Assessing the improvement potentials of climate model partitioning and time-variant feature extraction for soil organic carbon prediction
CATENA(2024)
摘要
The monitoring of soil organic carbon (SOC) content is of significance for the global carbon cycle and the sustainability of soil quality under climate change. SOC prediction based on multi-source remote sensing data has been integrated well into different local regression strategies and model algorithms. However, the application of mixing local regression strategies with high generalizability and extracting more advanced information from time-variant data are rare. Here, we propose a climate model partitioning strategy, compared to common local regression strategies (soil classification and spectral clustering), with the aim of improving the accuracy of regional SOC content prediction. In this study, 1248 topsoil samples were collected in Northeast China. Environmental covariates representing soil-forming elements of meteorology, organisms, terrain and parent materials factors were explored, and then different time-variant covariate pre-processing were performed, and form Dataset I (conventional mean values of covariates) and Dataset II (shapelet features extracted from covariates) according to the data type. Next, we explored the effectiveness of global regression and local regression strategies (soil classification and five scenarios of Shared Socio-economic Pathways (SSPs)-based ant colony optimization clustering) for SOC prediction with a convolutional neural network (CNN) model. The results demonstrated that the optimal SOC content prediction model with the SSP245 local regression strategy and Dataset II as input yielded the lowest root mean square error (RMSE) of 5.83 g kg−1, the highest coefficient of determination (R2) and a ratio of performance to interquartile distance (RPIQ) of 0.73 and 1.99, respectively. Second, the order of SOC prediction accuracy among the different regression strategies was SSP245 > SSP119 > SSP370 > soil classification > SSP126 > SSP585 > global regression. Third, compared with Dataset I, the CNN model-based Dataset II had a 12 % increase in average R2 values, a 5.27 % decrease in RMSE, and a 4.27 % increase in RPIQ, which indicates that the shapelet feature extraction algorithm could better mine the information of time-variant variables in SOC content assessment. Finally, we identified that CNN could perform better in regions with low spatial heterogeneity. Our results suggest that the paradigm of “local regression + feature extraction” has great potential for SOC prediction and mapping, especially for larger scales.
更多查看译文
关键词
Soil organic carbon,Local regression,Shared Socio-economic Pathways (SSPs),Shapelet feature extraction,Convolutional neural network
AI 理解论文
溯源树
样例
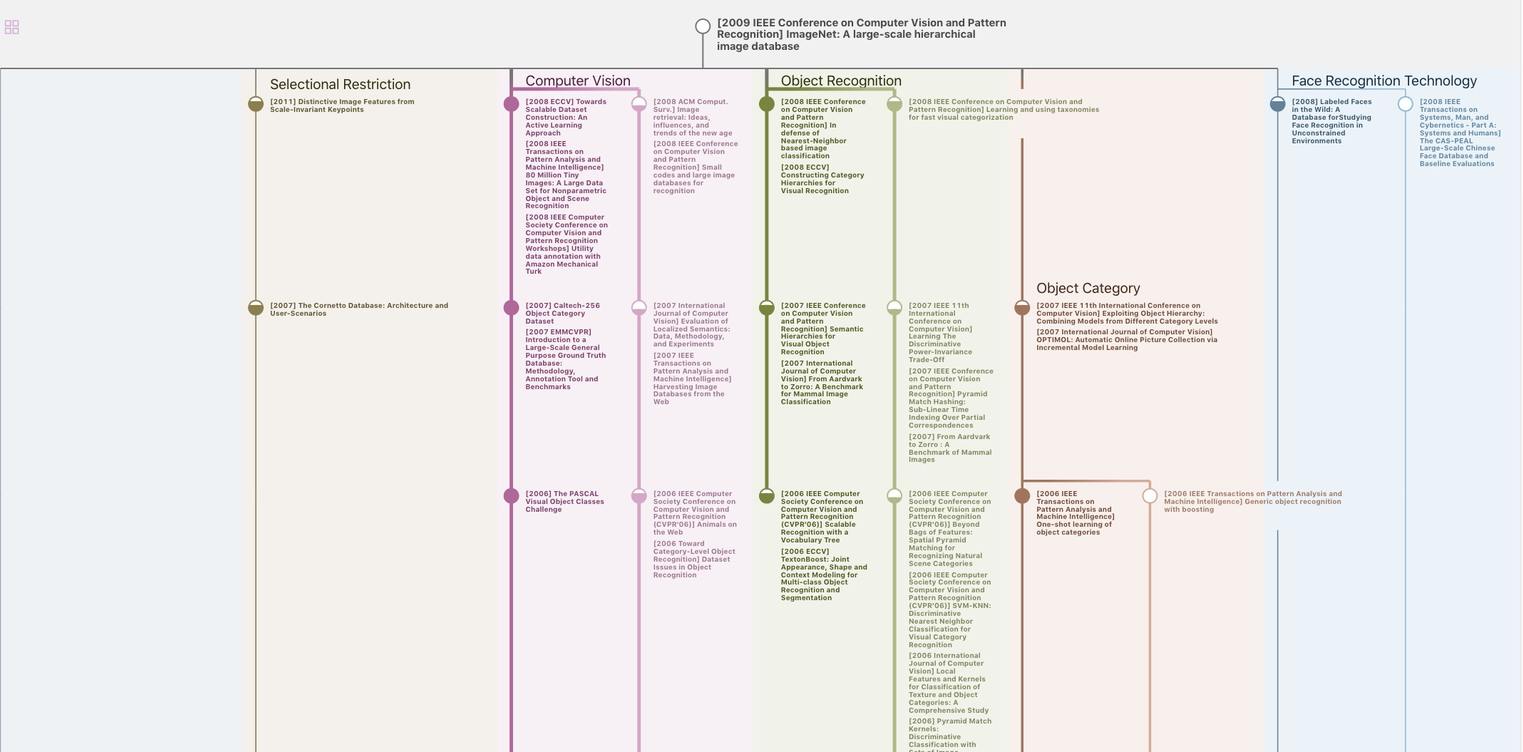
生成溯源树,研究论文发展脉络
Chat Paper
正在生成论文摘要