Assessing and Improving the Robustness of Bayesian Evidential Learning in One Dimension for Inverting Time-Domain Electromagnetic Data: Introducing a New Threshold Procedure
WATER(2024)
摘要
Understanding the subsurface is of prime importance for many geological and hydrogeological applications. Geophysical methods offer an economical alternative for investigating the subsurface compared to costly borehole investigations. However, geophysical results are commonly obtained through deterministic inversion of data whose solution is non-unique. Alternatively, stochastic inversions investigate the full uncertainty range of the obtained models, yet are computationally more expensive. In this research, we investigate the robustness of the recently introduced Bayesian evidential learning in one dimension (BEL1D) for the stochastic inversion of time-domain electromagnetic data (TDEM). First, we analyse the impact of the accuracy of the numerical forward solver on the posterior distribution, and derive a compromise between accuracy and computational time. We also introduce a threshold-rejection method based on the data misfit after the first iteration, circumventing the need for further BEL1D iterations. Moreover, we analyse the impact of the prior-model space on the results. We apply the new BEL1D with a threshold approach on field data collected in the Luy River catchment (Vietnam) to delineate saltwater intrusions. Our results show that the proper selection of time and space discretization is essential for limiting the computational cost while maintaining the accuracy of the posterior estimation. The selection of the prior distribution has a direct impact on fitting the observed data and is crucial for a realistic uncertainty quantification. The application of BEL1D for stochastic TDEM inversion is an efficient approach, as it allows us to estimate the uncertainty at a limited cost.
更多查看译文
关键词
uncertainty,saltwater intrusion,TDEM,BEL1D,SimPEG
AI 理解论文
溯源树
样例
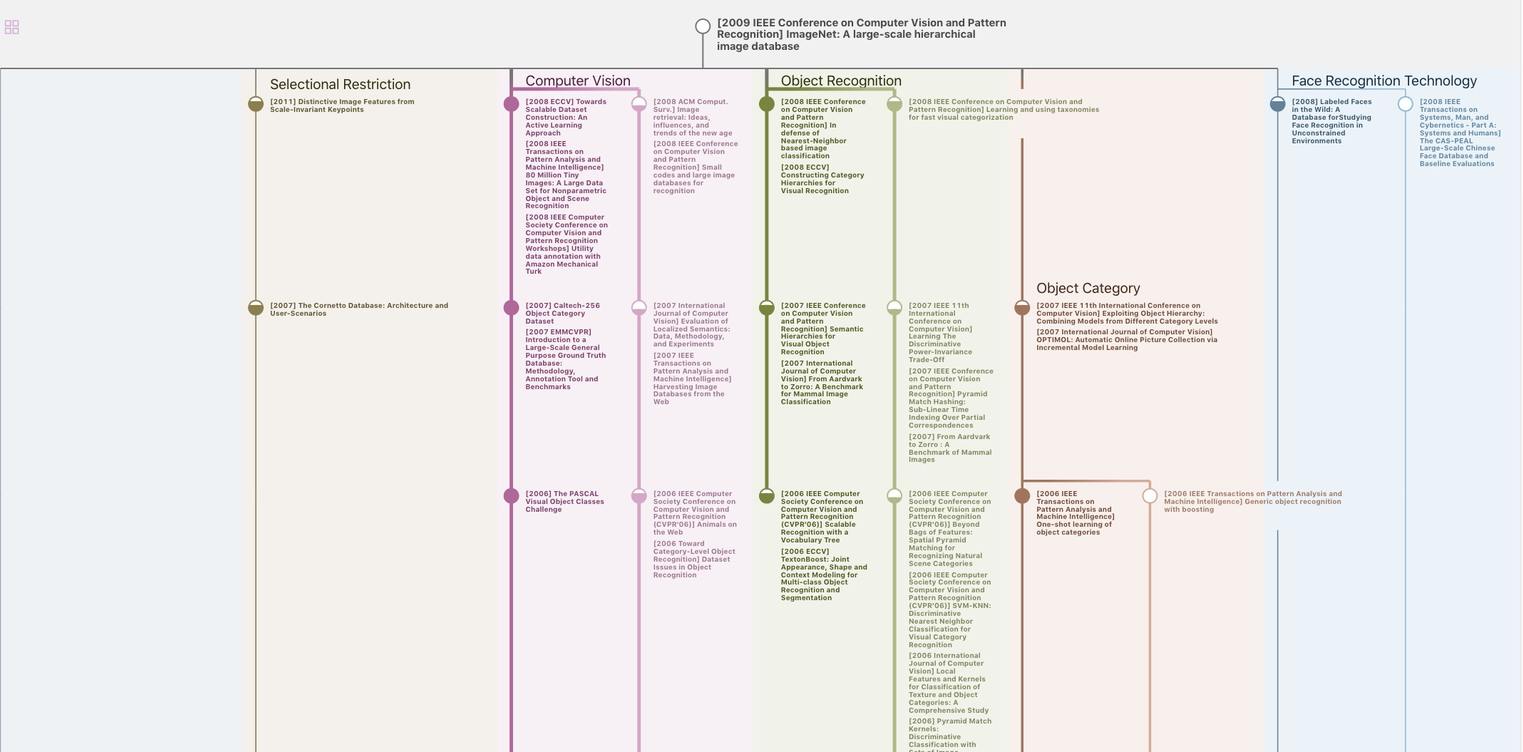
生成溯源树,研究论文发展脉络
Chat Paper
正在生成论文摘要