A machine learning-enabled prediction of damage properties for fiber-reinforced polymer composites under out-of-plane loading
Engineering Structures(2024)
摘要
For understanding the damage morphology in fiber-reinforced plastics under out-of-plane loading, engineers/ and designers mostly rely on experimental investigations and numerical models, which could be costly in terms of resources and computational requirements. Thus, pragmatic predictive approaches that can offer ready-to-use tools for material optimization have gained traction in recent years. This paper proposes a machine learning model, developed with the use of previous experiments, to predict the damage behaviour of E-glass fiber-reinforced plastics in sandwich structures and laminates. The focus is on quasi-static indentation with different indenter geometries, using acoustic signals for damage monitoring. A total of 10 optimal-performing regression algorithms were employed to develop the prediction models. The developed models based on total energy gave acceptable results for all specimens (R-squared value: 0.9932 – 0.9999), while prediction models using force produced less accurate results (R-squared value: 0.8693) for a sandwich structure subjected to a conical indenter. Results showed that energy absorption of composites provided the most reliable data for the development of predictive models. The load profiles could also be used when the contact area is limited (e.g., for hemispherical and conical indenters). The best-performing model for higher surface areas of the indenter increased was k-NN, thanks to its ability to capture complex and nonlinear relationships between the input features and the target variable. Overall, the developed model could provide more autonomy and control for the material optimisation of composite structures for industrial applications with reduced time and cost constraints.
更多查看译文
关键词
Damage prediction,Fibre-reinforced plastics,Machine learning,Quasi-static indentation
AI 理解论文
溯源树
样例
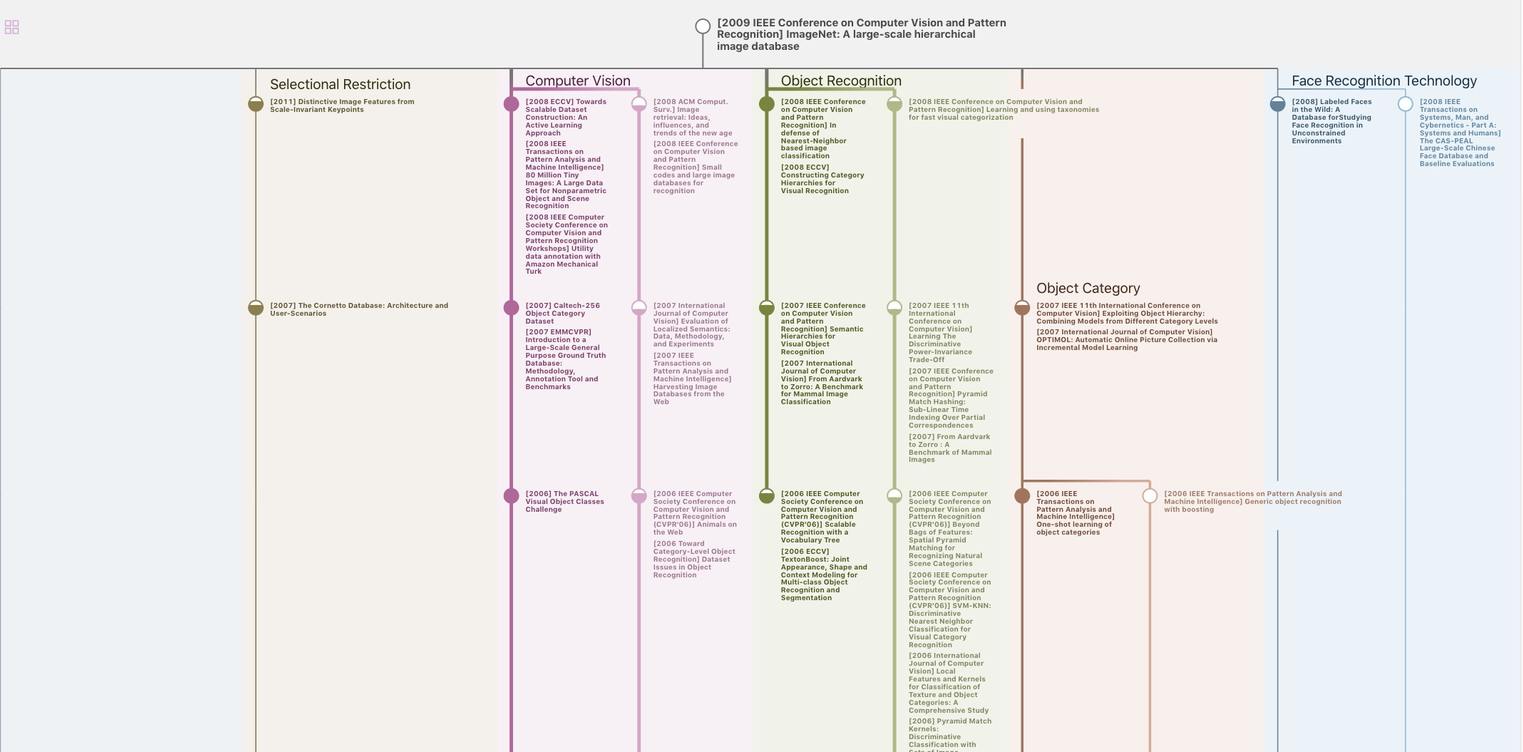
生成溯源树,研究论文发展脉络
Chat Paper
正在生成论文摘要