A Neuroevolution Approach to Keypoint-Based Sign Language Fingerspelling Classification.
IIAI-AAI-Winter(2023)
摘要
Non-verbal communication frameworks such as Sign Language and Makaton serve as a vital means of communication for millions of people with hearing impairments. The development of accurate and efficient recognition systems for non-verbal communication is of great importance towards fostering inclusion through accessible systems. In this paper, we propose a novel approach to improving fingerspelling recognition through the application of neuroevolution as a means to hyperheuristically improve deep neural networks. We propose the use of these algorithms to optimise the classification of low-dimensional datasets given the mixed levels of computational resources in the community setting. A dataset of 1678 images comprised of seven subjects performing ASL fingerspelling is processed into normalised keypoints, and three neuroevolution simulations are executed to search the problem space for the most effective topology. The results show that the simulation finds a promising set of hyperparameters, achieving a mean 10-fold cross-validation accuracy of 97.44% by using a total of 1478 hidden units within four layers. Our neuroevolution approach demonstrates remarkable potential for the enhancement of finger-spelling recognition in non-verbal communication systems, paving the way for more inclusive technologies in the future.
更多查看译文
关键词
Automated Algorithm,Evolving Learning,Human-Computer Interaction
AI 理解论文
溯源树
样例
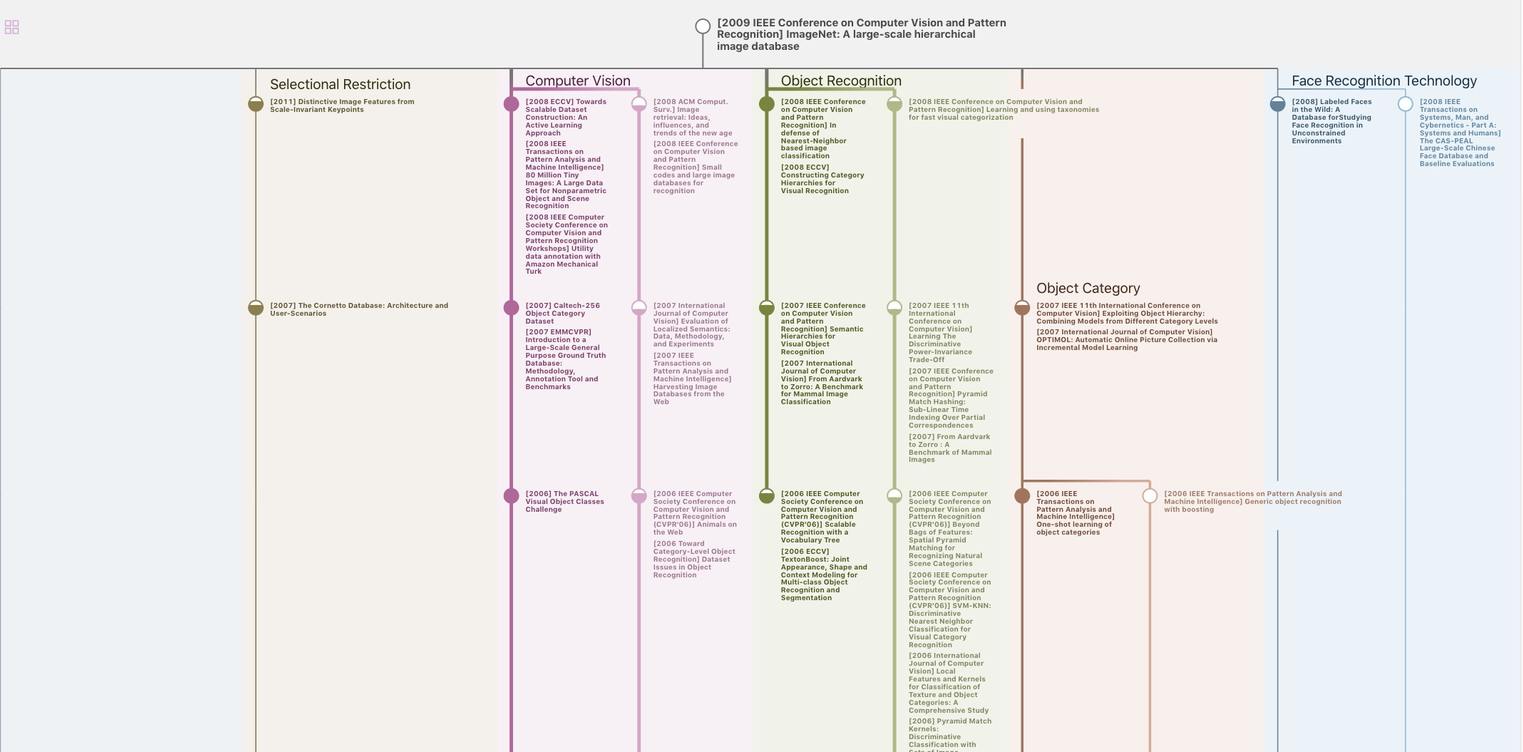
生成溯源树,研究论文发展脉络
Chat Paper
正在生成论文摘要