A quantum information theoretic analysis of reinforcement learning-assisted quantum architecture search
arxiv(2024)
摘要
In the field of quantum computing, variational quantum algorithms (VQAs)
represent a pivotal category of quantum solutions across a broad spectrum of
applications. These algorithms demonstrate significant potential for realising
quantum computational advantage. A fundamental aspect of VQAs involves
formulating expressive and efficient quantum circuits (namely ansatz) and
automating the search of such ansatz is known as quantum architecture search
(QAS). RL-QAS involves optimising QAS using reinforcement learning techniques.
This study investigates RL-QAS for crafting ansatzes tailored to the
variational quantum state diagonalization problem. Our investigation includes a
comprehensive analysis of various dimensions, such as the entanglement
thresholds of the resultant states, the impact of initial conditions on the
performance of RL-agent, the phase change behavior of correlation in
concurrence bounds, and the discrete contributions of qubits in deducing
eigenvalues through conditional entropy metrics. We leverage these insights to
devise an optimal, admissible QAS to diagonalize random quantum states.
Furthermore, the methodologies presented herein offer a generalised framework
for constructing reward functions within RL-QAS applicable to variational
quantum algorithms.
更多查看译文
AI 理解论文
溯源树
样例
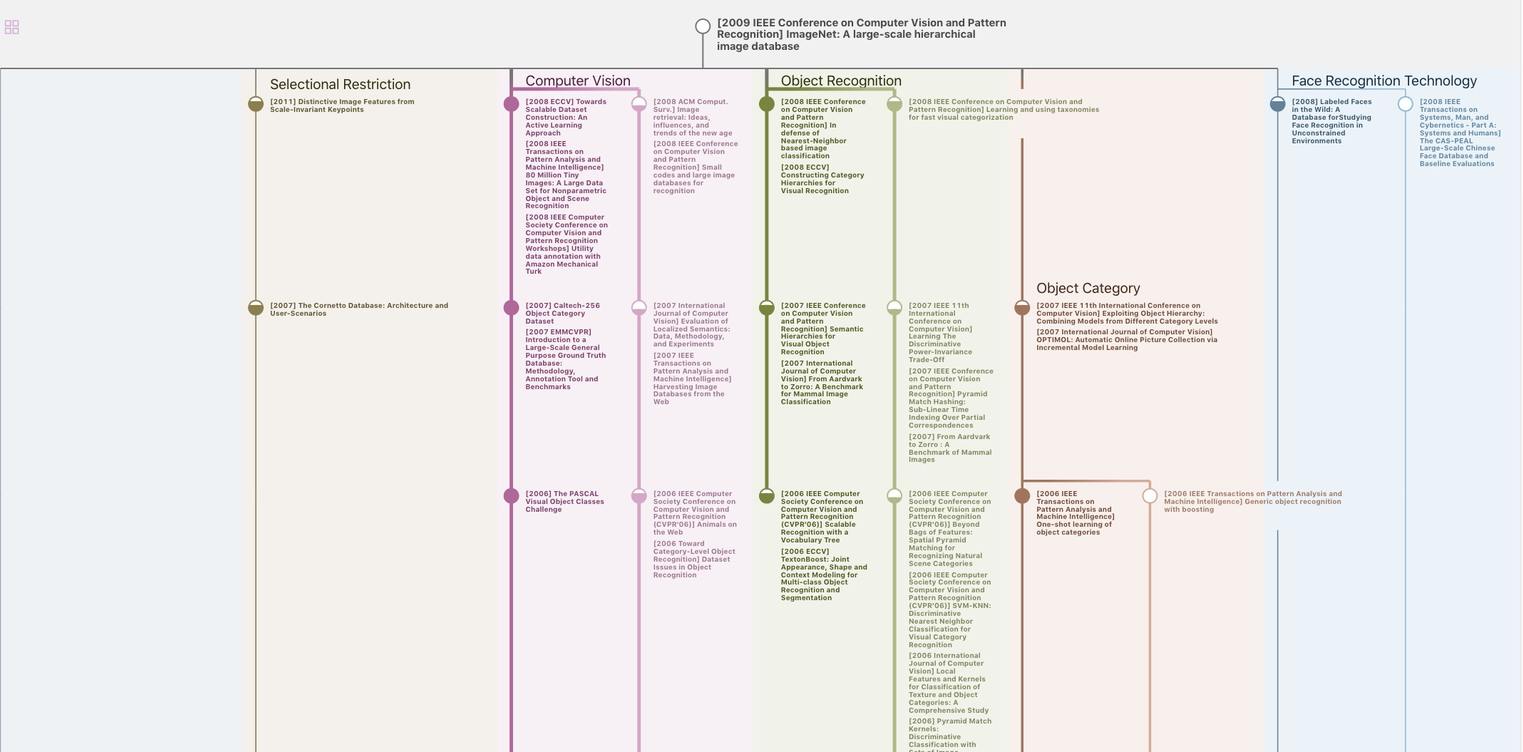
生成溯源树,研究论文发展脉络
Chat Paper
正在生成论文摘要