Multivariate Technique for Detecting Variations in High-Dimensional Imagery
IEEE ACCESS(2024)
摘要
The field of immunology requires refined techniques to identify detailed cellular variance in high-dimensional images. Current methods mainly capture general immune cell proportion variations and often overlook specific deviations in individual patient samples from group baseline. We introduce a simple technique that integrates Hotelling's T-2 statistic with random projection (RP) methods, specifically designed to identify changes in immune cell composition in high-dimensional images. Uniquely, our method provides deeper insights into individual patient samples, allowing for a clearer understanding of group differences. We assess the efficacy of the technique across various RPs: Achlioptas (AP), plus-minus one (PM), Li, and normal projections (NP), considering shift size, dimension reduction, and image dimensions. Simulations reveal variable detection performances across RPs, with PM outperforming and Li lagging. Practical tests using single-cell images of basophils (BAS) and promyelocytes (PMO) emphasise their utility for individualised detection. Our approach elevates high-dimensional image data analysis, particularly for identifying shifts in immune cell composition. This breakthrough potentially transforms healthcare practitioners' cellular interpretation of the immune landscape, promoting personalised patient care, and reshaping the discernment of diverse patient immune cell samples.
更多查看译文
关键词
Dimensionality reduction,high-dimension data,image monitoring,multivariate Shewhart control chart,quality control in healthcare,random projection methods
AI 理解论文
溯源树
样例
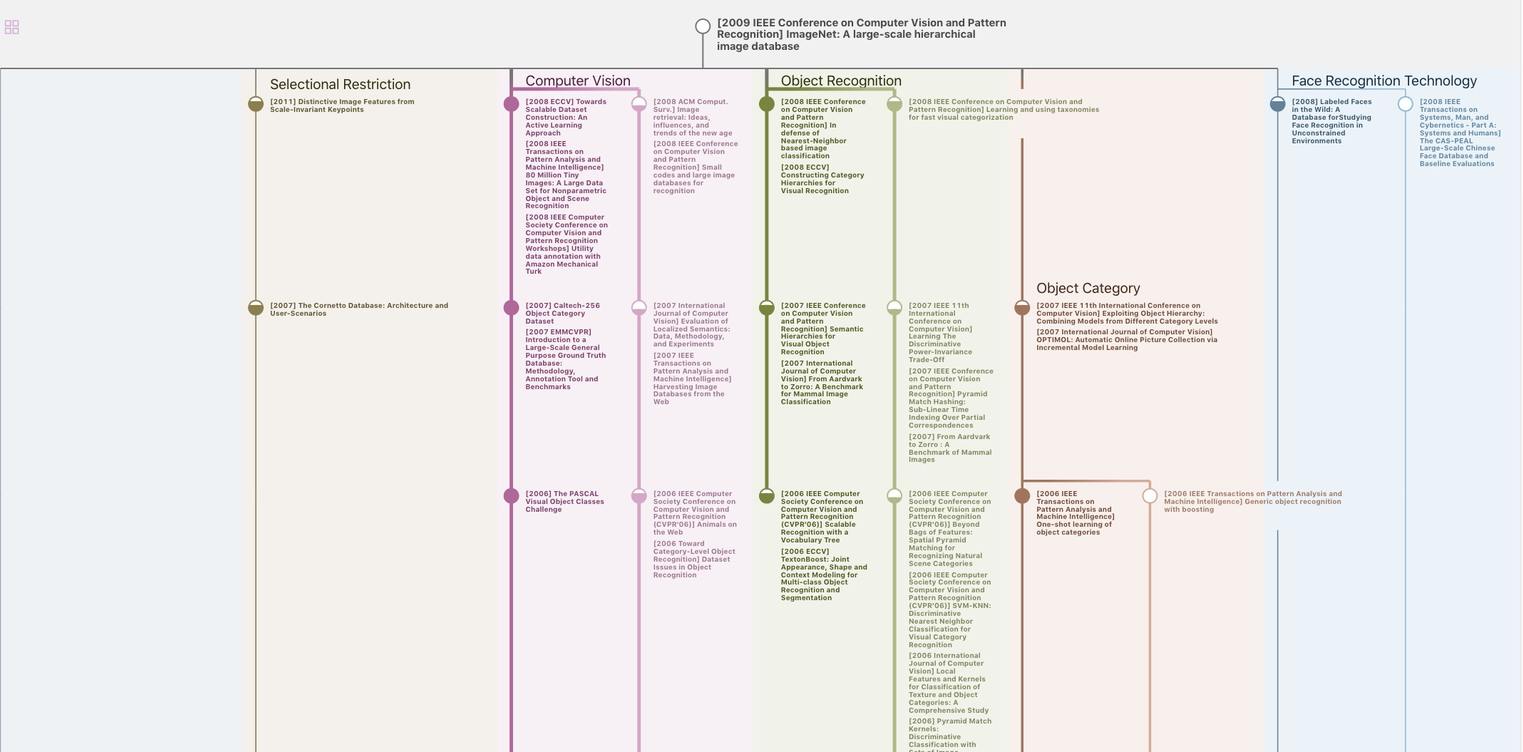
生成溯源树,研究论文发展脉络
Chat Paper
正在生成论文摘要