A Robust Anomaly Detection Model for Pumps Based on the Spectral Residual With Self-Attention Variational Autoencoder
IEEE Transactions on Industrial Informatics(2024)
摘要
Multivariate time-series (MTS) collected from multiple sensors on industrial pumps often exhibit concept drift and noise contamination due to variable working conditions and complex environments. To detect anomalies in such MTS, we propose a novel model called spectral residual with self-attention variational autoencoder (SR-SAVAE). Specifically, the spectral residual operation is used to mitigate concept drift, while the variational inference combined with a total variation regularization is used to address the issue of noise contamination. Experimental results on three public datasets indicate that the SR-SAVAE model achieves good anomaly detection results for general MTS. More importantly, compared to other state-of-the-art models on a private dataset about pumps, the results illustrate the superiority of the SR-SAVAE model in anomaly detection for MTS with concept drift and noise contamination. Finally, ablation studies on the SR-SAVAE model detail the efficacy of each component.
更多查看译文
关键词
Anomaly detection,concept drift,industrial pumps,multivariate time-series,noise contamination,self-attention,spectral residual,variational inference
AI 理解论文
溯源树
样例
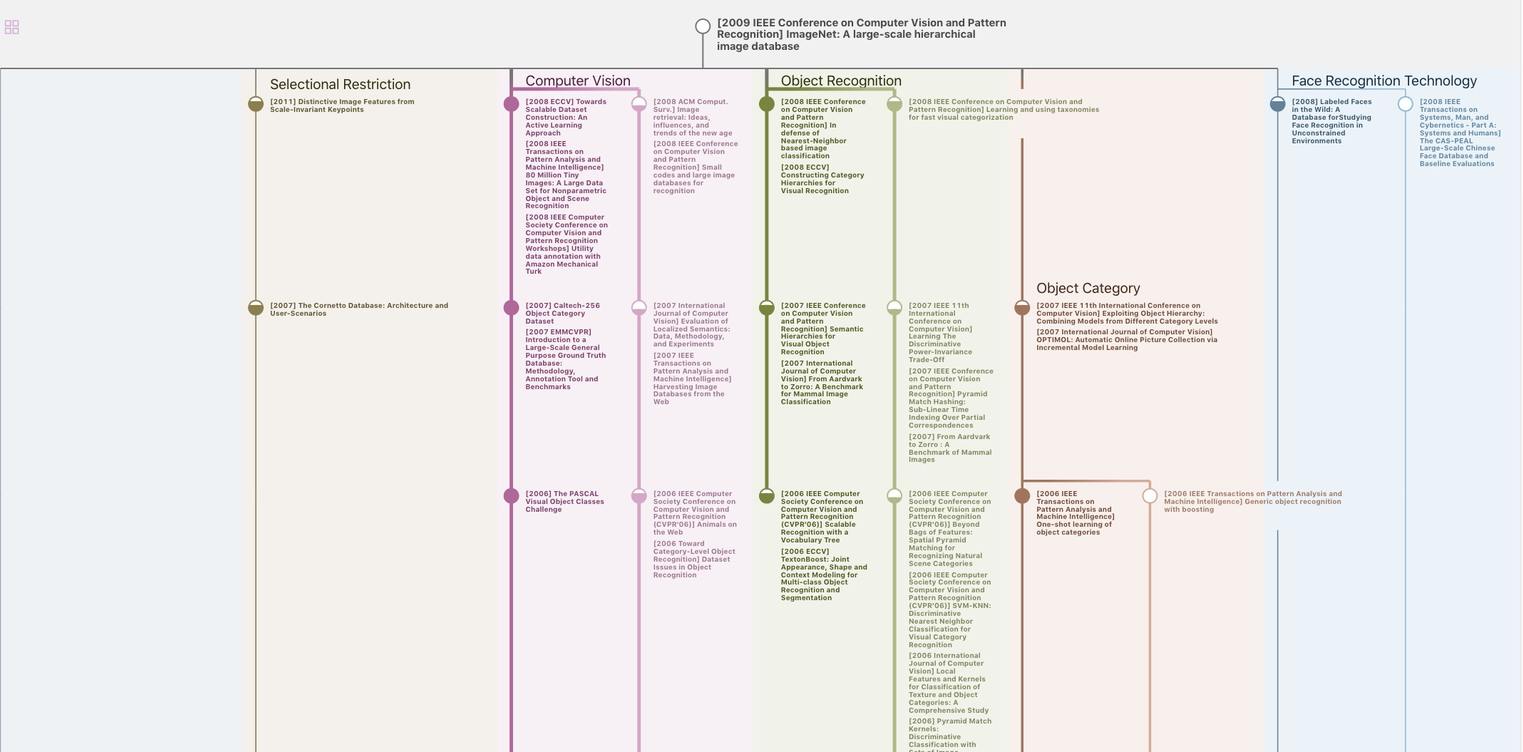
生成溯源树,研究论文发展脉络
Chat Paper
正在生成论文摘要