F2S-Net: learning frame-to-segment prediction for online action detection
Journal of Real-Time Image Processing(2024)
Abstract
Online action detection (OAD) aims at predicting action per frame from a streaming untrimmed video in real time. Most existing approaches leverage all the historical frames in the sliding window as the temporal context of the current frame since single-frame prediction is often unreliable. However, such a manner inevitably introduces useless even noisy video content, which often misleads action classifier when recognizing the ongoing action in the current frame. To alleviate this difficulty, we propose a concise and novel F2S-Net, which can adaptively discover the contextual segments in the online sliding window, and convert current frame prediction into relevant-segment prediction. More specifically, as the current frame can be either action or background, we develop F2S-Net with a distinct two-branch structure, i.e., the action (or background) branch can exploit the action (or background) segments. Via multi-level action supervision, these two branches can complementarily enhance each other, allowing to identify the contextual segments in the sliding window to robustly predict what is ongoing. We evaluate our approach on popular OAD benchmarks, i.e., THUMOS-14, TVSeries and HDD. The extensive results show that our F2S-Net outperforms the recent state-of-the-art approaches.
MoreTranslated text
Key words
Online action detection,Contextual segment prediction,Multi-level action supervision
AI Read Science
Must-Reading Tree
Example
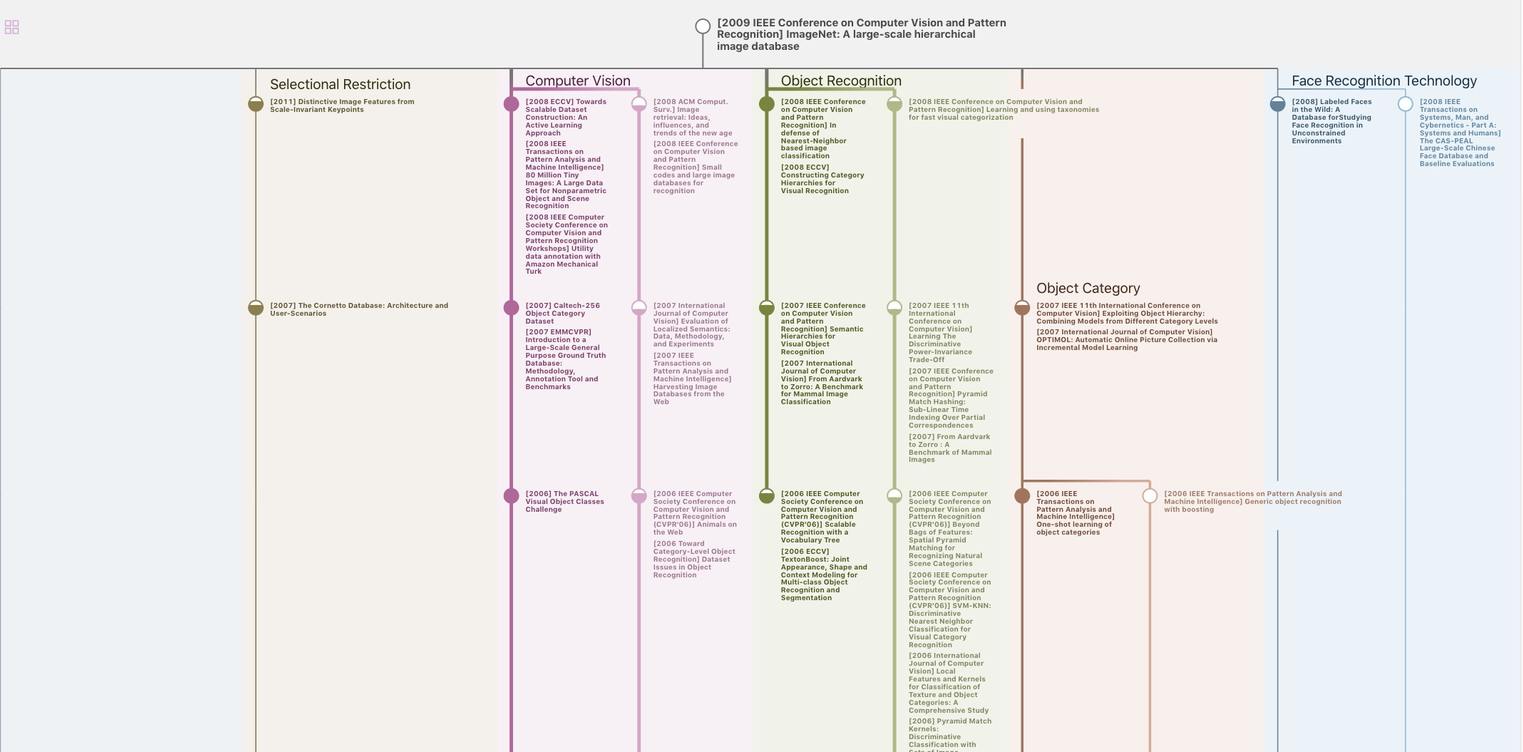
Generate MRT to find the research sequence of this paper
Chat Paper
Summary is being generated by the instructions you defined