Estimating Blood Alcohol Level Through Facial Features for Driver Impairment Assessment.
IEEE/CVF Winter Conference on Applications of Computer Vision(2024)
摘要
Drunk driving-related road accidents contribute significantly to the global burden of road injuries. Addressing alcohol-related harm, particularly during safety-critical activities like driving, requires real-time monitoring of an individual’s blood alcohol concentration (BAC). We devise an in-vehicle machine learning system that harnesses standard commercial RGB cameras to predict critical levels of BAC. Our system can detect instances of alcohol intoxication impairment as subtle as 0.05 g/dL (WHO recommended legal limit for driving), with an accuracy of 75%, by leveraging the physiological manifestations of alcohol intoxication on a driver’s face. This system holds great promise for improving road safety. In tandem, we have compiled a data set of 60 subjects engaged in simulated driving scenarios, spanning three levels of alcohol intoxication. These scenarios were captured and divided into video segments labeled "sober","low’, and "severe" Alcohol Intoxication Impairment (AII), constituting the basis for evaluating our system’s performance. To the best of our knowledge, this study is the first to create a large-scale real-life dataset of alcohol intoxication and assess intoxication levels using an off-the-shelf RGB camera to detect drunk driving.
更多查看译文
关键词
Algorithms,Datasets and evaluations,Algorithms,Video recognition and understanding,Applications,Embedded sensing / real-time techniques
AI 理解论文
溯源树
样例
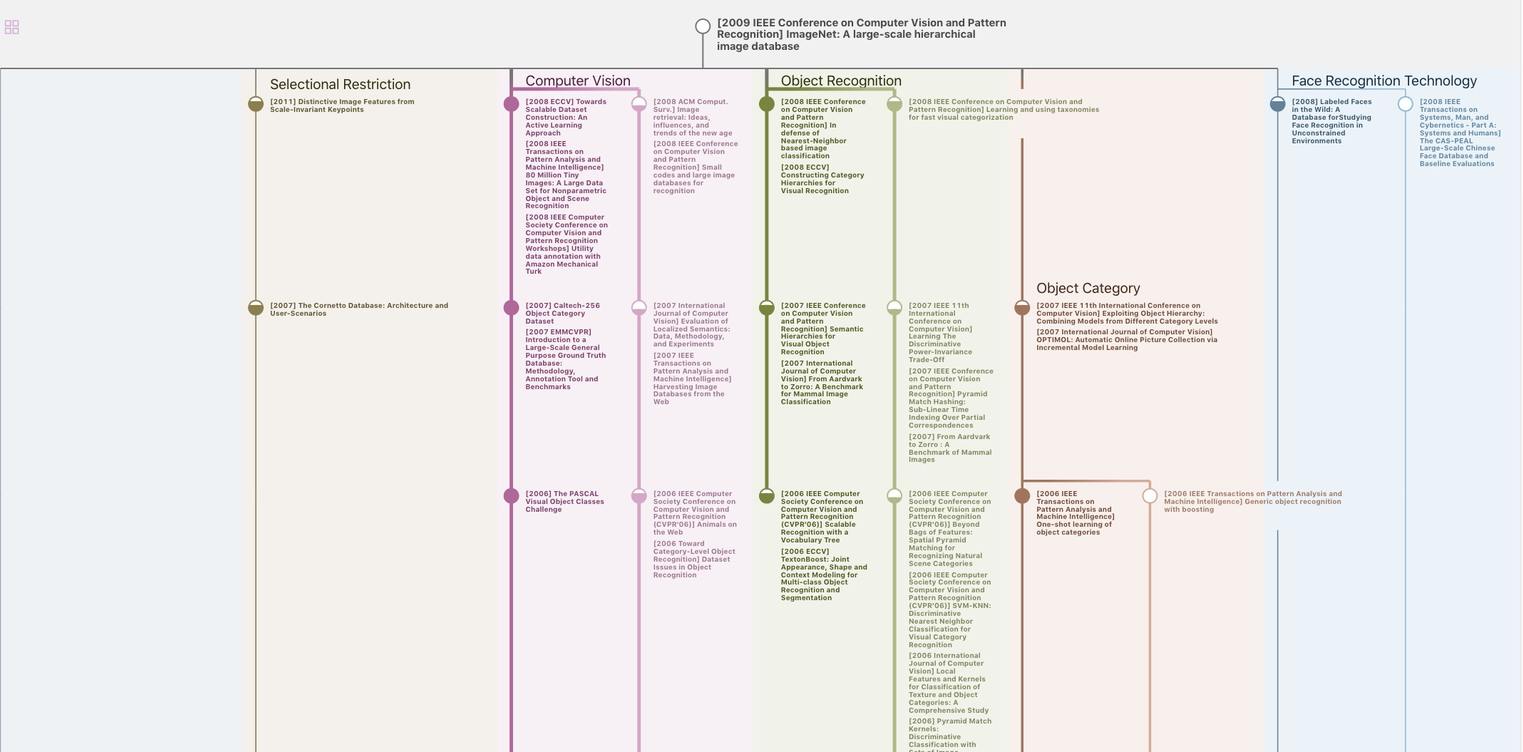
生成溯源树,研究论文发展脉络
Chat Paper
正在生成论文摘要