A Graph-Based Semi-Supervised Approach for Few-Shot Class-Incremental Modulation Classification
CHINA COMMUNICATIONS(2024)
摘要
With the successive application of deep learning (DL) in classification tasks, the DL -based modulation classification method has become the preference for its state-of-the-art performance. Nevertheless, once the DL recognition model is pre -trained with fixed classes, the pre -trained model tends to predict incorrect results when identifying incremental classes. Moreover, the incremental classes are usually emergent without label information or only a few labeled samples of incremental classes can be obtained. In this context, we propose a graphbased semi -supervised approach to address the fewshot classes -incremental (FSCI) modulation classification problem. Our proposed method is a twostage learning method, specifically, a warm-up model is trained for classifying old classes and incremental classes, where the unlabeled samples of incremental classes are uniformly labeled with the same label to alleviate the damage of the class imbalance problem. Then the warm-up model is regarded as a feature extractor for constructing a similar graph to connect labeled samples and unlabeled samples, and the label propagation algorithm is adopted to propagate the label information from labeled nodes to unlabeled nodes in the graph to achieve the purpose of incremental classes recognition. Simulation results prove that the proposed method is superior to other finetuning methods and retrain methods.
更多查看译文
关键词
deep learning,few-shot,label propaga- tion,modulation classification,semi-supervised learn- ing
AI 理解论文
溯源树
样例
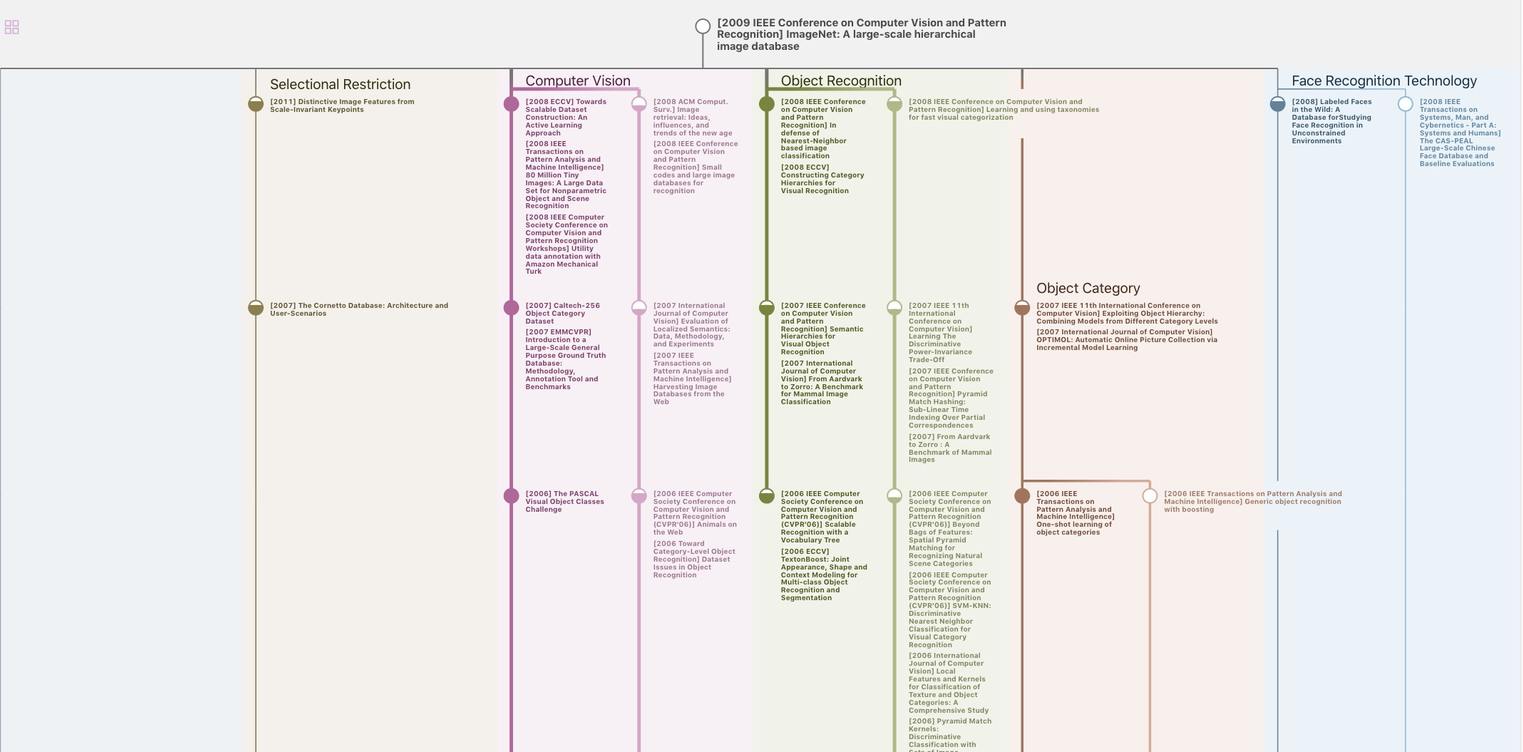
生成溯源树,研究论文发展脉络
Chat Paper
正在生成论文摘要