Integrated Road Information Perception Framework for Road Type Recognition and Adaptive Evenness Assessment
SAE Technical Paper Series(2024)
摘要
With the rapid advancement in intelligent vehicle technologies, comprehensive environmental perception has become crucial for achieving higher levels of autonomous driving. Among various perception tasks, monitoring road types and evenness is particularly significant. Different road categories imply varied surface adhesion coefficients, and the evenness of the road reflects distinct physical properties of the road surface. This paper introduces a two-stage road perception framework. Initially, the framework undergoes pre-training on a large annotated drivable area dataset, acquiring a set of pre-trained parameters with robust generalization capabilities, thereby endowing the model with the ability to locate road areas in complex regions. Subsequently, guided by a mask attention mechanism, the model undergoes fine-tuning on a smaller dataset annotated with road type regions using a weighted joint loss function, effectively addressing issues of class imbalance and limited labeled samples. Moreover, a hole-filling algorithm is employed to rectify missed detection areas in road perception. For monitoring road evenness, a simplified vehicular kinematics model is used, coupled with state information such as vehicle turning angle and speed, to predict the vehicle’s forward anticipatory trajectory. Within the overlapping region of this trajectory and the drivable area, pure road samples are adaptively extracted for assessing the evenness of the road. Experimental results demonstrate that our method can accurately perceive road information in advance, thereby laying a foundation for enhancing the driving safety and comfort of intelligent vehicles in complex environments, as well as for adaptive control.
更多查看译文
AI 理解论文
溯源树
样例
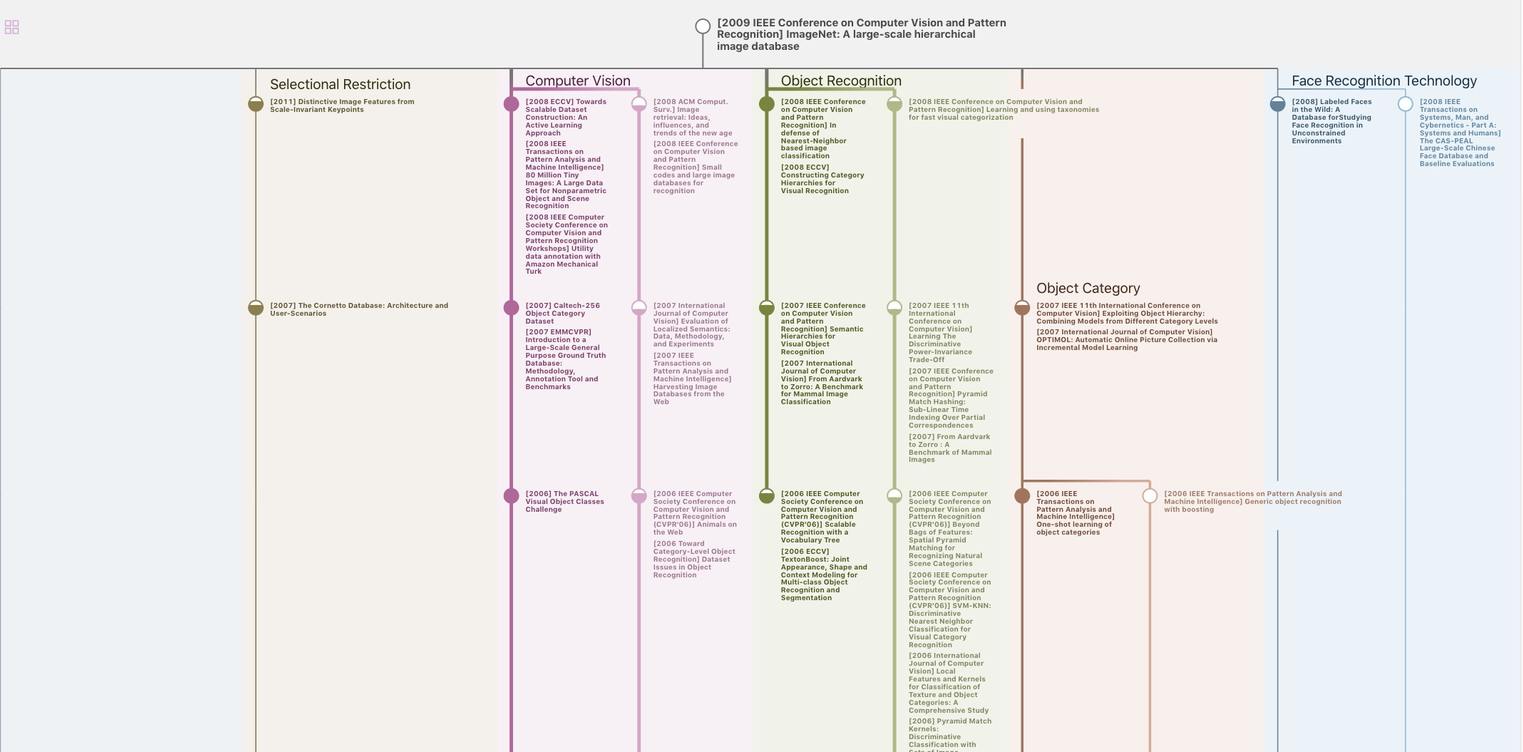
生成溯源树,研究论文发展脉络
Chat Paper
正在生成论文摘要