Massive MIMO-FDD Self-Attention CSI Feedback Networkfor Outdoor Environments
crossref(2024)
摘要
Abstract In situations where traditional feedback methods face high complexity and rely on channel sparsity,many deep learning-based CSI compression feedback methods have shown the potential to fullycapture the diversity and multiplexing gains of Massive MIMO technology in Frequency DivisionDuplex (FDD) mode. In order to further enhance the accuracy of obtaining downlink channel stateinformation at the base station side, this paper proposes a novel neural network, SfNet. Basedon visual analysis, the convolutional layers in the encoder are designed to extract long-distancetime-delay correlations of the same antenna across different subcarriers. The SimAM module isintroduced to mitigate clustering effects. In the decoder, a spatial-temporal joint modeling approachis presented, utilizing the CBAM module to preserve the original spatial structure features lostduring the dimension reduction of the CSI matrix to sequence data. Subsequently, two layers ofTransformer multi-head attention are employed to achieve more global time-series modeling.Theexperimental results indicate that, compared to CLNet, the average complexity of SfNet’s encoder isreduced by 3.4%. It meets the demand for lightweight devices on the user side. In terms of accuracy,SfNet exhibits an average improvement of 30.17% compared to TransNet in outdoor environments.
更多查看译文
AI 理解论文
溯源树
样例
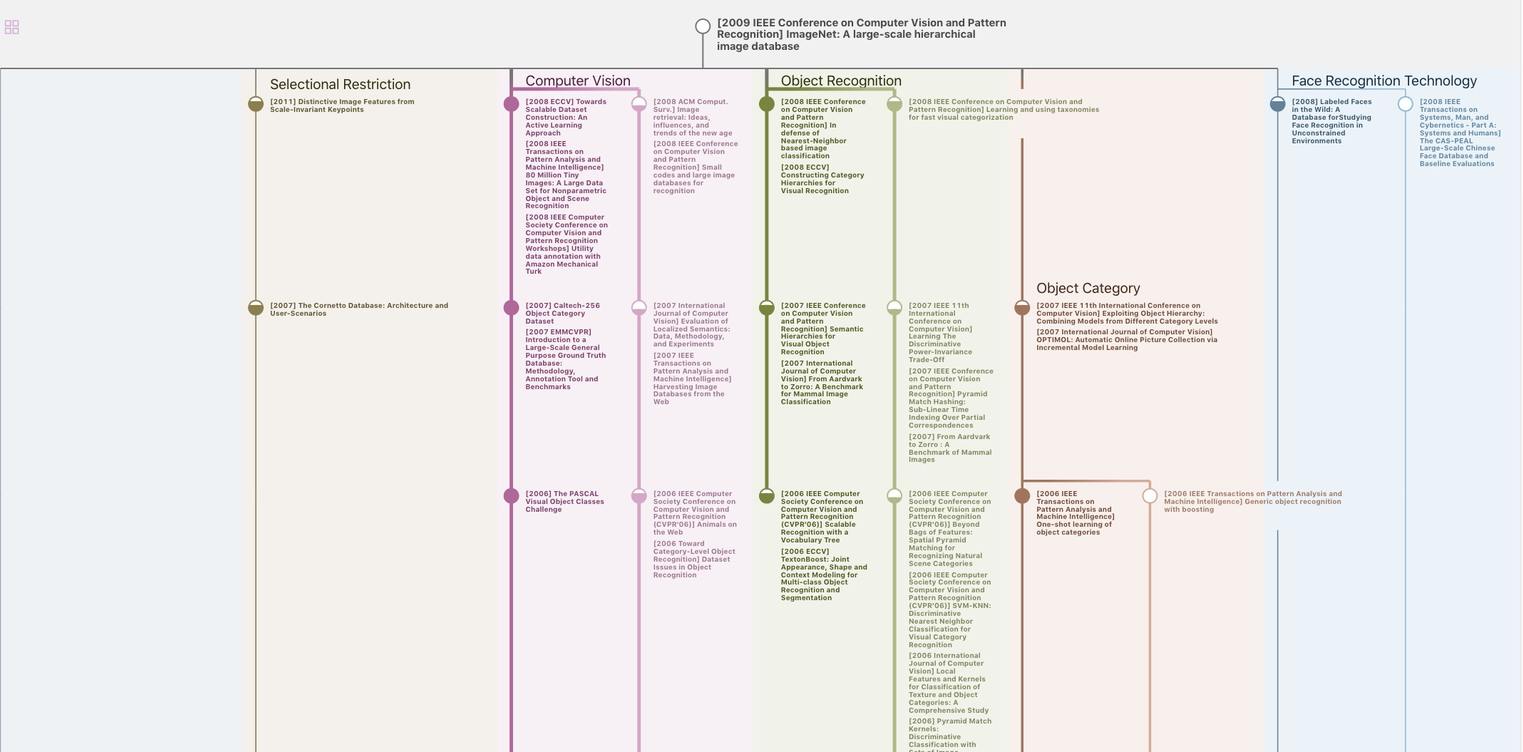
生成溯源树,研究论文发展脉络
Chat Paper
正在生成论文摘要