An improved CapsNet based on data augmentation for driver vigilance estimation with forehead single-channel EEG
Cognitive Neurodynamics(2024)
摘要
Various studies have shown that it is necessary to estimate the drivers’ vigilance to reduce the occurrence of traffic accidents. Most existing EEG-based vigilance estimation studies have been performed on intra-subject and multi-channel signals, and these methods are too costly and complicated to implement in practice. Hence, aiming at the problem of cross-subject vigilance estimation of single-channel EEG signals, an estimation algorithm based on capsule network (CapsNet) is proposed. Firstly, we propose a new construction method of the input feature maps to fit the characteristics of CapsNet to improve the algorithm accuracy. Meanwhile, the self-attention mechanism is incorporated in the algorithm to focus on the key information in feature maps. Secondly, we propose substituting the traditional multi-channel signals with the single-channel signals to improve the utility of algorithm. Thirdly, since the single-channel signals carry fewer dimensions of the information compared to the multi-channel signals, we use the conditional generative adversarial network to improve the accuracy of single-channel signals by increasing the amount of data. The proposed algorithm is verified on the SEED-VIG, and Root-mean-square-error (RMSE) and Pearson Correlation Coefficient (PCC) are used as the evaluation metrics. The results show that the proposed algorithm improves the computing speed while the RMSE is reduced by 3
更多查看译文
关键词
Cross-subject,Vigilance estimation,Single-channel EEG signals,Capsule network,CGAN,Self-attention
AI 理解论文
溯源树
样例
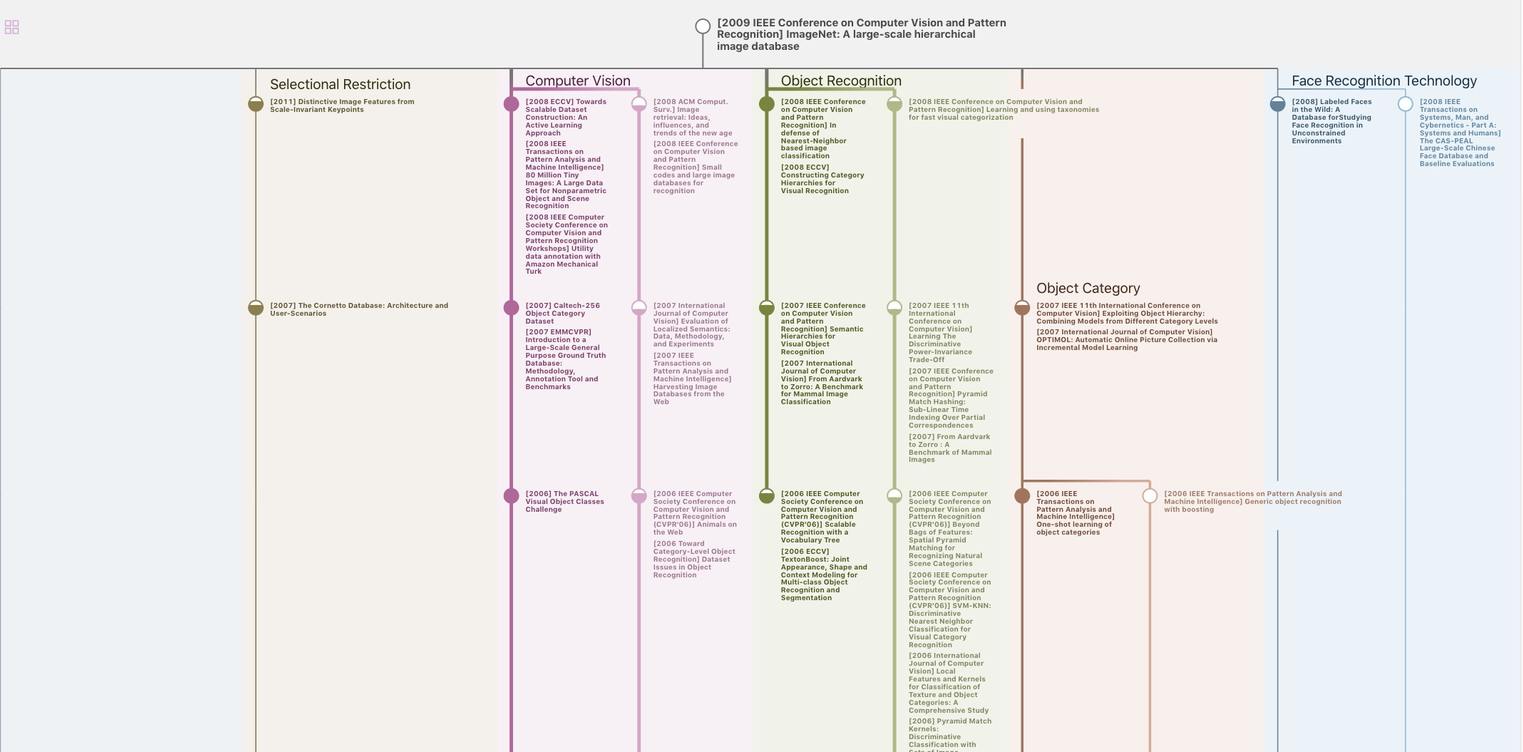
生成溯源树,研究论文发展脉络
Chat Paper
正在生成论文摘要