Skin Cancer Prediction Using CNN-Based Decision Fusion with Dermoscopic Images
2023 Congress in Computer Science, Computer Engineering, & Applied Computing (CSCE)(2023)
摘要
Skin cancer is a type of cancer that develops in the cells of the skin and can affect any part of the body. Early-stage detection is crucial for the effective treatment and curability of this cancer. Skin cancer diagnosis includes visual screening, dermoscopic image analysis, biopsy, and histopathological examination. The accuracies of these diagnostic procedures are highly dependent on the experience of dermatologists. However, the number of experts in this field is limited. Therefore, automatic skin cancer detection from skin lesion images is an increasingly important field that can aid in medical research. This research proposes a Convolutional Neural Network (CNN) architecture-based decision-level fusion model to predict skin cancer from dermoscopic images with improved accuracy. In so doing, the research uses majority voting decision fusion to combine three individual CNN models. The models are validated using the International Skin Imaging Collaboration (ISIC) 2018 challenges dataset available in the ISIC archive to predict binary and multiclass skin cancer with validation accuracy of 0.83±0.01 and 0.91±0.01, respectively. Paired t-tests are conducted to test the difference in mean accuracies between the fused and best-performing individual models. The test results show that the fused models' mean accuracy is significantly better at a 5% significance level than the best individual models for both cases. The performance of the binary fused model is further compared to several state-of-the-art models and is found to outperform most of them, suggesting the considerable clinical significance.
更多查看译文
关键词
Dermoscopic image,melanoma detection,majority voting,paired t-test,decision fusion,CNN
AI 理解论文
溯源树
样例
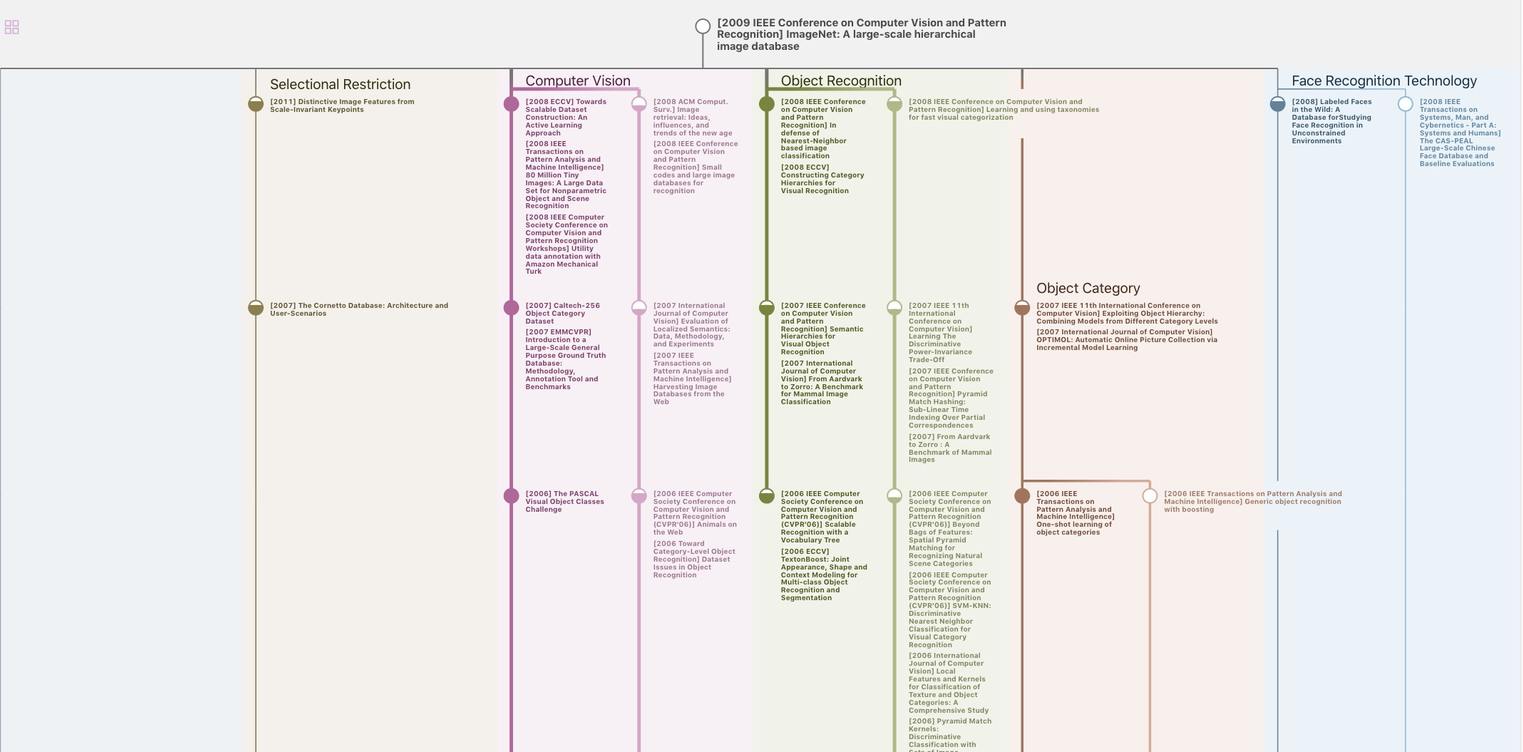
生成溯源树,研究论文发展脉络
Chat Paper
正在生成论文摘要