Extracting Structured Data from Organic Synthesis Procedures Using a Fine-Tuned Large Language Model
crossref(2024)
摘要
The popularity of data-driven approaches and machine learning (ML) techniques in the field of organic chemistry and its various subfields has increased the value of structured reaction data. Most data in chemistry is represented by unstructured text, and due to the vastness of the organic chemistry literature (papers, patents), manual conversion from unstructured text to structured data remains a largely manual endeavor. Software tools for this task would facilitate downstream applications such as reaction prediction and condition recommendation. In this study, we leverage the power of fine-tuned large language models (LLMs) to extract reaction information from organic synthesis procedure text into structured data following the Open Reaction Database (ORD) schema, a comprehensive data structure designed for organic reactions. The fine-tuned model produces syntactically correct ORD records with an average accuracy of 91.25% for ORD “messages” (e.g., full compound, workups, or condition definitions) and 92.25% for individual data fields (e.g., compound identifiers, mass quantities), with the ability to recognize compound-referencing tokens and to infer reaction roles. We investigate its failure modes and evaluate performance on specific subtasks such as reaction role classification.
更多查看译文
AI 理解论文
溯源树
样例
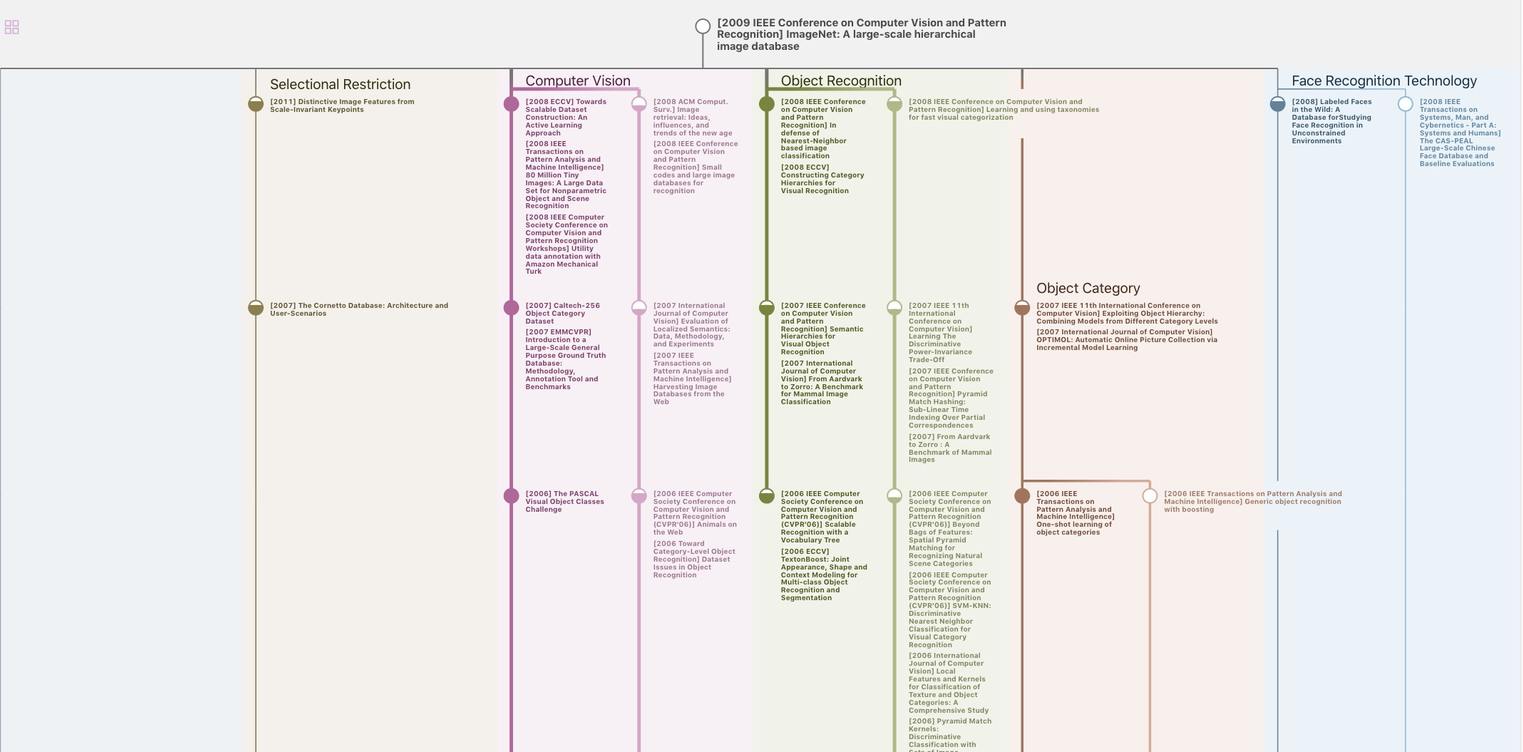
生成溯源树,研究论文发展脉络
Chat Paper
正在生成论文摘要