A Multi-Agent Deep Constrained Q-Learning Method for Smart Building Energy Management Under Uncertainties
IEEE Transactions on Smart Grid(2024)
摘要
Data-driven energy management with flexible appliances in smart buildings is a key towards power system operational intelligence. However, the low efficiency of existing deep reinforcement learning (DRL) methods in terms of optimization and computational performance, caused by reward shaping, large neural networks, system-wide constraints and reward allocation of photovoltaic power generation, signifies the need for new system-specific DRL methods. To address these challenges, this paper proposes a multi-agent deep constrained Q-learning method to obtain online optimal solutions for smart building energy management in presence of various uncertainties. The proposed method minimizes daily energy cost via real-time adjustment of flexible appliances, and addressing impacts of the uncertainties. A deep constrained Q-learning algorithm is developed to effectively avoid reward shaping. By adopting multi-layer perception to estimate thermodynamics and electric vehicle charging states, and developing appliance-specific logic, it is novel to calculate the joint safe action space of all appliances during the training process. A multi-agent approach is developed to address the system-wide constraints and the reward allocation, directly in the Q-update, where hyper-parameters of individual agents are tuned separately. Numerical simulation results verify the high efficiency of the proposed method in daily energy cost minimization and online energy management.
更多查看译文
关键词
Data-driven optimization,deep reinforcement learning,constrained Q-learning,building energy management system,uncertainty
AI 理解论文
溯源树
样例
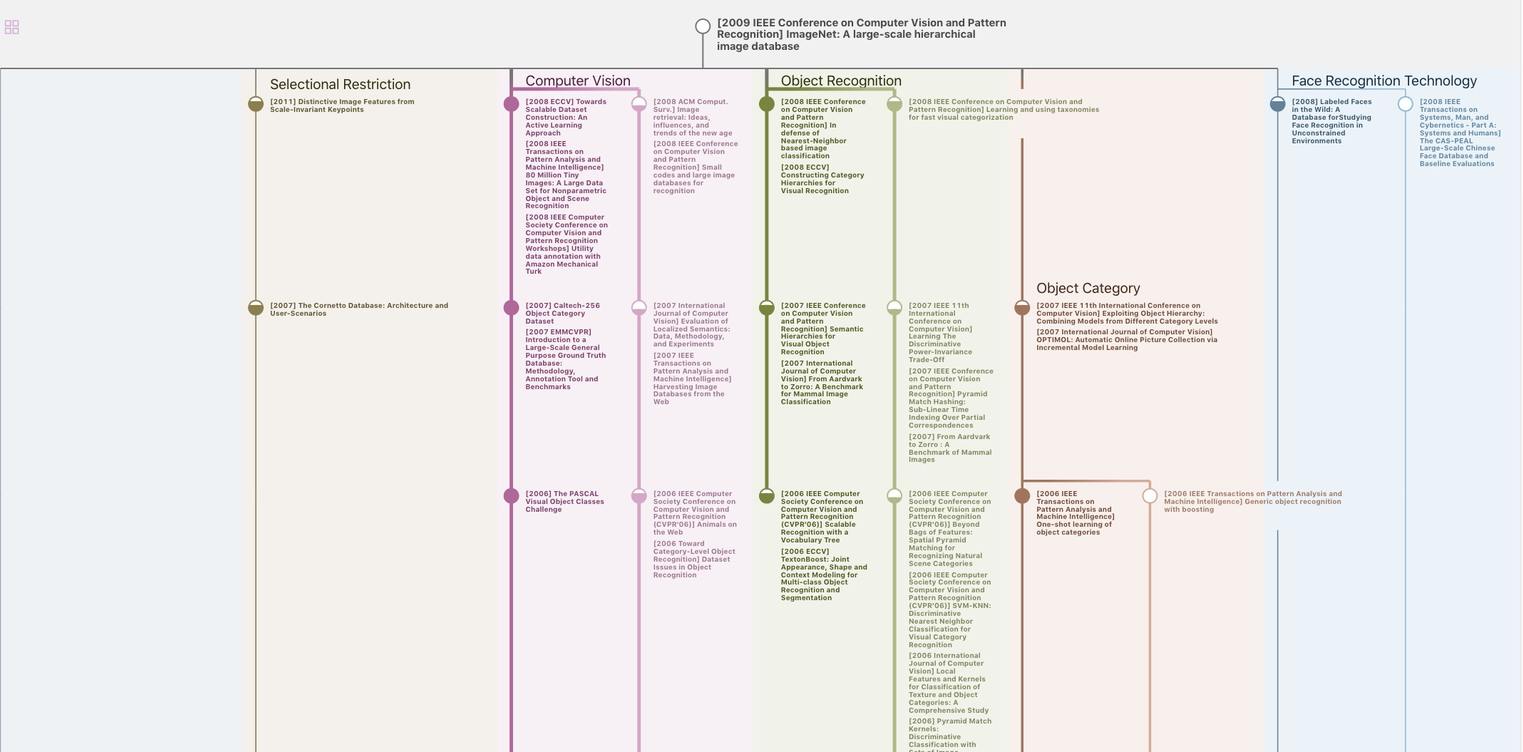
生成溯源树,研究论文发展脉络
Chat Paper
正在生成论文摘要