Predicting Atmospheric Air Pollution: A Convolutional- Transformer Approach for Spatial and Temporal Analysis of PM2.5
2023 Congress in Computer Science, Computer Engineering, & Applied Computing (CSCE)(2023)
摘要
6.7 million premature deaths occur annually due to household and ambient air pollution. Air pollution affects individuals globally and can be derived from a variety of factors including household cooking fuel, motor vehicles, industrial practices, and natural fires. To tackle this global crisis, our research focuses on understanding the spatial and temporal patterns between air pollutants to predict future levels of air pollutants. Our approach uses a novel deep learning methodology that involves a spatiotemporal Convolutional-Transformer archi-tecture (ConvTransformer) We harnessed multiple data sources as inputs to our ConvTransformer, including several remote sensing instruments such as NASA's Terra+Aqua satellites with the Moderate Resolution Imaging Spectroradiometer (MODIS), and the European Space Agency's Sentinel-5P satellite with the Tropospheric Monitoring Instrument (TROPOMI), gridMET ground-based meteorological remote-sensing data from North American Land Data (NLDAS-2), US Census TIGER Roadways, and ground-level PM2.5 sensing data sourced from EPA AirNow. Our approach shows a 38.8% decrease in 5-frame average SSIM error compared to existing modern deep learning architectures utilizing remote-sensing data, satellite imagery, and ground-level data for PM2.5 prediction.
更多查看译文
关键词
Air Pollution,Atmospheric Air Pollution,Data Sources,Deep Learning,Spatial Patterns,Meteorological Data,Satellite Imagery,Moderate Resolution Imaging Spectroradiometer,US Census,European Space Agency,Ambient Air Pollution,Remote Sensing Data,Ground-based Data,Structural Similarity Index Measure,Modern Deep Learning,Satellite Imagery Data,Rainfall,Root Mean Square Error,Geographic Regions,Input Features,Aerosol Optical Depth,Planetary Boundary Layer,Graph Convolutional Network,Topographic Maps,Attention Mechanism,Attention Scores,Nitrogen Dioxide,Air Pollution Measurements,Carbon Monoxide,Hourly Data
AI 理解论文
溯源树
样例
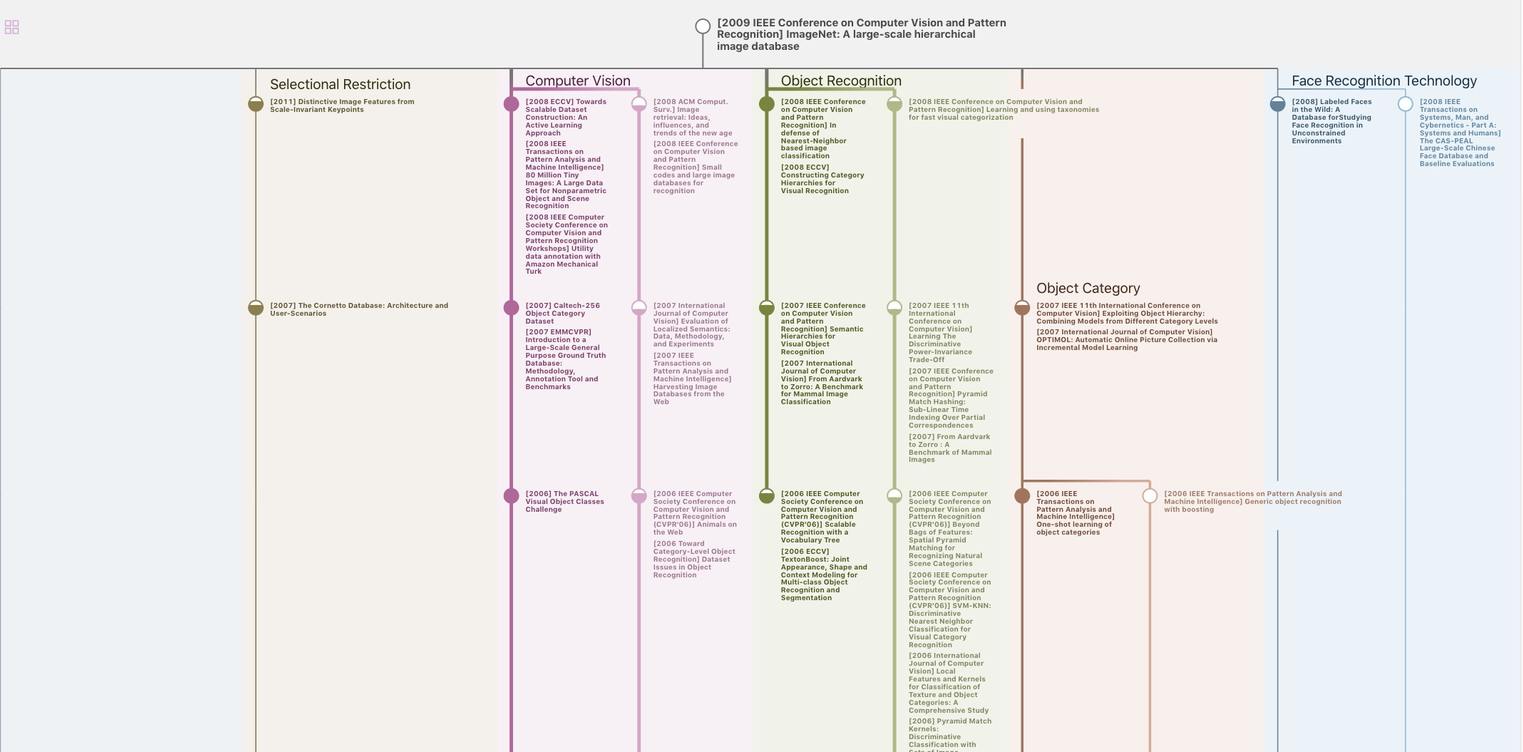
生成溯源树,研究论文发展脉络
Chat Paper
正在生成论文摘要