Improved flood depth estimation with SAR image, digital elevation model, and machine learning schemes
Journal of Hydrology: Regional Studies(2024)
摘要
Study region
Nhat Le river basin, the floodplains of Central coast of Vietnam.
Study focus
Flood disasters have a significant impact on population and economies worldwide. To accurately assess flood damage, it is crucial to estimate the water depth and predict the potential spread of damage. However, conventional methods of estimating flood depth can be time-consuming and costly due to the large amount of data required from hydraulic and rain-runoff models. To overcome these limitations, we propose a new approach that integrates a machine learning (ML) algorithm with a floodwater depth estimation tool (FwDET) based on SAR imagery and digital elevation models (DEMs). To evaluate our approach, we trained and tested six ML regression algorithms using 804 sets of inventory flood depth data from the historic flood on the Nhat Le River in Vietnam in October 2020 as the dependent variable. This approach significantly improves flood depth prediction accuracy, enabling a faster and more cost-effective estimation of flood damage.
New hydrological insights for the region
Our research shows that by combining ML with FwDET, we were able to significantly enhance water depth prediction accuracy. We observed a 25.05% increase in the R2 coefficient and a 13.46% increase in the R coefficient. We recommend integrating satellite imagery, DEM, and ML for flood depth predictions, especially for floodplains with similar topographical conditions as the Nhat Le basin. This approach offers a practical, cost-effective, and near real-time solution for flood response. Implementing this approach can help improve flood damage assessment and mitigate the potential impacts of flood disasters on communities and economies.
更多查看译文
关键词
Flood depth,FwDET,Machine learning,Nhat Le,Vietnam
AI 理解论文
溯源树
样例
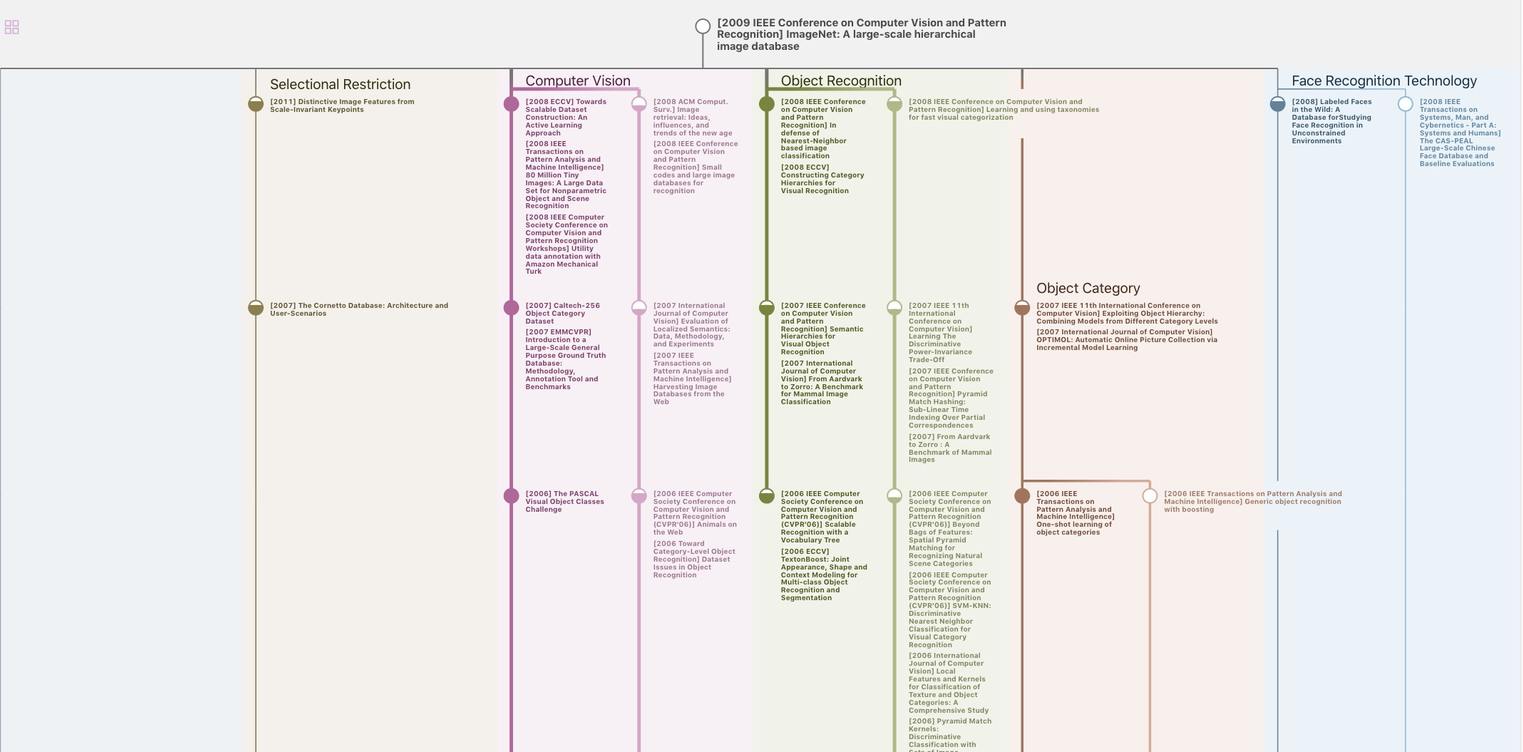
生成溯源树,研究论文发展脉络
Chat Paper
正在生成论文摘要