Hyperdimensional computing with holographic and adaptive encoder
FRONTIERS IN ARTIFICIAL INTELLIGENCE(2024)
摘要
Introduction Brain-inspired computing has become an emerging field, where a growing number of works focus on developing algorithms that bring machine learning closer to human brains at the functional level. As one of the promising directions, Hyperdimensional Computing (HDC) is centered around the idea of having holographic and high-dimensional representation as the neural activities in our brains. Such representation is the fundamental enabler for the efficiency and robustness of HDC. However, existing HDC-based algorithms suffer from limitations within the encoder. To some extent, they all rely on manually selected encoders, meaning that the resulting representation is never adapted to the tasks at hand.Methods In this paper, we propose FLASH, a novel hyperdimensional learning method that incorporates an adaptive and learnable encoder design, aiming at better overall learning performance while maintaining good properties of HDC representation. Current HDC encoders leverage Random Fourier Features (RFF) for kernel correspondence and enable locality-preserving encoding. We propose to learn the encoder matrix distribution via gradient descent and effectively adapt the kernel for a more suitable HDC encoding.Results Our experiments on various regression datasets show that tuning the HDC encoder can significantly boost the accuracy, surpassing the current HDC-based algorithm and providing faster inference than other baselines, including RFF-based kernel ridge regression.Discussion The results indicate the importance of an adaptive encoder and customized high-dimensional representation in HDC.
更多查看译文
关键词
brain-inspired computing,hyperdimensional computing,holographic representation,vector function architecture,efficient machine learning
AI 理解论文
溯源树
样例
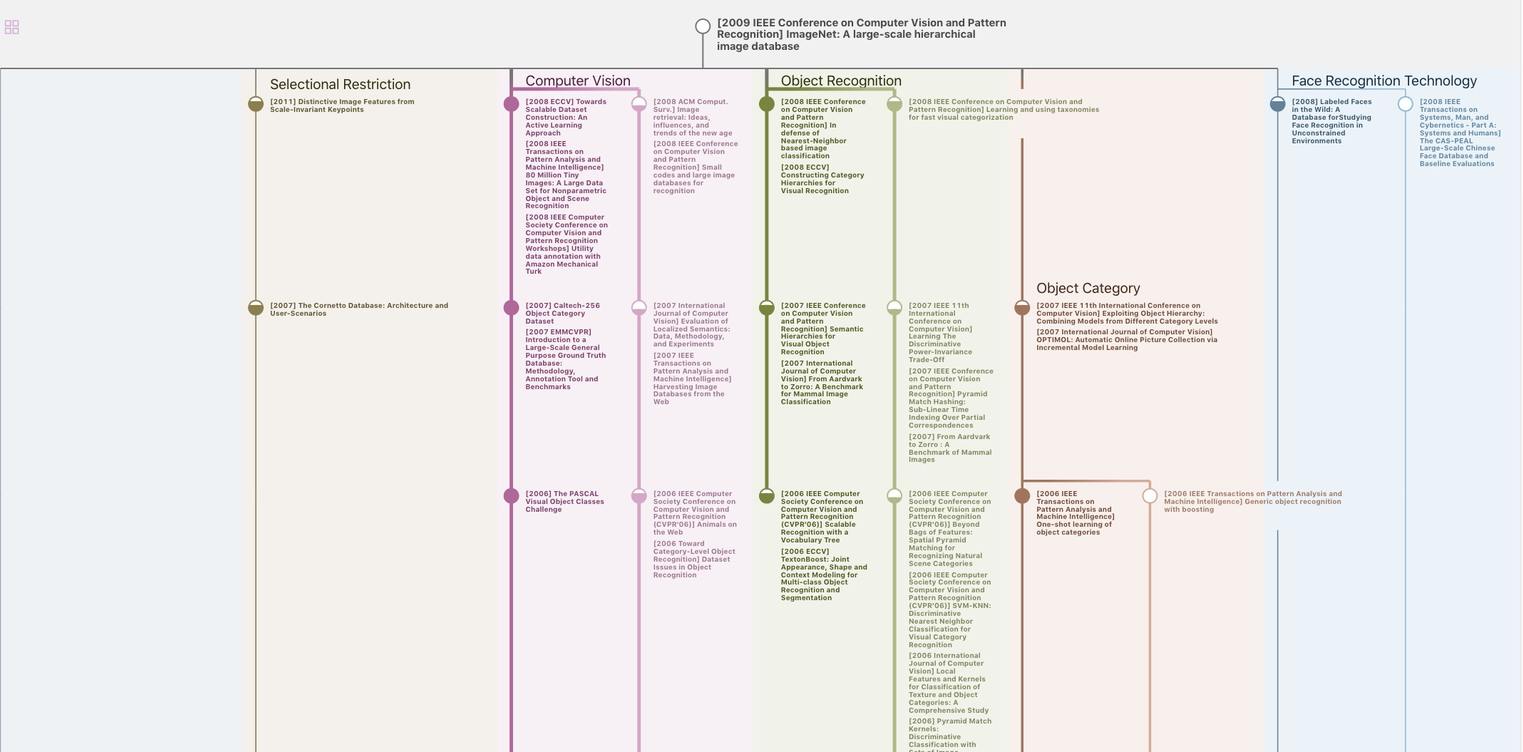
生成溯源树,研究论文发展脉络
Chat Paper
正在生成论文摘要