Predicting Ocean Wave Fun Factor with Machine Learning Using Buoy Data and Human Observations
2023 Congress in Computer Science, Computer Engineering, & Applied Computing (CSCE)(2023)
摘要
This study explores a niche of ocean wave prediction by using machine learning to predict ocean waves' “fun factor,” which estimates how enjoyable surfing the waves would be. We created several machine learning models to achieve satisfactory results, including Random Forest regression and k-Nearest Neighbors. The data used to train these models came from a buoy in Wrightsville Beach, NC, and a business local to Wrightsville Beach called WBLiveSurf. WBLiveSurf's employees post a report approximately four times daily, including fun factor, swell size, and water surface. These reports were merged with the buoy data by timestamp and supplied to the various machine learning models implemented in this study. We also developed models to act as surrogates for swell size and water surface as a supplement. These values are human-observed and thus subject to variation and error. Further, the short-lived nature of a wave's peak amplifies this error. We discuss how we deal with the inconsistency introduced in the WBLiveSurf data due to the individual reporting changing throughout the day and week. We discuss the results of the various models, potential limitations, and a proposed model chain to estimate fun factor in real-time and the future at Wrightsville Beach.
更多查看译文
关键词
Deep learning,Machine learning,Wave-condition forecasting
AI 理解论文
溯源树
样例
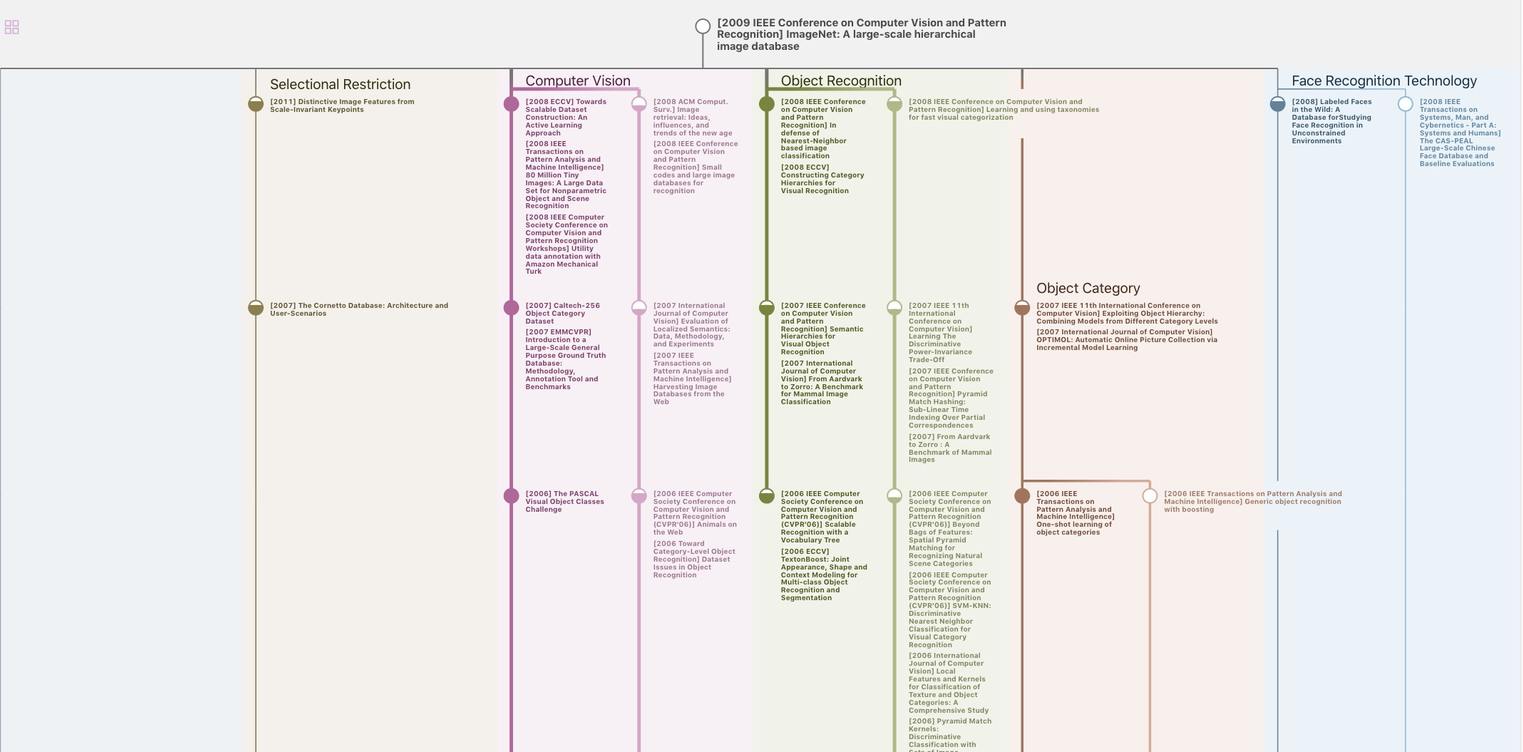
生成溯源树,研究论文发展脉络
Chat Paper
正在生成论文摘要