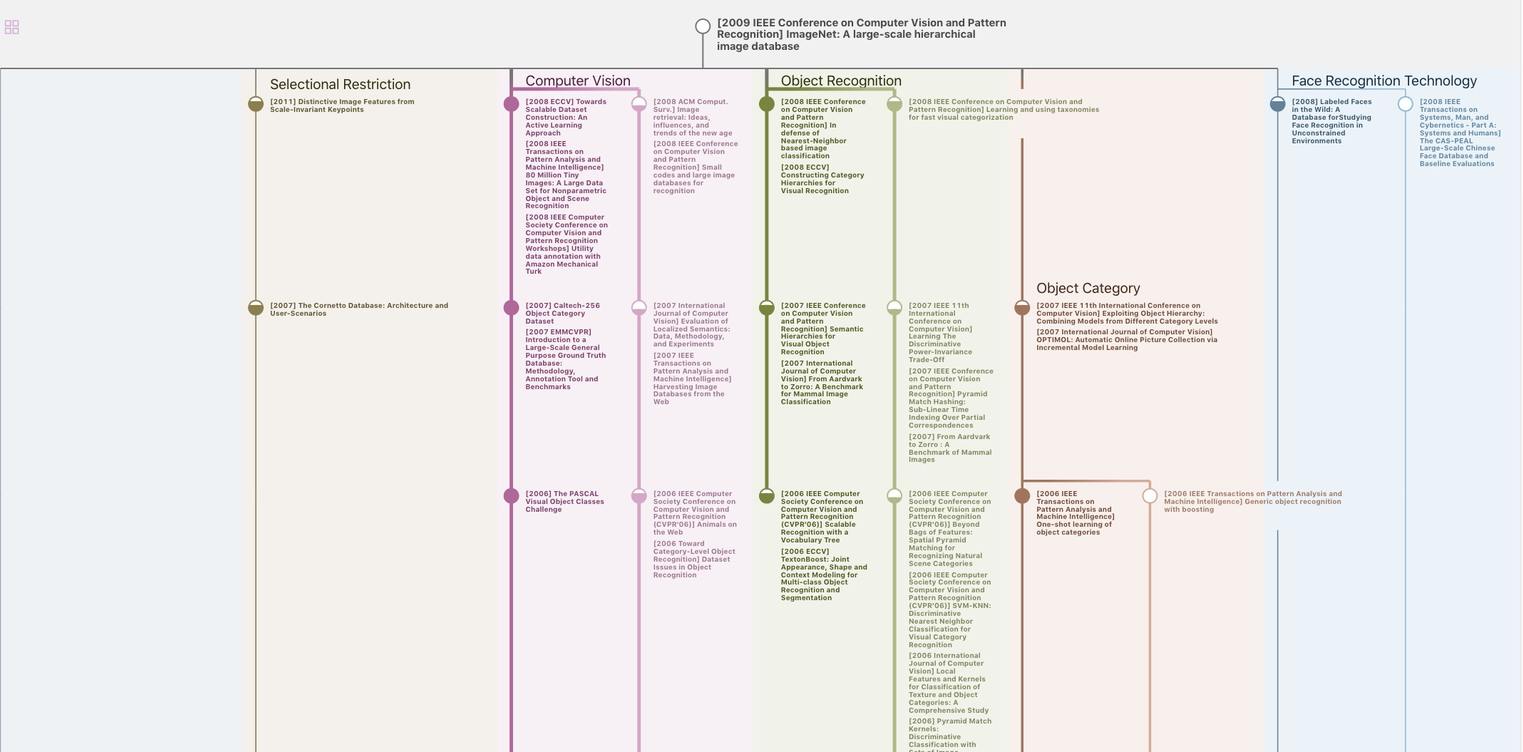
生成溯源树,研究论文发展脉络
Chat Paper
正在生成论文摘要