BMFS: Bidirectional weighted approach for multi-label feature selection algorithm
crossref(2024)
摘要
Abstract Shortcomings of the existing multi-label feature selection algorithms, such as non-considering the correlation of label space, ignoring the possible difference of label importance in feature space, cause the selected features to not accurately and comprehensively describe the sample, and make the calculation process complex. To solve these problems, this paper proposes an improved affinity propagation (AP) clustering method. Primarily in this method, the label space is divided according to the label correlation, and the label importance measurement and feature weight are also introduced to simplify the calculation. A multi-label rough membership function is proposed based on the definitions of the multi-label classification margin and the adaptive neighborhood radius and the neighborhood class , and then the multi-label fuzzy neighborhood rough set model is constructed by mapping the rough set to the fuzzy set, in which the fuzzy neighborhood entropy and multi-label fuzzy neighborhood entropy are defined. Finally, the bidirectional weighted multi-label feature selection algorithm is proposed based on fuzzy neighborhood entropy(BMFS). The experimental results on 10 multi-label datasets under Multi-label K-Nearest Neighbor (MLKNN) classifier show effectiveness and feasibility of this algorithm.
更多查看译文
AI 理解论文
溯源树
样例
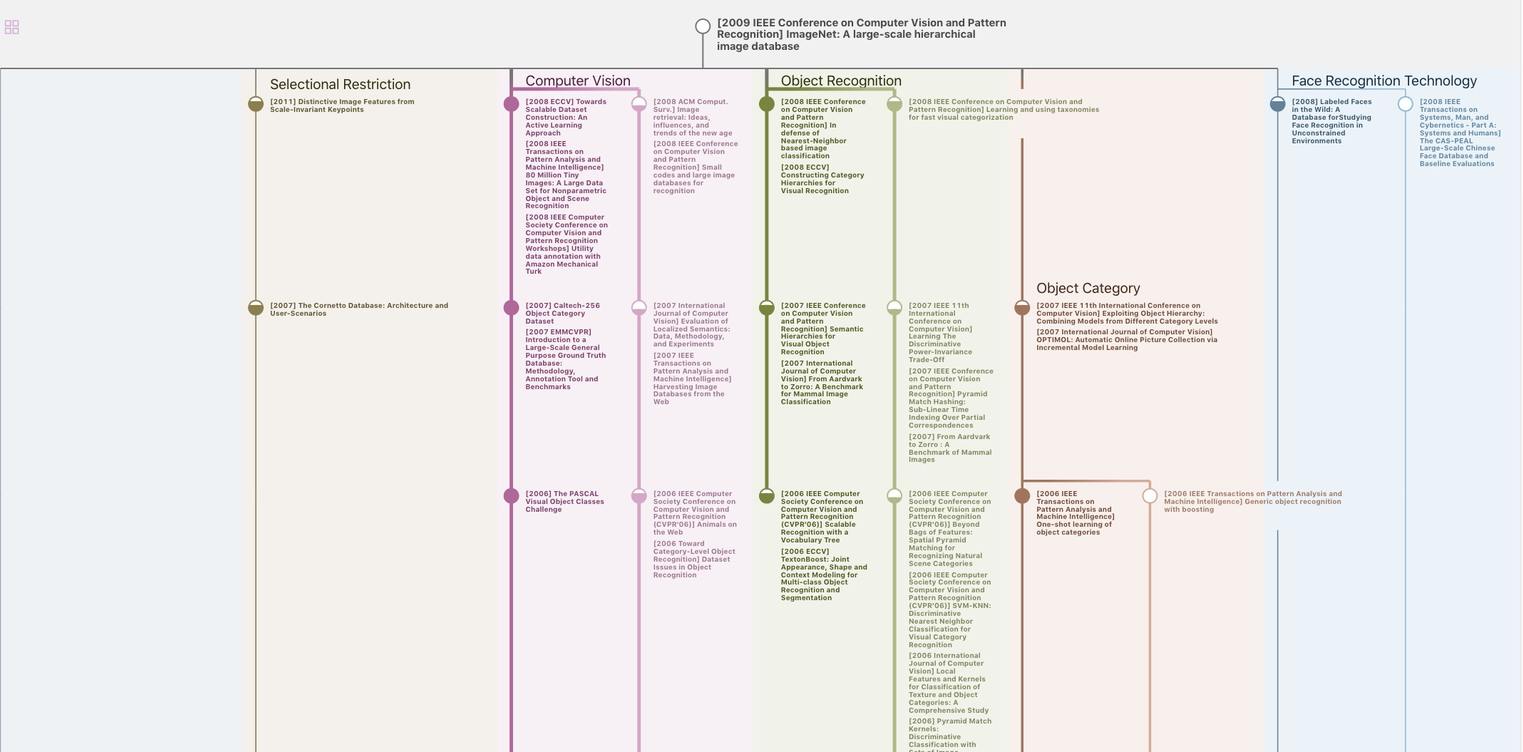
生成溯源树,研究论文发展脉络
Chat Paper
正在生成论文摘要