UFNet: Joint U-Net and fully connected neural network to bias correct precipitation predictions from climate models
Artificial Intelligence for the Earth Systems(2024)
摘要
Abstract Precipitation values produced by climate models are biased due to the parameterization of physical processes and limited spatial resolution. Current bias correction approaches usually focus on correcting lower-order statistics (mean, standard deviation), which make it difficult to capture precipitation extremes. However, accurate modeling of extremes is critical for policymaking to mitigate and adapt to the effects of climate change. We develop a deep learning framework, leveraging information from key dynamical variables impacting precipitation to also match higher-order statistics (skewness and kurtosis) for the entire precipitation distribution, including extremes. The deep learning framework consists of a two-part architecture: a U-Net convolutional network to capture the spatiotemporal distribution of precipitation and a fully connected network to capture the distribution of higher-order statistics. The joint network, termed UFNet, can simultaneously improve the spatial structure of the modeled precipitation and capture the distribution of extreme precipitation values. Using climate model simulation data and observations that are climatologically similar but not strictly paired, the UFNet identifies and corrects the climate model biases, significantly improving the estimation of daily precipitation as measured by a broad range of spatiotemporal statistics. In particular, UFNet significantly improves the underestimation of extreme precipitation values seen with current bias-correction methods. Our approach constitutes a generalized framework for correcting other climate model variables which improves the accuracy of the climate model predictions, while utilizing a simpler and more stable training process.
更多查看译文
AI 理解论文
溯源树
样例
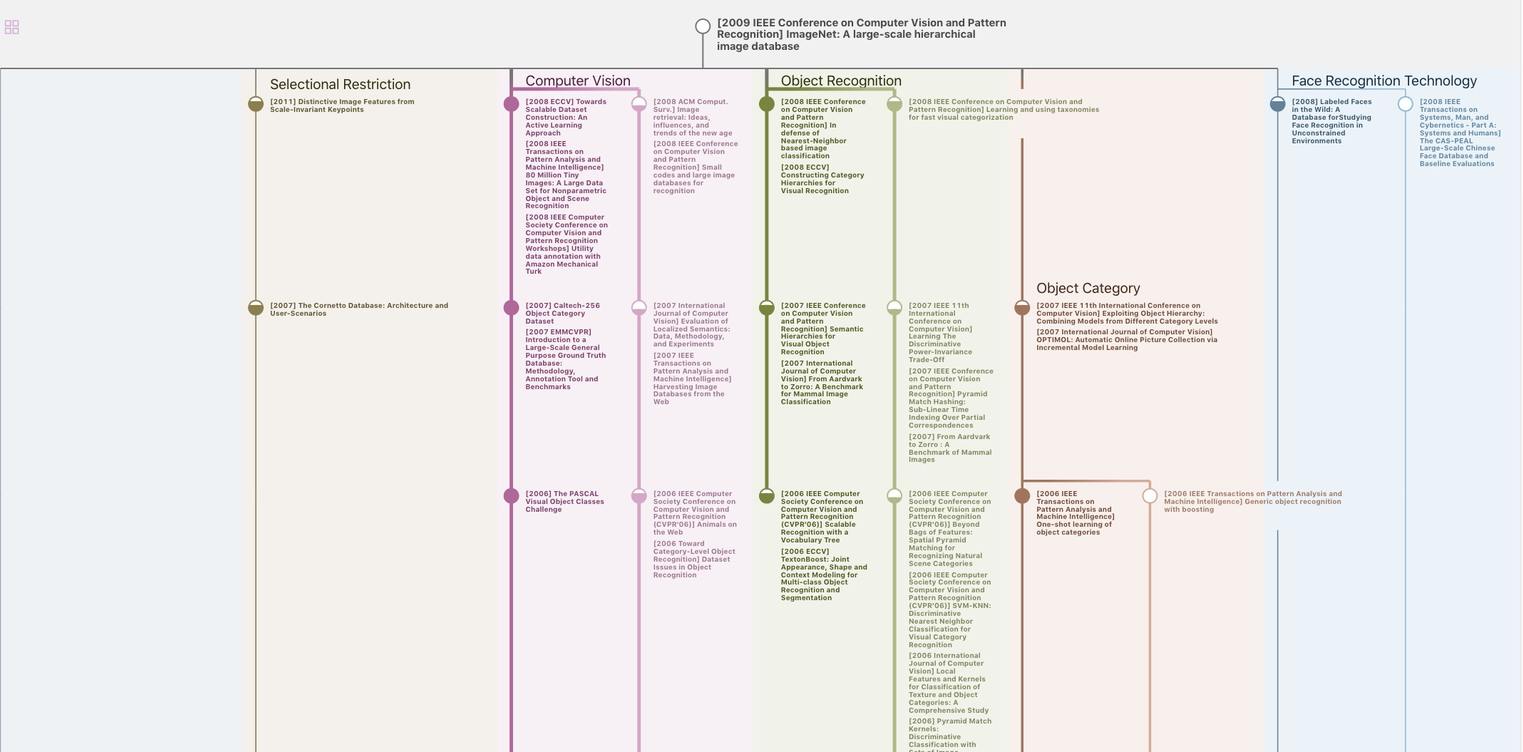
生成溯源树,研究论文发展脉络
Chat Paper
正在生成论文摘要